VTwins: inferring causative microbial features from metagenomic data of limited samples
Science Bulletin(2023)
摘要
It is difficult to infer causality from high-dimension metagenomic data due to interference from numer-ous confounders. By imitating the twin studies in genetic research, we develop a straightforward method-virtual twins (VTwins)-to eliminate the confounder effects by transforming the original cohort into a paired cohort of "Twin" samples with distinct phenotypes but matched taxonomic profiles. The results show that VTwins outperforms the conventional approach in the sensitivity of identifying causa-tive features and only requires a 10-fold reduced sample size for recalling disease-associated microbes or pathways, as tested by simulated and empirical data. Benchmark test with other 16 kinds of software fur-ther validates the power and applicability of VTwins for handling high-dimension compositional datasets and mining causalities in metagenomic research. In conclusion, VTwins is straightforward and effective in handling high-diversity, high-dimension compositional data, promising applications in mining causali-ties for metagenomic and potentially other omics data. VTwins is open access and available at https:// github.com/mengqingren/VTwins.(c) 2023 Science China Press. Published by Elsevier B.V. and Science China Press. This is an open access article under the CC BY license (http://creativecommons.org/licenses/by/4.0/).
更多查看译文
关键词
Causality,High-dimensional data,Metagenome,Differential abundance,Paired cohort
AI 理解论文
溯源树
样例
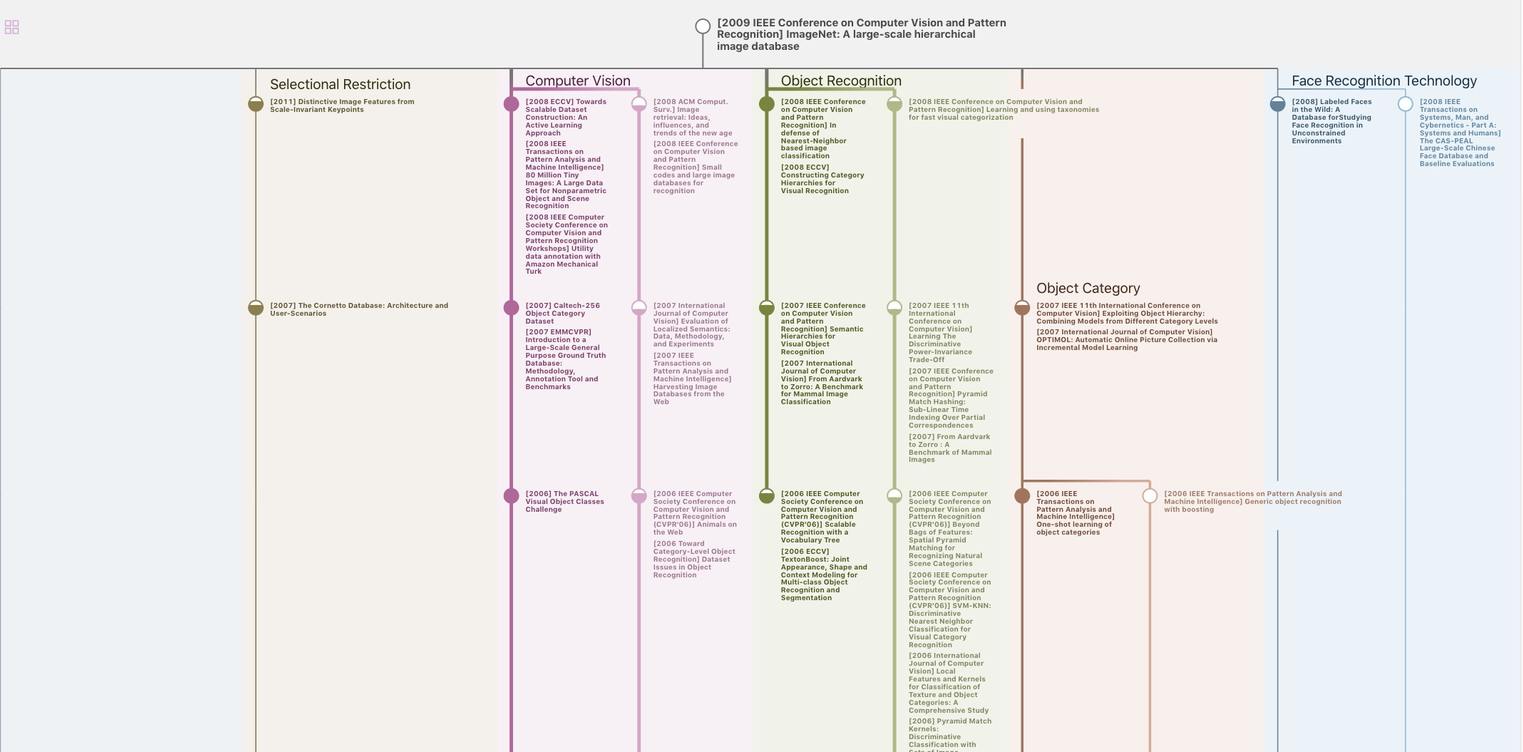
生成溯源树,研究论文发展脉络
Chat Paper
正在生成论文摘要