Full Shape Cosmology Analysis from BOSS in configuration space using Neural Network Acceleration
arXiv (Cornell University)(2023)
摘要
Recently, a new wave of full modeling analyses have emerged within the Large-Scale Structure community, leading mostly to tighter constraints on the estimation of cosmological parameters, when compared with standard approaches used over the last decade by collaboration analyses of stage III experiments. However, the majority of these full-shape analyses have primarily been conducted in Fourier space, with limited emphasis on exploring the configuration space. Investigating n-point correlations in configuration space demands a higher computational cost compared to Fourier space because it typically requires an additional integration step. This can pose a limitation when using these approaches, especially when considering higher-order statistics. One avenue to mitigate the high computation time is to take advantage of neural network acceleration techniques. In this work, we present a full shape analysis of Sloan Digital Sky Survey III/BOSS in configuration space using a neural network accelerator. We show that the efficacy of the pipeline is enhanced by a time factor $10^{3}$ without sacrificing precision, making it possible to reduce the error associated with the surrogate modeling to below $10^{-2}$ percent which is compatible with the precision required for current stage IV experiments such as DESI. We find $\Omega_m=0.286\pm 0.009$, $H_0=68.8\pm 1.2$ $\mathrm{km} \mathrm{s^{-1}}\mathrm{Mpc^{-1}}$ and $A_s \times 10^9 =2.09 ^{+0.25}_{-0.29}$. Our results on public BOSS data are in good agreement with BOSS official results and compatible with other independent full modeling analyses. We explore relaxing the prior on $\omega_b$ and varying $n_s$, without significant changes in the mean values of the cosmological parameters posterior distributions, but enlarging their widths. Finally, we explore the information content of the multipoles when constraining cosmological parameters.
更多查看译文
关键词
cosmology,neural network acceleration,neural network,space,shape
AI 理解论文
溯源树
样例
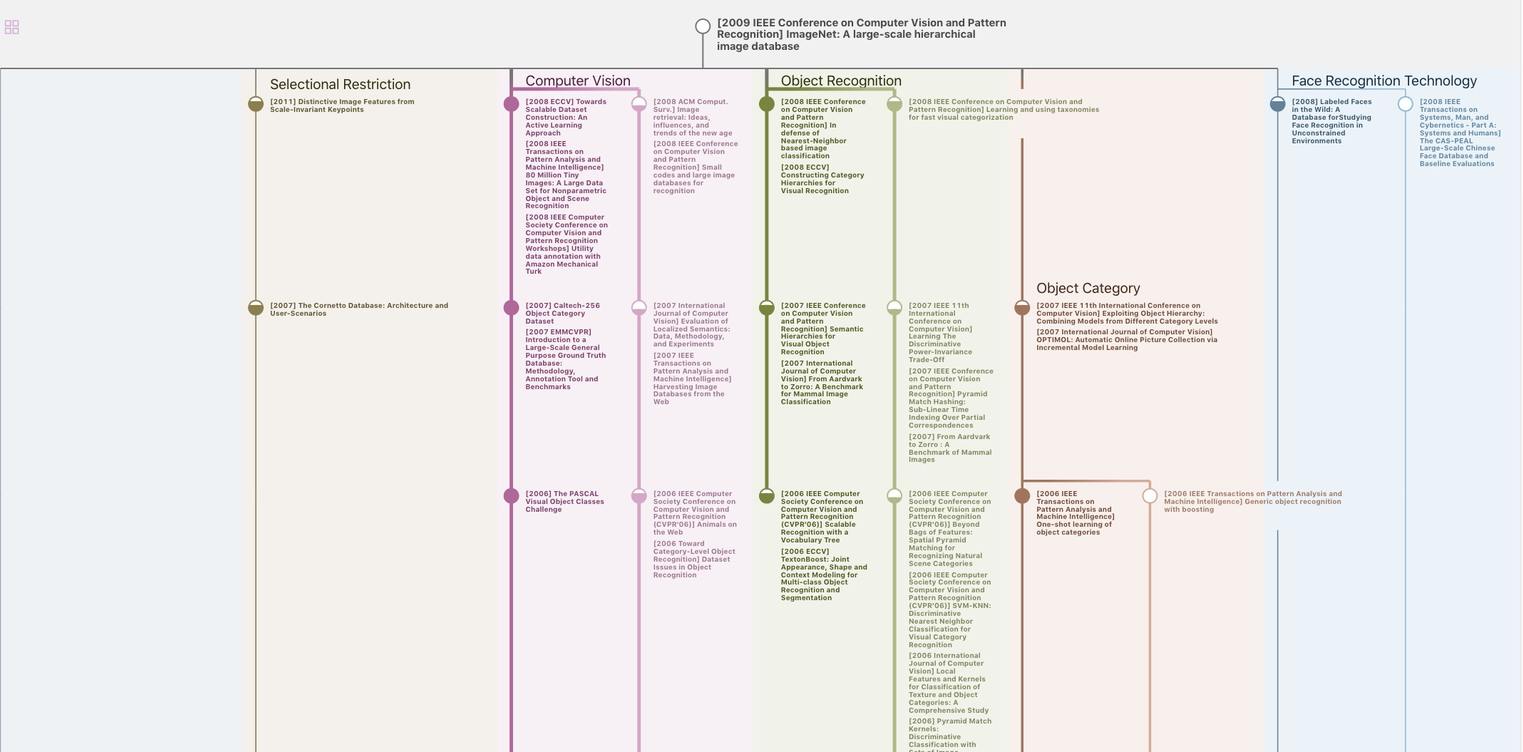
生成溯源树,研究论文发展脉络
Chat Paper
正在生成论文摘要