Gradient Complexity and Non-stationary Views of Differentially Private Empirical Risk Minimization
Theoretical computer science(2024)
摘要
In this paper, we study the Differentially Private Empirical Risk Minimization (DP-ERM) problem, considering both convex and non-convex loss functions. For cases where DP-ERM involves smooth (strongly) convex loss functions with or without non-smooth regularization, we propose several novel methods. These methods achieve (near) optimal expected excess risks (i.e., utility bounds) while reducing the gradient complexity compared to existing approaches. When dealing with DP-ERM and smooth convex loss functions in high dimensions, we introduce an algorithm that achieves a superior upper bound with lower gradient complexity than previous solutions. In the second part of the paper, for DP-ERM with non-convex loss functions, we explore both low and high dimensional spaces. In the low dimensional case with a non-smooth regularizer, we extend an existing approach by measuring the utility using the ℓ 2 norm of the projected gradient. Furthermore, we introduce a novel error bound measurement, transitioning from empirical risk to population risk by employing the expected ℓ 2 norm of the gradient. For the high dimensional case, we demonstrate that by measuring utility with the Frank-Wolfe gap, we can bound the utility using the Gaussian Width of the constraint set, instead of the dimensionality ( p ) of the underlying space. We also show that the advantages of this approach can be achieved by measuring the ℓ 2 norm of the projected gradient. Finally, we reveal that the utility of certain special non-convex loss functions can be reduced to a level (depending only on log p) similar to that of convex loss functions.
更多查看译文
关键词
Differential privacy,Empirical risk minimization,Convex optimization,Non-convex optimization,Supervised learning
AI 理解论文
溯源树
样例
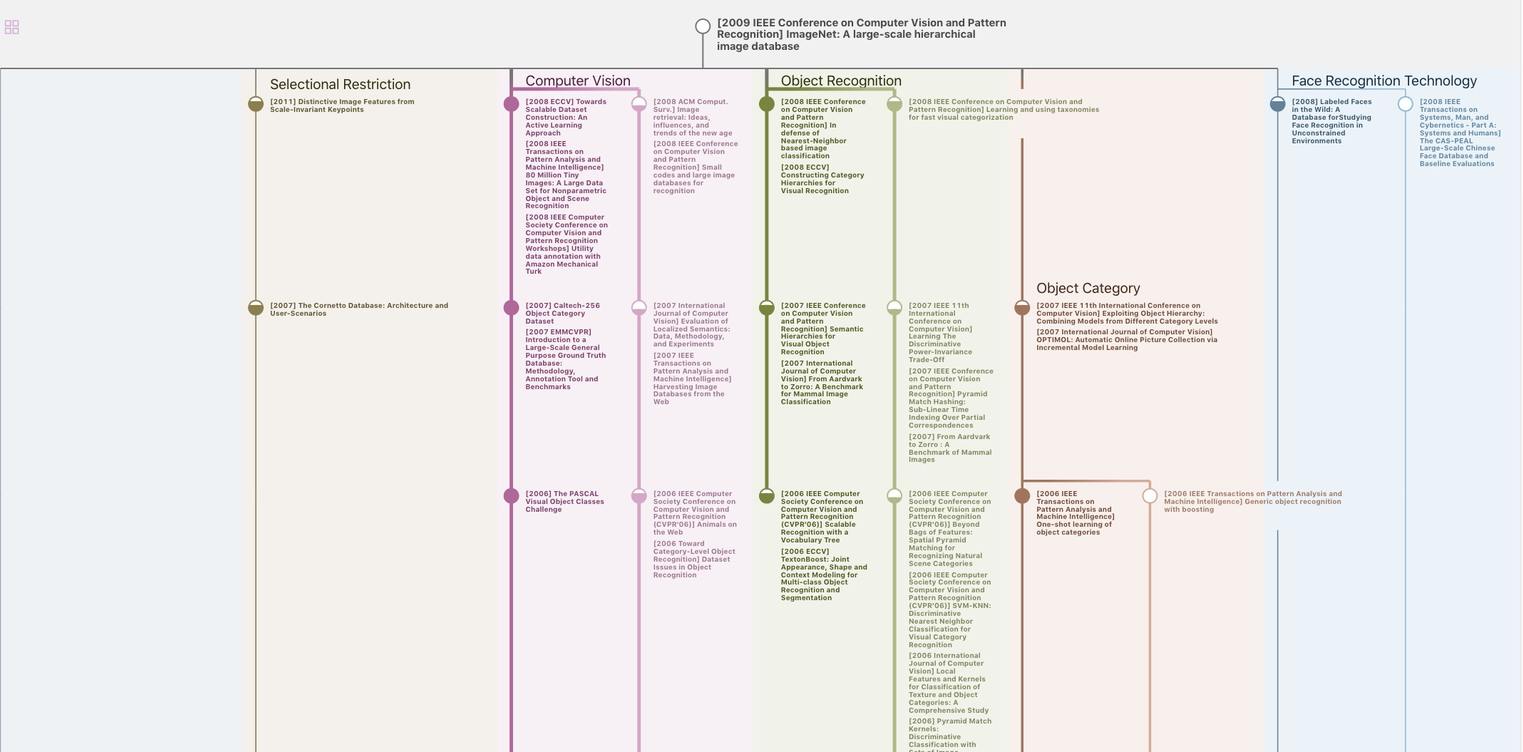
生成溯源树,研究论文发展脉络
Chat Paper
正在生成论文摘要