A Sparse Bayesian Learning Method for Direction of Arrival Estimation in Underwater Maneuvering Platform Noise
Journal of Marine Science and Engineering(2023)
摘要
The underwater maneuvering platform generates self-noise when sailing, which shows spatial directionality to the arrays fixed on the platform. In this paper, it is called spatially colored noise (SCN). The direction of arrival (DOA) estimation results are often influenced by this self-noise, leading to a decrease in estimation accuracy and to the appearance of spurious peaks. To resolve this problem, a sparse Bayesian learning (SBL) method adapted to underwater maneuvering platform noise is proposed in this paper. The SBL framework with unknown SCN is established first. Then, the SCN covariance matrix is estimated by projecting the received data covariance matrix into the noise subspace, and the DOA estimation results are finally obtained through multiple iterations. The simulation results show that the proposed method avoids spurious peaks, and compared to the existing methods, the proposed method achieves a higher accuracy in the case of low SNRs and small snapshot numbers. The sea trial data processing results show that the proposed method provides lower and flatter noise spectrum levels without spurious peaks.
更多查看译文
关键词
DOA estimation,sparse Bayesian learning,underwater maneuvering platform noise,spatially colored noise
AI 理解论文
溯源树
样例
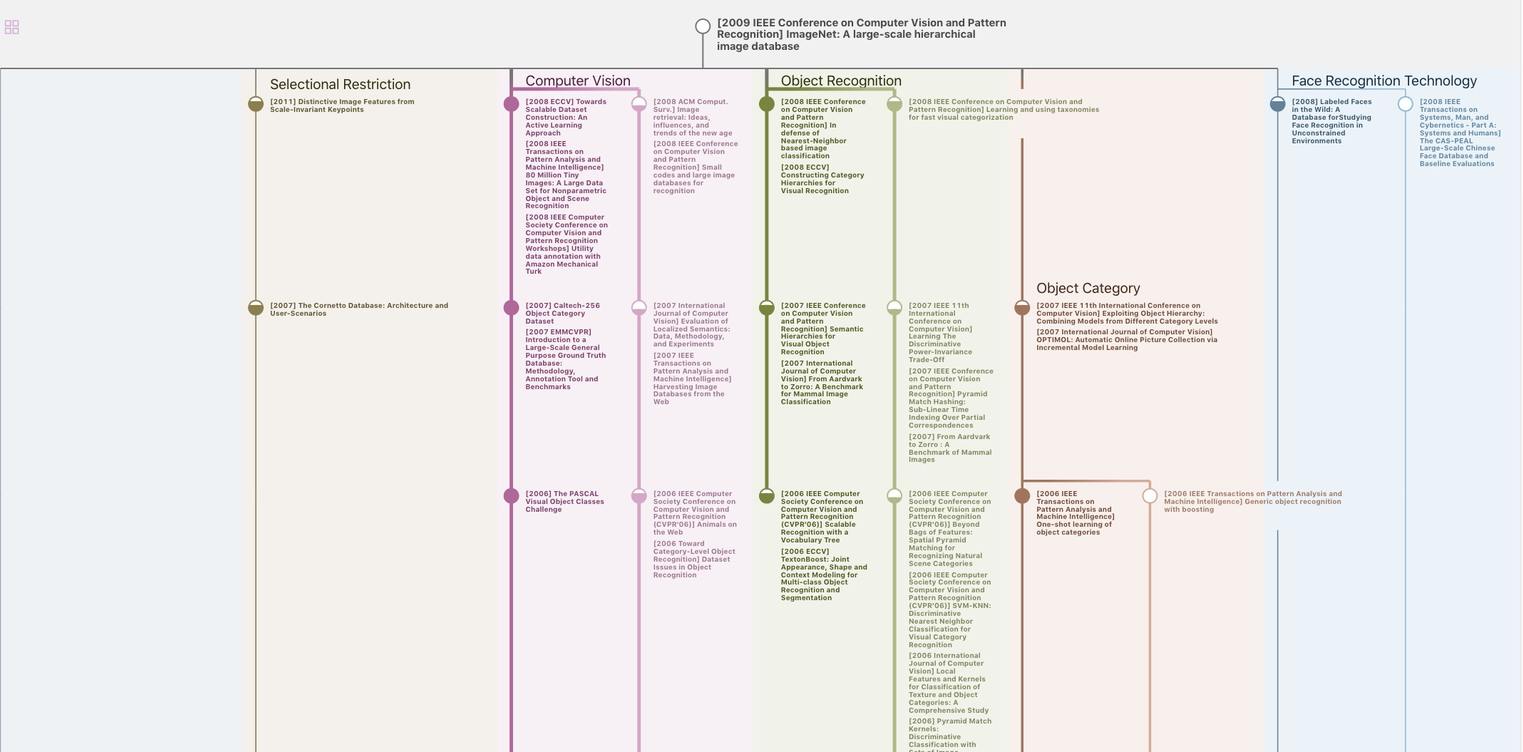
生成溯源树,研究论文发展脉络
Chat Paper
正在生成论文摘要