Assessment of Hybrid Transfer Learning Method for Forecasting EV Profile and System Voltage Using Limited EV Charging Data
Sustainable Energy, Grids and Networks(2023)
摘要
The number of electric vehicles (EV) is increasing exponentially, significantly affecting the planning and operation of future electricity grids, albeit the availability of EV data is very limited to perform power system studies. To overcome the poor forecasting accuracy associated with limited available data, this research proposes a time-series-based hybrid transfer learning forecasting approach, namely CNN-BiLSTM (Convolutional Neural Network – Bidirectional Long Short-Term Memory) to forecast EV charging profile. Additionally, the proposed algorithm has been applied to forecast network voltage from the EV data without performing power flow. EV charging demand datasets collected over a year for residential, slow commercial, and fast commercial charging stations and their corresponding voltage profiles have been used to test the effectiveness of the proposed hybrid transfer learning framework. The results confirm the improved accuracy of the proposed hybrid CNN-BiLSTM model compared to the conventional CNN model, newly created models in predicting the EV charging demand and voltage profiles.
更多查看译文
关键词
Electric vehicle,Forecasting,Transfer learning,Time series,Voltage profile
AI 理解论文
溯源树
样例
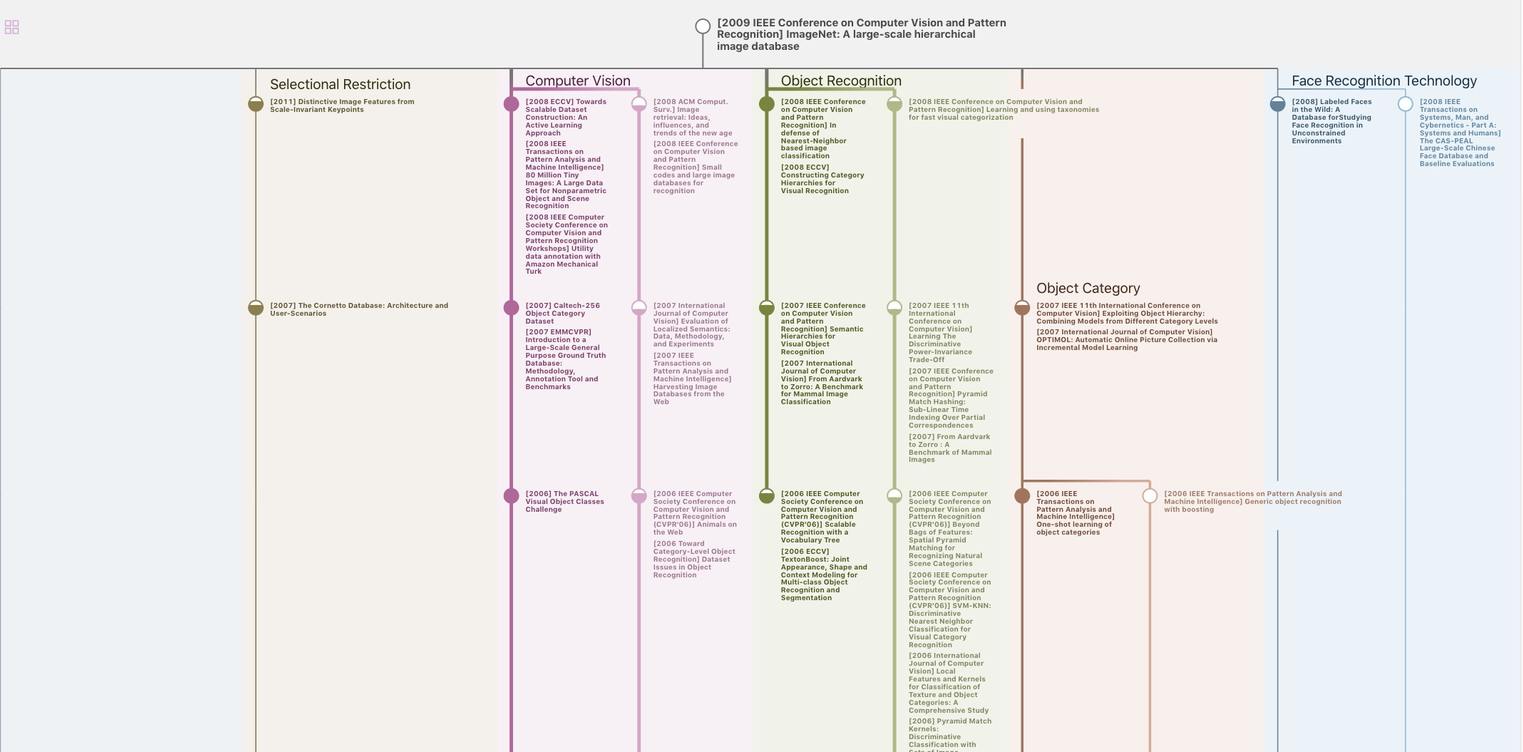
生成溯源树,研究论文发展脉络
Chat Paper
正在生成论文摘要