A Jackknife-Inspired Deep Learning Approach to Subject-Independent Classification of EEG
Pattern Recognition Letters(2023)
摘要
One of the significant challenges in Brain-Computer Interface (BCI) is to develop a classifier that can decode users' mental states based on electroencephalogram (EEG) data collected from independent subjects. The focus of such subject-independent (SI) classification is justified because it can lead to BCIs that do not require a user-specific calibration process. In recent years, the emergence of deep neural networks (DNNs) has significantly improved the performance of EEG classification. Among various deep learning techniques, the training efficiency and the performance of Convolutional Neural Networks (CNNs), in particular, have led to several state-of-the-art architectures for accurate classification of EEG. Not surprisingly, the efforts to improve the performance of these architectures for EEG classification have been ramped up in recent years. In this regard, a trivial approach is to train and tune a large number of architectures and hyperparameters and hope to improve upon the existing results. In contrast with this ad hoc approach, here we put forward a systematic method inspired by the jackknife estimation to improve the performance of existing CNN architectures. Using EEGNet and ShallowConvNet as archetypical, our empirical results show that the proposed "Delete-a-Subject Jackknife"(DASJ) technique can potentially improve the performance of existing CNN architectures for SI classification of EEG.
更多查看译文
关键词
Convolutional neural network,Delete-a-Subject-Jackknife,Deep learning,Subject-independent
AI 理解论文
溯源树
样例
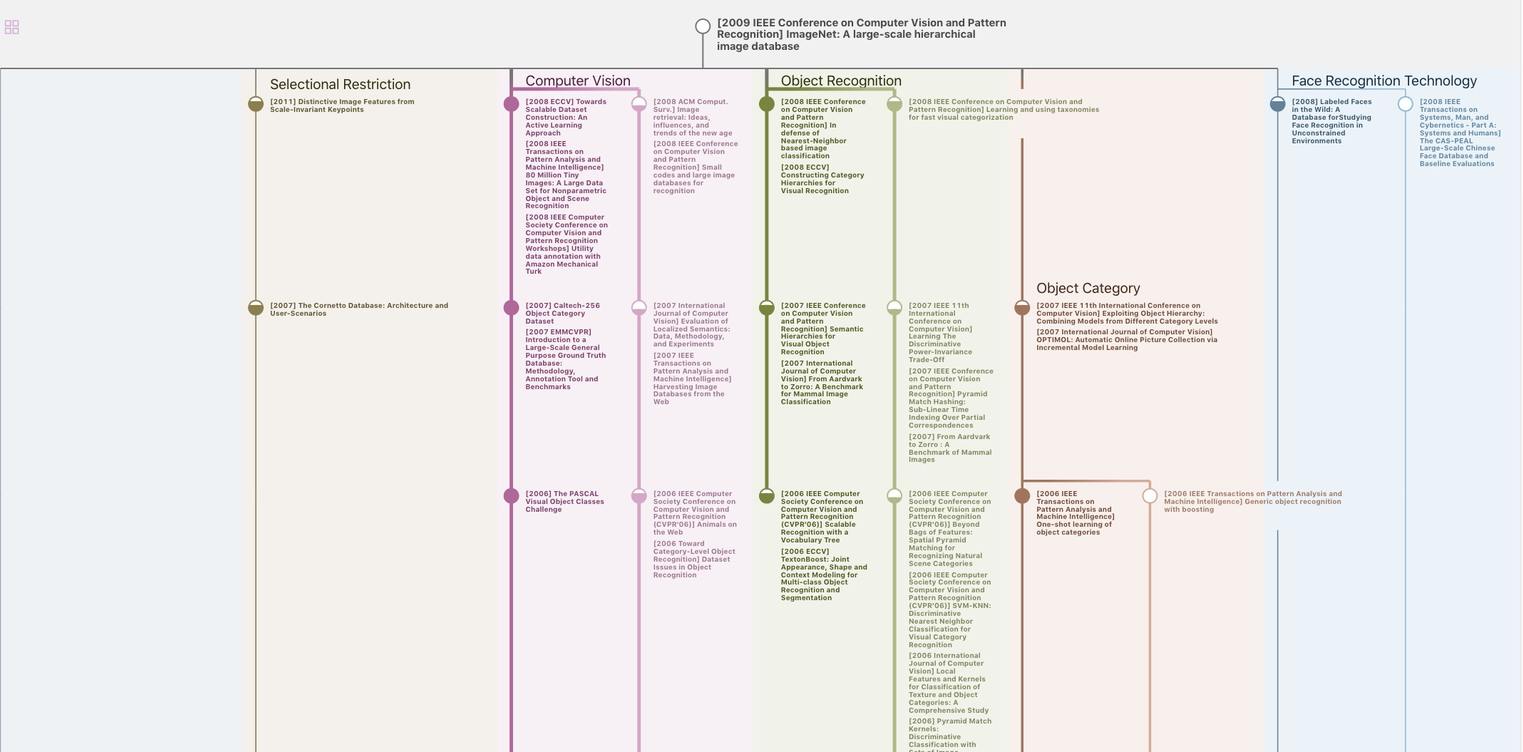
生成溯源树,研究论文发展脉络
Chat Paper
正在生成论文摘要