A Two-Stage Hypervolume-Based Evolutionary Algorithm for Many-Objective Optimization
Mathematics(2023)
摘要
Many-objective optimization is a critical research topic in the evolutionary computing community. Many algorithms have been proposed to tackle this problem, with evolutionary algorithms based on the hypervolume being among the most effective ones. However, calculating the hypervolume indicator in high-dimensional objective spaces remains time-consuming. To address this issue, we propose a two-stage hypervolume-based evolutionary algorithm (ToSHV) that separates global search and local search to ensure both convergence and diversity. ToSHV performs a global search in the first stage by generating multiple offspring per generation. We modified the R2HCA method to estimate the overall hypervolume contribution, avoiding the time-consuming nature of updating the hypervolume contribution with the greedy method. In the second stage, only one offspring is produced per generation to emphasize local exploration and enhance population diversity. Furthermore, a stage-switching mechanism is designed to dynamically select the appropriate search mode based on the prevailing population distribution. We evaluate our algorithm on WFG and DTLZ test suites, comparing it with three hypervolume-based algorithms and four state-of-the-art algorithms. Experimental results show that our approach is competitive in most cases.
更多查看译文
关键词
evolutionary algorithm,optimization,two-stage,hypervolume-based,many-objective
AI 理解论文
溯源树
样例
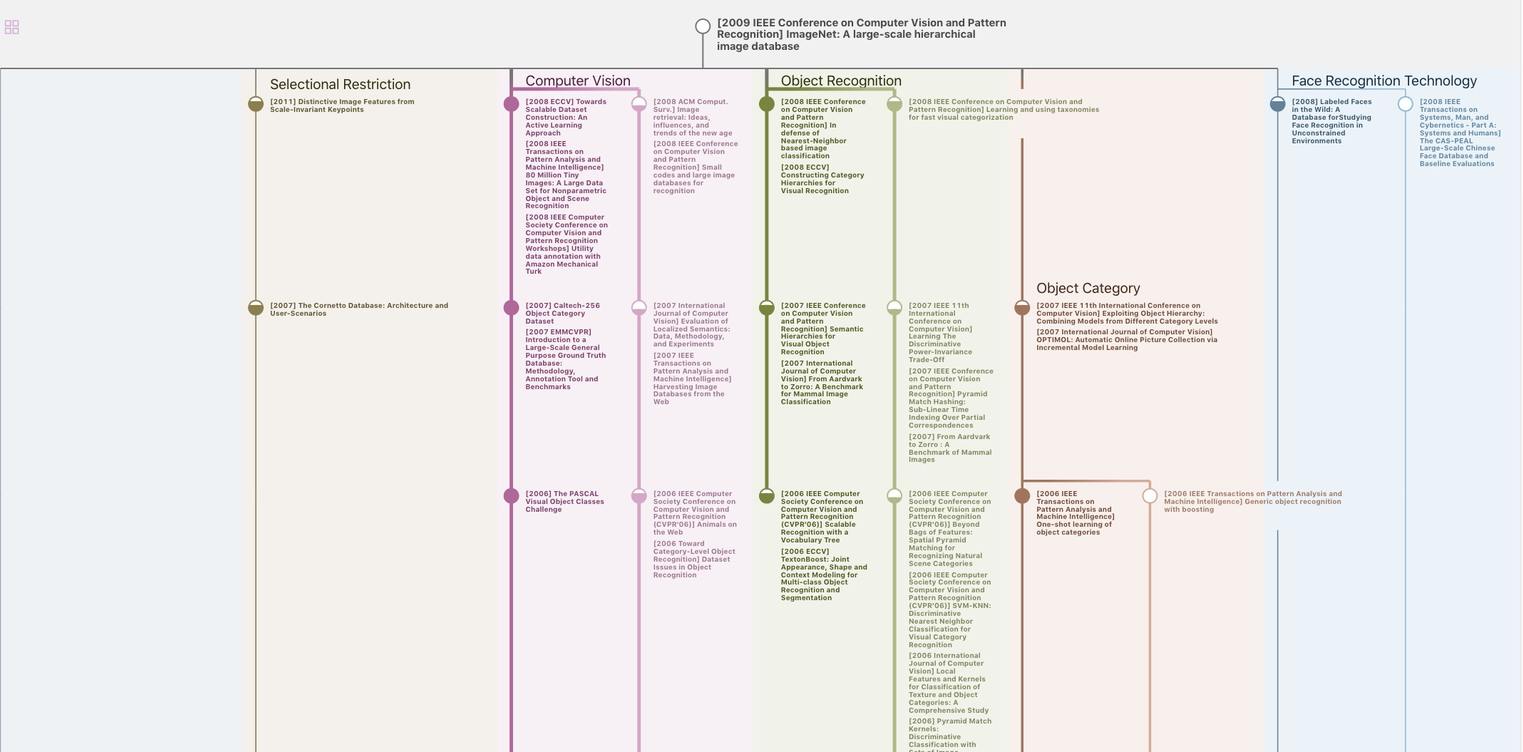
生成溯源树,研究论文发展脉络
Chat Paper
正在生成论文摘要