Memory-Driven Region Contrast for Enhanced Polyp Semantic Segmentation
2023 International Conference on Multimedia Analysis and Pattern Recognition (MAPR)(2023)
摘要
Colon polyp recognition is challenging for the automatic analysis of endoscopic images in computer-aided clinical support systems. While recent deep learning models have shown impressive results in polyp segmentation, they often reveal weaknesses when dealing with scarce and imbalanced training data. To overcome this issue, we present a novel effective method for polyp semantic segmentation using contrastive learning supported by a memory bank. Contrastive learning disentangles the feature representation of different object classes. Meanwhile, the memory bank gathers hard pixel samples within successive mini-batches to deal with the scarcity of polyp class that may occur in a single mini-batch. Furthermore, we leverage the teacher-student training to force the model to learn consistent feature representation, resulting in final polyp segmentation improvement. Experiments show that our method yields superior performance by a large margin compared to other state-of-the-art approaches. Our code is available at: https://github.com/hoainv99/meco-polyp.
更多查看译文
关键词
Semantic Segmentation,Contrastive Learning,Deep Learning,Colonoscopy
AI 理解论文
溯源树
样例
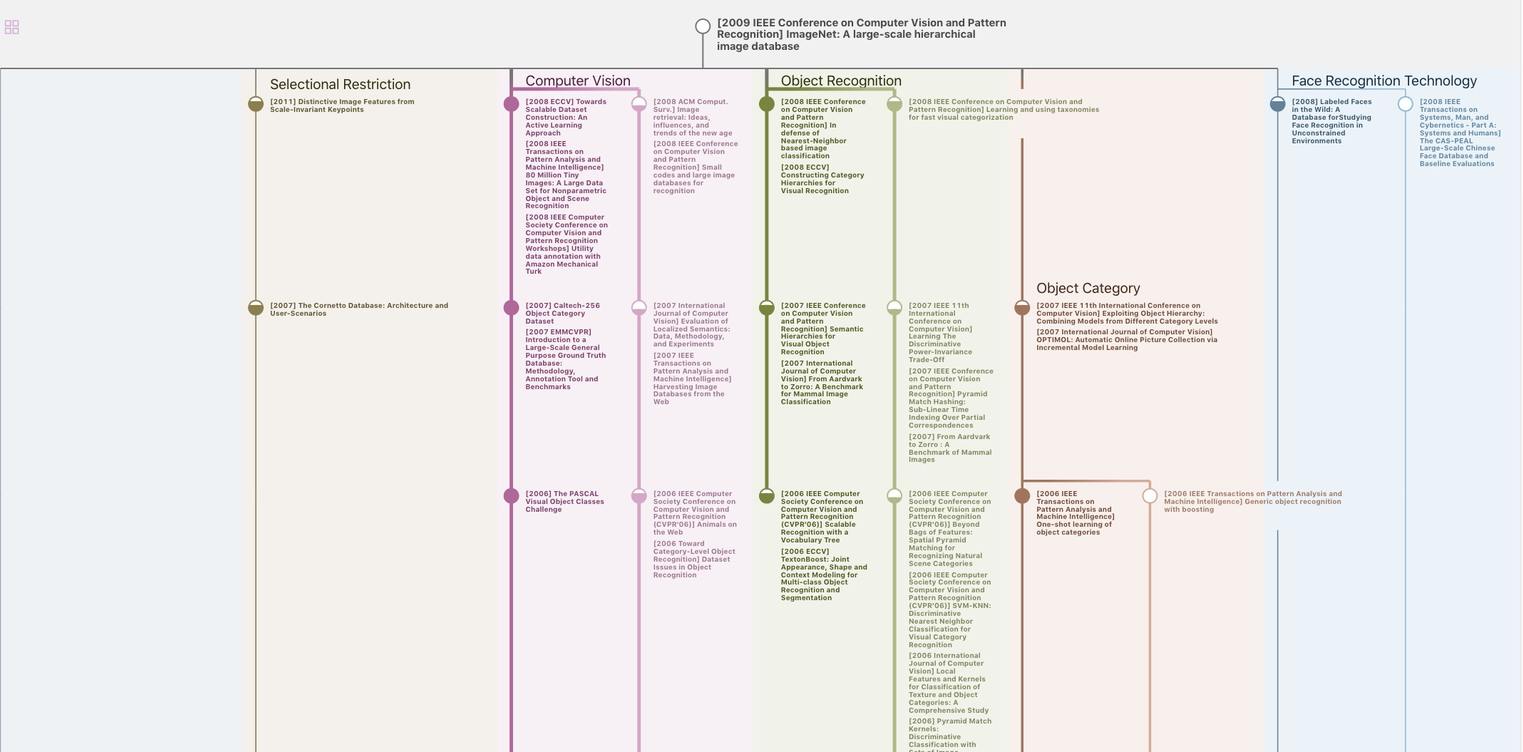
生成溯源树,研究论文发展脉络
Chat Paper
正在生成论文摘要