Reconstructive Latent-Space Neural Radiance Fields for Efficient 3D Scene Representations
CoRR(2023)
摘要
Neural Radiance Fields (NeRFs) have proven to be powerful 3D representations, capable of high quality novel view synthesis of complex scenes. While NeRFs have been applied to graphics, vision, and robotics, problems with slow rendering speed and characteristic visual artifacts prevent adoption in many use cases. In this work, we investigate combining an autoencoder (AE) with a NeRF, in which latent features (instead of colours) are rendered and then convolutionally decoded. The resulting latent-space NeRF can produce novel views with higher quality than standard colour-space NeRFs, as the AE can correct certain visual artifacts, while rendering over three times faster. Our work is orthogonal to other techniques for improving NeRF efficiency. Further, we can control the tradeoff between efficiency and image quality by shrinking the AE architecture, achieving over 13 times faster rendering with only a small drop in performance. We hope that our approach can form the basis of an efficient, yet high-fidelity, 3D scene representation for downstream tasks, especially when retaining differentiability is useful, as in many robotics scenarios requiring continual learning.
更多查看译文
关键词
scene representations,efficient 3d
AI 理解论文
溯源树
样例
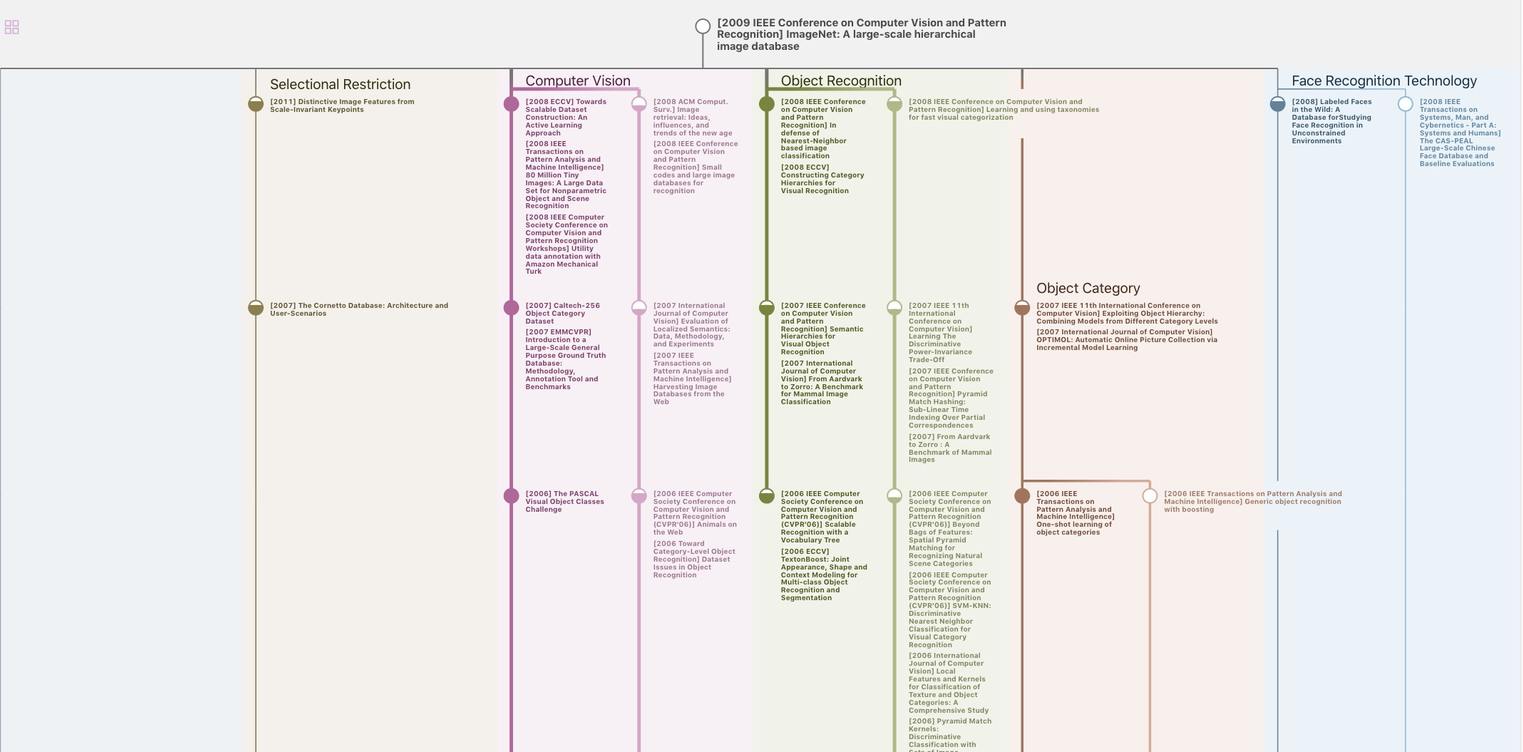
生成溯源树,研究论文发展脉络
Chat Paper
正在生成论文摘要