The Impact of Dynamic Learning on Adversarial Attacks in Networks (IEEE CNS 23 Poster)
2023 IEEE Conference on Communications and Network Security (CNS)(2023)
摘要
The widespread utilization of Machine Learning (ML) in Network Intrusion Detection Systems (NIDS) provides automated and highly accurate data processing. However, the vulnerability of ML to adversarial attacks, designed to manipulate its predictions, raises concerns. Unlike the conventional focus on computer vision, recent attention has shifted towards assessing the feasibility of such attacks in network security. This poster presents a taxonomy outlining the practical challenges of adversarial attacks on ML-based NIDS and an initial investigation into the impact of continuous training on these attacks. Results suggest that re-training, even without adversarial training, can mitigate the effects of attacks. This work aims to highlight the gap between research and real-world practicality in this field.
更多查看译文
关键词
machine learning,continuous machine learning,adversarial attacks,network intrusion detection systems
AI 理解论文
溯源树
样例
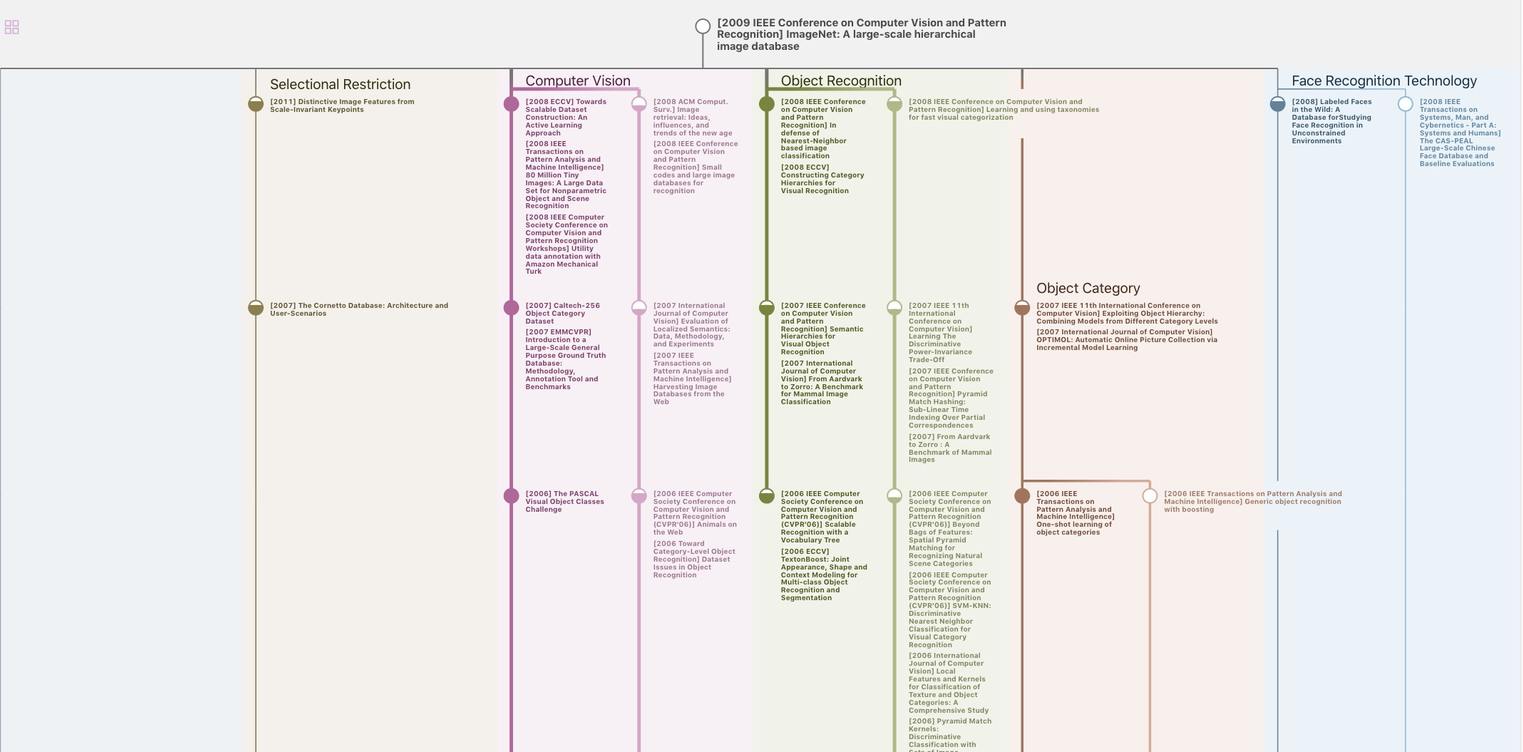
生成溯源树,研究论文发展脉络
Chat Paper
正在生成论文摘要