BMDENet: Bi-Directional Modality Difference Elimination Network for Few-Shot RGB-T Semantic Segmentation
IEEE Transactions on Circuits and Systems II: Express Briefs(2023)
摘要
Few-shot semantic segmentation (FSS) aims to segment the target prospects of query images using a few labeled support samples. Compared with the fully-supervised methods, FSS has a greater ability to generalize to unseen classes and reduce the pressure to label large pixel-level datasets. To cope with the complex outdoor lighting environment, we introduce the thermal infrared images (T) to the FSS task. However, the existing RGB-T FSS methods all ignore the differences between various modalities for direct fusion, which may hinder cross-modal information interaction. Also considering the effect of successive downsampling on the results, we propose a bi-directional modality difference elimination network (BMDENet) to boost the segmentation performance. Concretely, the bi-directional modality difference elimination module (BMDEM) reduces the heterogeneity between RGB and thermal images in the prototype space. The residual attention fusion module (RAFM) mines the bimodal features to fully fuse the cross-modal information. In addition, the mainstay and subsidiary enhancement module (MSEM) enhances the fusion features for the existing problem of the advanced model. Extensive experiments on Tokyo Multi-Spectral-
$4^{\mathrm{ i}}$
dataset prove that BMDENet achieves the state-of-the-art on both 1- and 5-shot settings.
更多查看译文
关键词
segmentation,bi-directional,few-shot
AI 理解论文
溯源树
样例
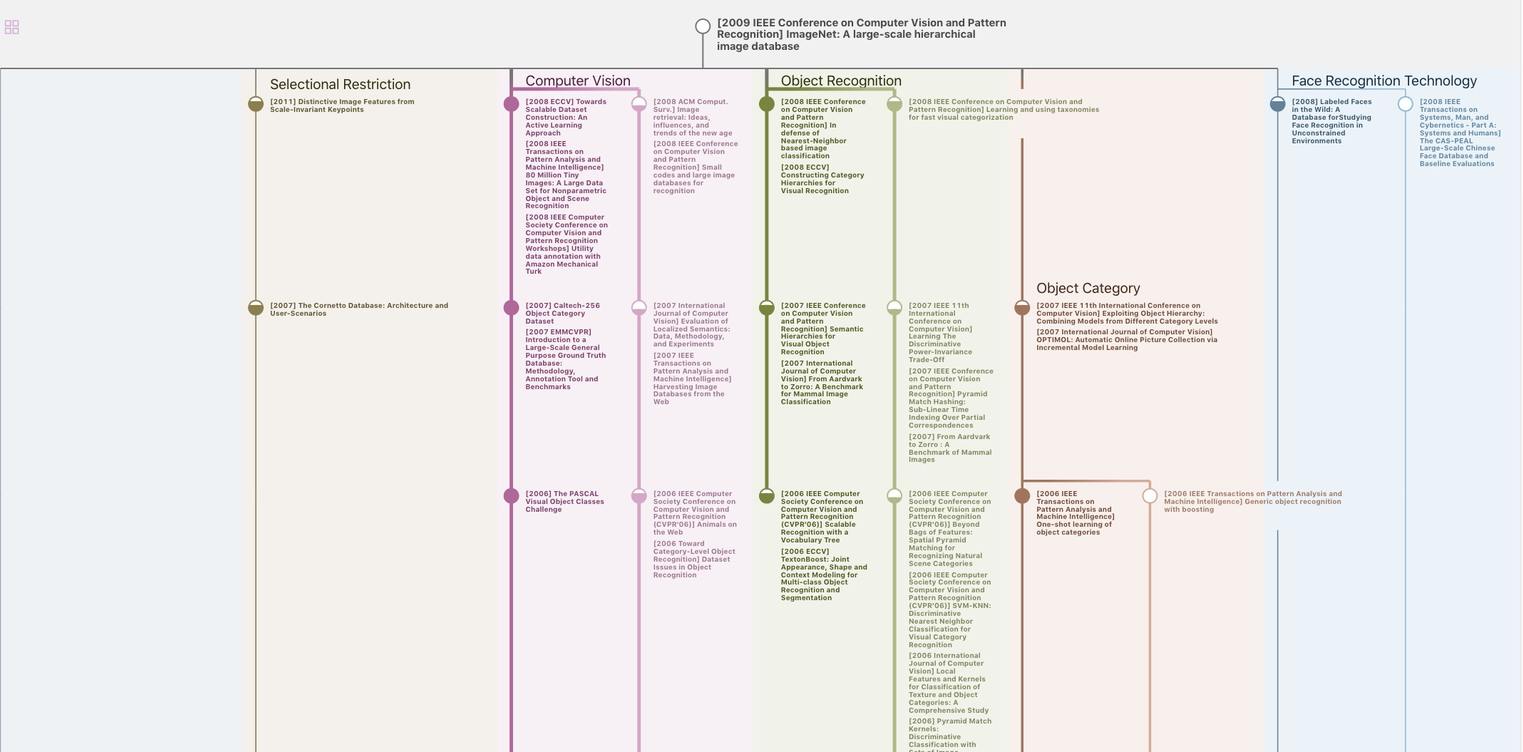
生成溯源树,研究论文发展脉络
Chat Paper
正在生成论文摘要