Intelligent and Small Samples Gear Fault Detection Based on Wavelet Analysis and Improved CNN
Processes(2023)
摘要
Traditional methods for identifying gear faults typically require a substantial number of faulty samples, which in reality are challenging to obtain. To tackle this challenge, this paper introduces a sophisticated approach for intelligent gear fault identification, utilizing discrete wavelet decomposition and an enhanced convolutional neural network (CNN) optimized for scenarios with limited sample data. Initially, the features of the sample signal are extracted and enhanced using discrete wavelet decomposition. Subsequently, the refined signal is transformed into a two-dimensional image through a Markov transition field, preparing it for improved two-dimensional CNN training. Finally, the refined network model is applied to assess the gear fault dataset, achieving a training accuracy of 97% and a classification accuracy of 88.33%. This demonstrates the method's feasibility and effectiveness in identifying gear faults with limited sample data.
更多查看译文
关键词
fault detection, improved neural network, wavelet analysis, small samples
AI 理解论文
溯源树
样例
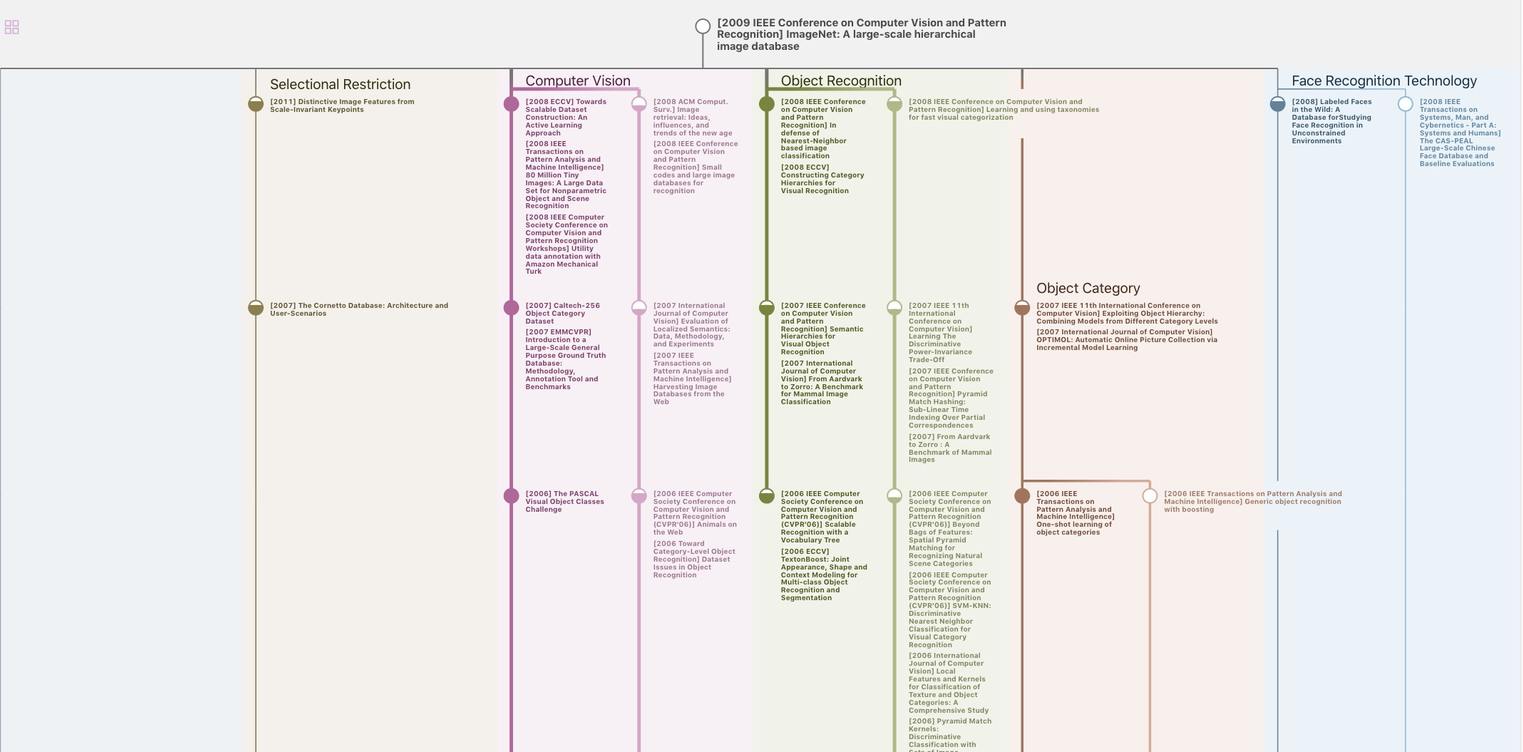
生成溯源树,研究论文发展脉络
Chat Paper
正在生成论文摘要