TCRec: A Novel Paper Recommendation Method Based on Ternary Coauthor Interaction
Knowledge-based systems(2023)
摘要
With the explosion in the number of papers being generated, it has become a common practice to recommend papers related to research interests of authors. Existing methods mainly focus on using binary author–paper interactions to mine the research interests of authors. However, sparse binary interactions make it extremely difficult to distinguish the authors’ research interests. This results in an inability to find papers that authors need. Generally, the research interests of coauthors are highly correlated and this is useful in broadening the distinctions between authors’ research interests. Therefore, we propose a new ternary coauthor recommendation (TCRec) method for paper recommendation. Specifically, we construct a coauthor ternary to describe the coauthorship between authors to explore potential ternary interactions. First, an attention-based bidirectional long short-term memory (Bi-LSTM) is built to learn title and abstract information to represent the research area of a paper and accordingly initialize the research interests for each author. Then, an academic heterogeneous graph with a dual attention mechanism is designed to aggregate the semantic information of neighboring nodes and metapaths. This mechanism can be employed to aggregate the relationships between authors and papers in the academic network to describe the research interests for authors. Finally, after constructing the ternary, a multilayer perceptron (MLP) is applied to mine potential associations between the ternary to enrich author–paper interactions for paper recommendation. Extensive experimental results from two real academic datasets not only show the superior performance of our model over state-of-the-art approaches but also demonstrate its potential value for paper recommendation.
更多查看译文
关键词
Paper recommendation,Coauthorship,Heterogeneous graph,Attention
AI 理解论文
溯源树
样例
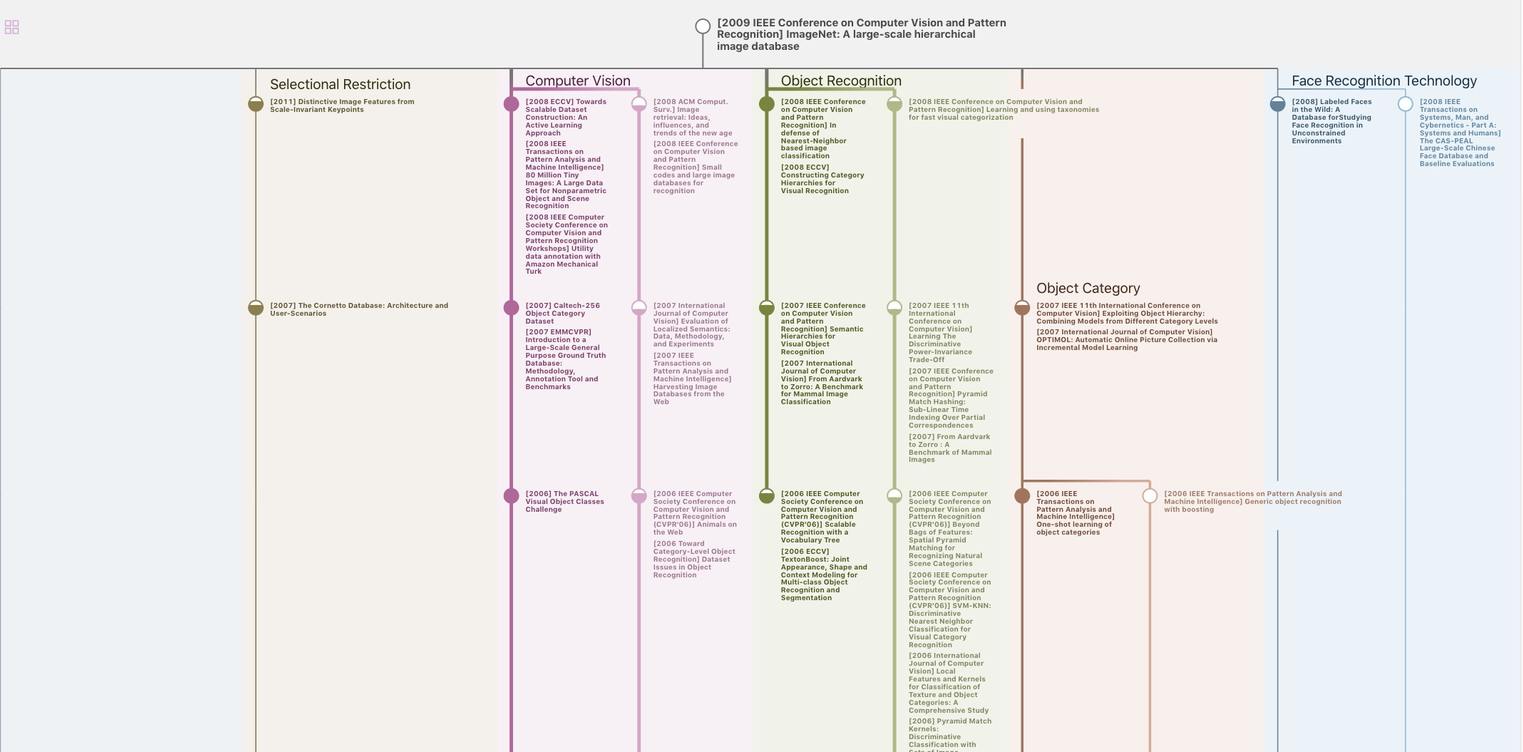
生成溯源树,研究论文发展脉络
Chat Paper
正在生成论文摘要