A novel fault detection framework integrated with variable importance analysis for quality-related nonlinear process monitoring
Control Engineering Practice(2023)
摘要
Monitoring the quality-related variables is an essential task in industrial processes. However, large amounts of variables and complex relationship between measured and quality variables pose challenges in improving fault detection performance. This paper proposes a novel quality-related fault detection framework based on variable importance analysis for nonlinear process monitoring. In the proposed framework, a new nonlinear variable division index called improved variable importance in the projection (IVIP) is designed to better identify quality-related variables. First, measured variables are divided into quality-related and independent spaces according to their IVIP values. Then, considering different data characteristics, a hybrid detection model combined multivariate exponentially weighted moving average and kernel principal component analysis (MEKPCA) is constructed in these two spaces to monitor abnormal events. To provide an integrated decision, the Bayesian inference strategy is finally utilized to fuse useful information from different spaces. Three case studies, including a simulated numerical system, the Tennessee Eastman process, and a wastewater treatment process, demonstrate that our proposed IVIP-MEKPCA outperforms other comparison methods.
更多查看译文
关键词
Fault detection, Nonlinear processes, Quality-related process monitoring, Variable division
AI 理解论文
溯源树
样例
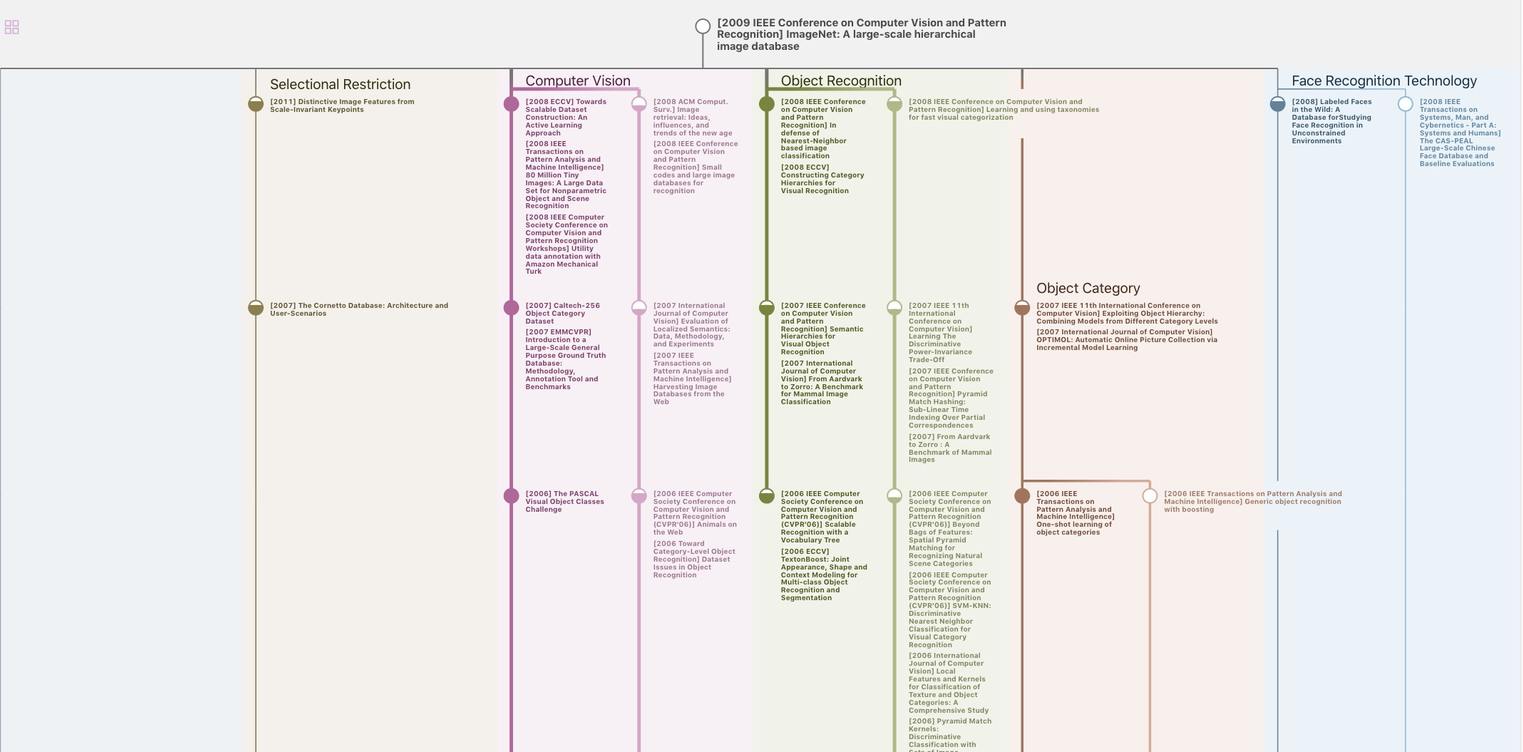
生成溯源树,研究论文发展脉络
Chat Paper
正在生成论文摘要