Deep reinforcement learning-based resilience enhancement strategy of unmanned weapon system-of-systems under inevitable interferences
RELIABILITY ENGINEERING & SYSTEM SAFETY(2024)
摘要
Unmanned weapon system-of-systems (UWSoS) is a collection of unmanned weapon systems providing multiple interdependent capabilities to support the mission completion. The soaring number of interconnected systems makes UWSoS vulnerable in the face of inevitable uncertain interferences. Thus, devising an effective resilience enhancement strategy is critical to handling inevitable disruption events. However, preventive and protective methods are not all-inclusive because of the high uncertainty operation environment and the lack of autonomy. Hence, we studied the resilience enhancement problem of UWSoS from the recovery perspective based on deep reinforcement learning (DRL). First, a DRL-based resilience enhancement strategy framework is proposed, combining the graph convolution network and proximal policy optimization algorithm to extract the entities' representation features and autonomously learn the resilience enhancement strategy to handle various interferences scenarios better. Subsequently, a collaboration action resilience contribution index-guided proximal policy optimization algorithm is proposed to improve training efficiency. Finally, extensive simulation experiments and comparisons with five similar algorithms demonstrate the effectiveness, adaptability, and superiority of the proposed strategy. This work could provide valuable scheduling schemes for decision-makers to guide the reliable operation of UWSoSs.
更多查看译文
关键词
Resilience enhancement strategy,Unmanned weapon system-of-systems (UWSoS),Deep reinforcement learning (DRL),Graph convolution network (GCN),Proximal policy optimization (PPO)
AI 理解论文
溯源树
样例
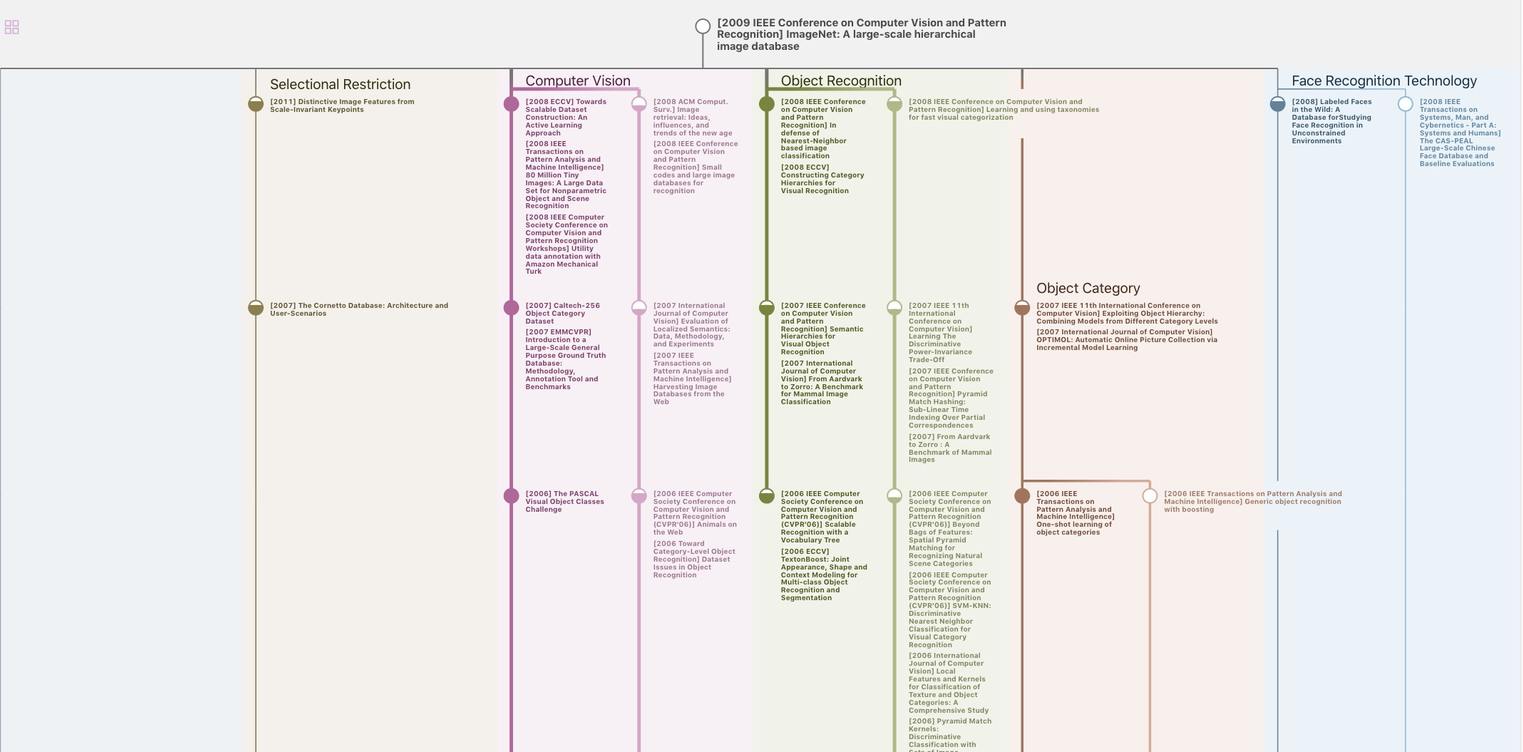
生成溯源树,研究论文发展脉络
Chat Paper
正在生成论文摘要