Scene Parsing Using Fully Convolutional Network for Semantic Segmentation
2023 IEEE Canadian Conference on Electrical and Computer Engineering (CCECE)(2023)
摘要
When it comes to computer vision, scene parsing is a crucial part of semantic segmentation. It has a wide range of applications, including autonomous driving, robotics, gaming, natural language processing, object detection, and image and video editing. Semantic segmentation works by classifying each pixel of an image according to the object it belongs to, and scene parsing provides contextual information to improve the accuracy and robustness of deep learning models used for this purpose. In this study, we used the Fully Convolutional Network (FCN-8) architecture, a popular deep learning-based technique that achieves higher accuracy than traditional and state-of-the-art methods. This is achieved by creating hierarchies of distinctive features in an image. The FCN-8 is used to perform semantic segmentation efficiently, taking an image of any size as input and producing correspondingly sized output with effective inference and learning. To fine-tune the FCN-8 for the MIT Scene Parsing Challenge Dataset, we employed a transfer learning approach. Our results showed that our proposed approach achieved an accuracy of 72% on the dataset. This is significant given the relatively small number of samples and the 150 classes of objects. Our work demonstrates a successful pilot study for deploying transfer learning and the FCN-8 architecture for scene parsing and semantic segmentation.
更多查看译文
关键词
Semantic Segmentation,Fully Convolutional Network (FCN),Scene Parsing
AI 理解论文
溯源树
样例
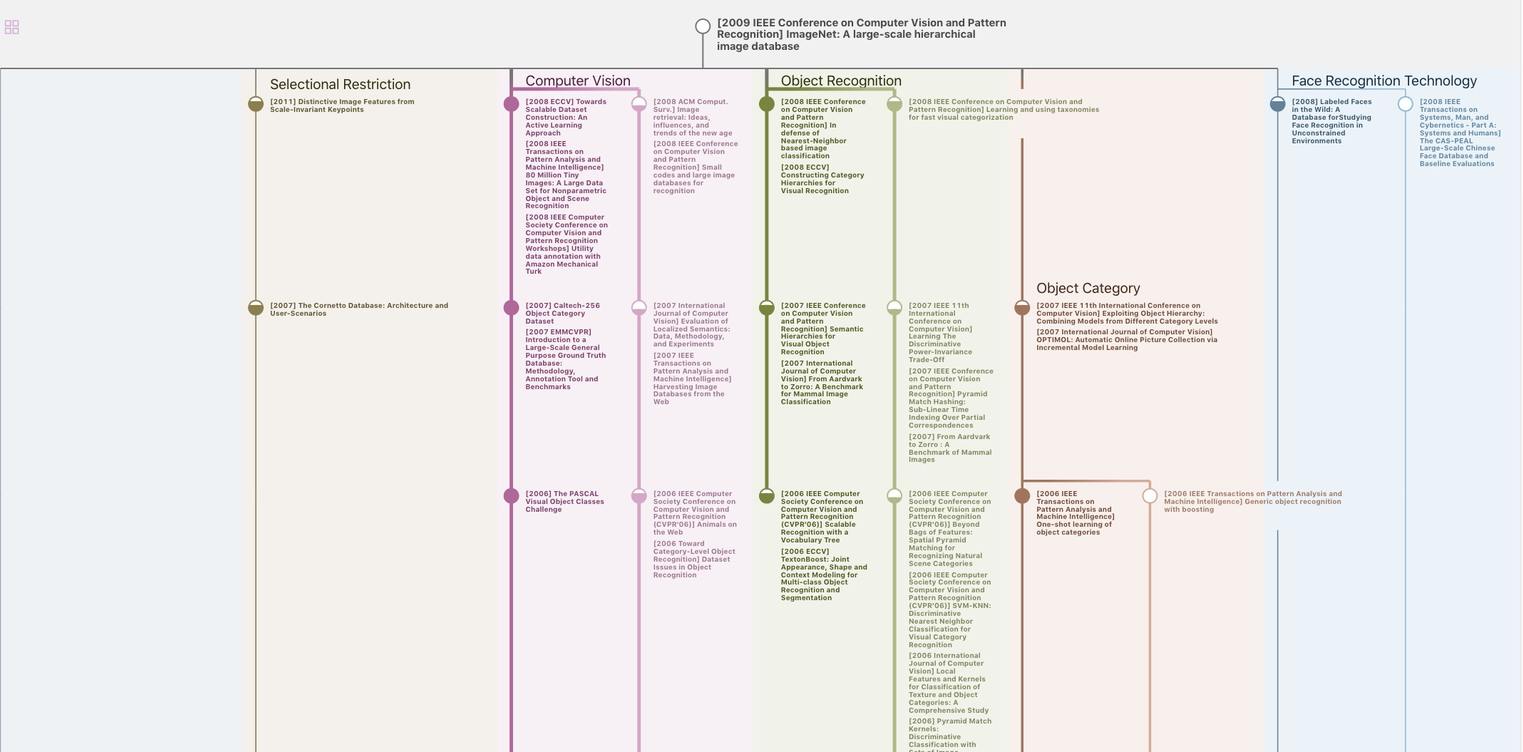
生成溯源树,研究论文发展脉络
Chat Paper
正在生成论文摘要