Attention-guided graph convolutional network for multi-behavior recommendation
Knowledge-Based Systems(2023)
摘要
Multi-behavior recommendation system aims to improve recommendation performance by using the interaction data of users ' multiple behaviors. Although some methods have explored the dependencies between different behaviors, there are also existing challenges: (1) user-item interactions have complex dependencies; (2) the dependencies between multiple behaviors vary due to users ' personalized preferences. To address these challenges, we propose a new model MB-AGCN (Attention-Guided Graph Convolutional Network for Multi -Behavior Recommendation), which considers personalized interaction patterns and cross-typed behavioral interdependencies. In the MB-AGCN framework, we take the different effects of multi-behavior information on predicting user preferences into account. We first model the user multi-behavior relationships with the attention mechanism to capture the personalized multi-behavior characteristics. Then, we explore the knowledge learned from the multi-behavior relationship modeling to generate a weight matrix that guides the graph neural network to learn the complex dependencies in different types of user-item interactions and capture the relationships between different types of behaviors. A comprehensive evaluation on three real-world datasets shows that MB-AGCN consistently outperforms state-of-the-art methods. Our codes will be available at https://github.com/3endurance/MB-AGCN.
更多查看译文
关键词
Recommendation system,Graph convolutional network,Attention mechanism,Multi-behavior
AI 理解论文
溯源树
样例
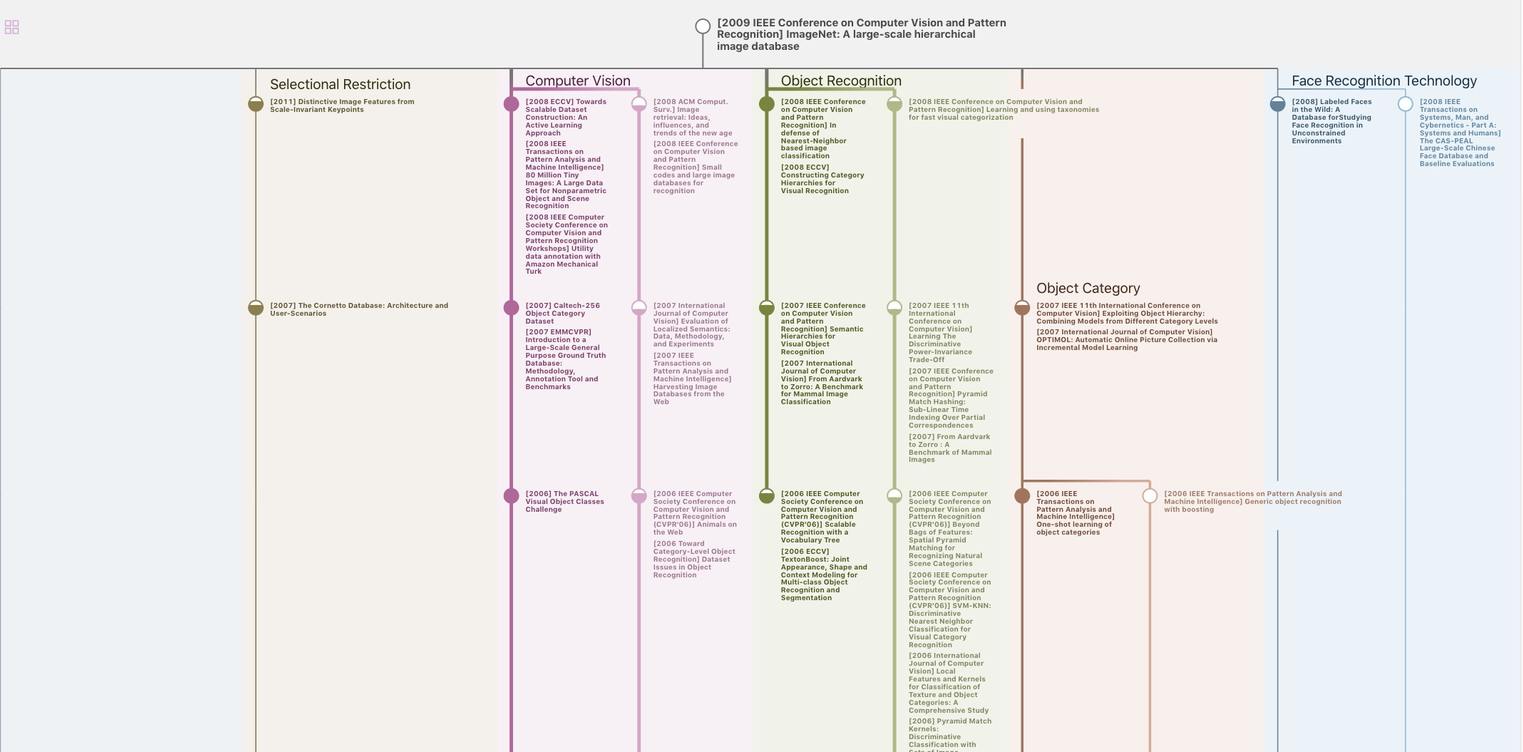
生成溯源树,研究论文发展脉络
Chat Paper
正在生成论文摘要