Multi-Objective Considered Process Parameter Optimization of Welding Robots Based on Small Sample Size Dataset
Sustainability(2023)
摘要
The welding process is characterized by its high energy density, making it imperative to optimize the energy consumption of welding robots without compromising the quality and efficiency of the welding process for their sustainable development. The above evaluation objectives in a particular welding situation are mostly influenced by the welding process parameters. Although numerical analysis and simulation methods have demonstrated their viability in optimizing process parameters, there are still limitations in terms of modeling accuracy and efficiency. This paper presented a framework for optimizing process parameters of welding robots in industry settings, where data augmentation was applied to expand sample size, auto machine learning theory was incorporated to quantify reflections from process parameters to evaluation objectives, and the enhanced non-dominated sorting algorithm was employed to identify an optimal solution by balancing these objectives. Additionally, an experiment using Q235 as welding plates was designed and conducted on a welding platform, and the findings indicated that the prediction accuracy on different objectives obtained by the enlarged dataset through ensembled models all exceeded 95%. It is proven that the proposed methods enabled the efficient and optimal determination of parameter instructions for welding scenarios and exhibited superior performance compared with other optimization methods in terms of model correctness, modeling efficiency, and method applicability.
更多查看译文
关键词
welding robots,process parameter optimization,multiple objectives,small sample size dataset,auto machine learning
AI 理解论文
溯源树
样例
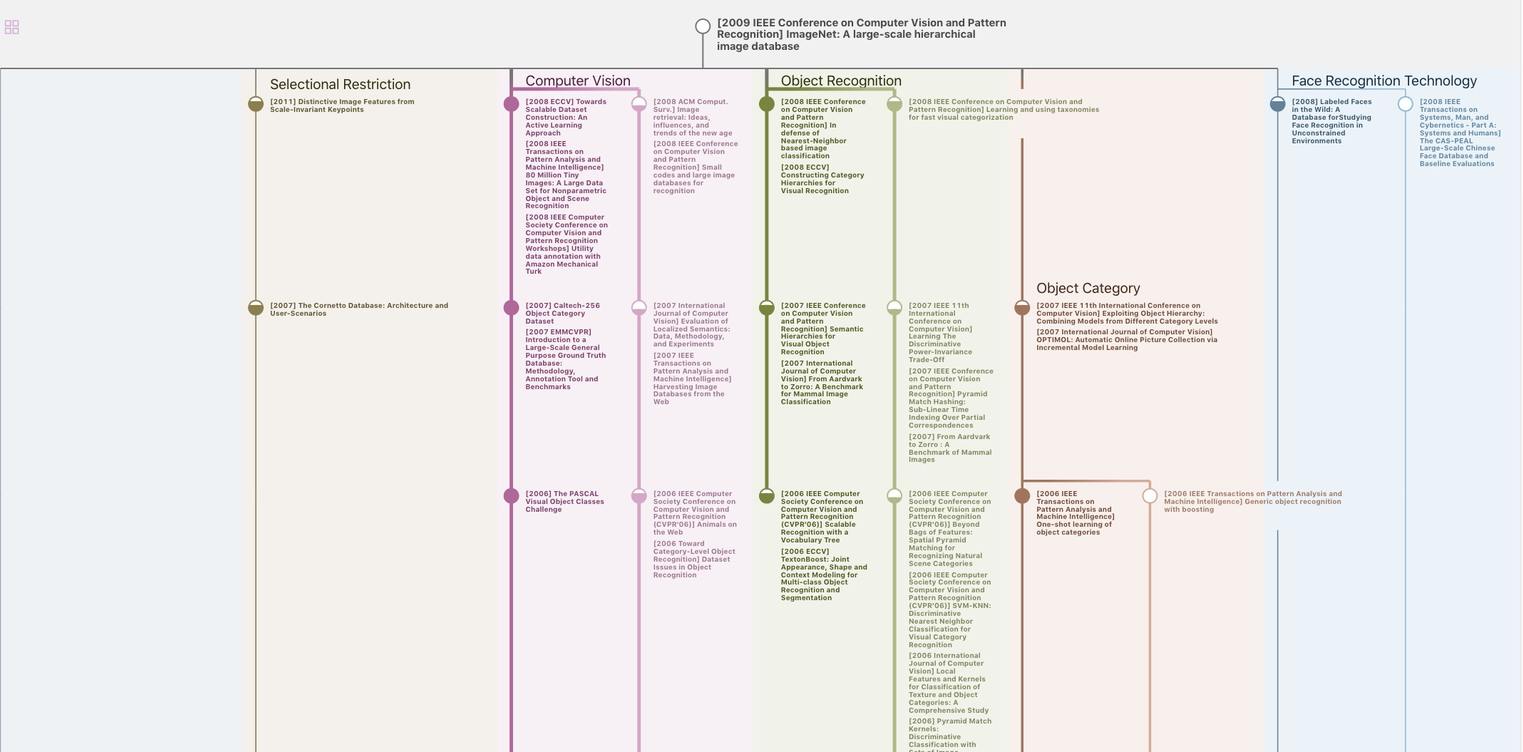
生成溯源树,研究论文发展脉络
Chat Paper
正在生成论文摘要