Controllable Unsupervised Snow Synthesis by Latent Style Space Manipulation.
Sensors (Basel, Switzerland)(2023)
摘要
In the field of intelligent vehicle technology, there is a high dependence on images captured under challenging conditions to develop robust perception algorithms. However, acquiring these images can be both time-consuming and dangerous. To address this issue, unpaired image-to-image translation models offer a solution by synthesizing samples of the desired domain, thus eliminating the reliance on ground truth supervision. However, the current methods predominantly focus on single projections rather than multiple solutions, not to mention controlling the direction of generation, which creates a scope for enhancement. In this study, we propose a generative adversarial network (GAN)-based model, which incorporates both a style encoder and a content encoder, specifically designed to extract relevant information from an image. Further, we employ a decoder to reconstruct an image using these encoded features, while ensuring that the generated output remains within a permissible range by applying a self-regression module to constrain the style latent space. By modifying the hyperparameters, we can generate controllable outputs with specific style codes. We evaluate the performance of our model by generating snow scenes on the Cityscapes and the EuroCity Persons datasets. The results reveal the effectiveness of our proposed methodology, thereby reinforcing the benefits of our approach in the ongoing evolution of intelligent vehicle technology.
更多查看译文
关键词
intelligent vehicles, snow scenes, unpaired image-to-image translation, diversity, style latent space, Gaussian distribution
AI 理解论文
溯源树
样例
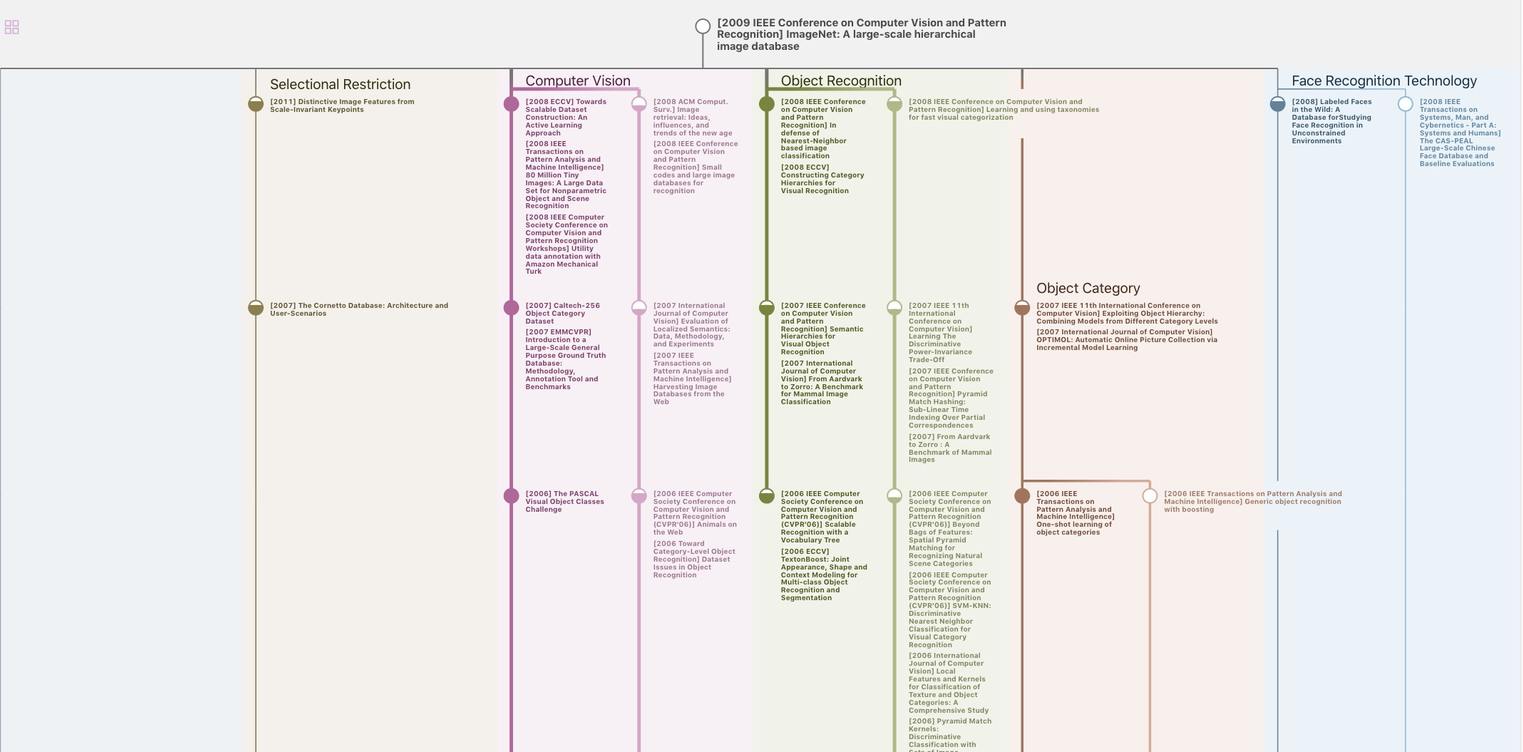
生成溯源树,研究论文发展脉络
Chat Paper
正在生成论文摘要