Gaussian boson sampling validation via detector binning
arXiv (Cornell University)(2023)
摘要
Gaussian boson sampling (GBS), a computational problem conjectured to be hard to simulate on a classical machine, has been at the forefront of recent years' experimental and theoretical efforts to demonstrate quantum advantage. The classical intractability of the sampling task makes validating these experiments a challenging and essential undertaking. In this paper, we propose binned-detector probability distributions as a suitable quantity to statistically validate GBS experiments employing photon-number-resolving detectors. We show how to compute such distributions by leveraging their connection with their respective characteristic function. The latter may be efficiently and analytically computed for squeezed input states as well as for relevant classical hypothesis like squashed states. Our scheme encompasses other validation methods based on marginal distributions and correlation functions. Additionally, it can accommodate various sources of noise, such as losses and partial distinguishability, a feature that have received limited attention within the GBS framework so far. We also illustrate how binned-detector probability distributions behave when Haar-averaged over all possible interferometric networks, extending known results for Fock boson sampling.
更多查看译文
关键词
gaussian boson,sampling,detector
AI 理解论文
溯源树
样例
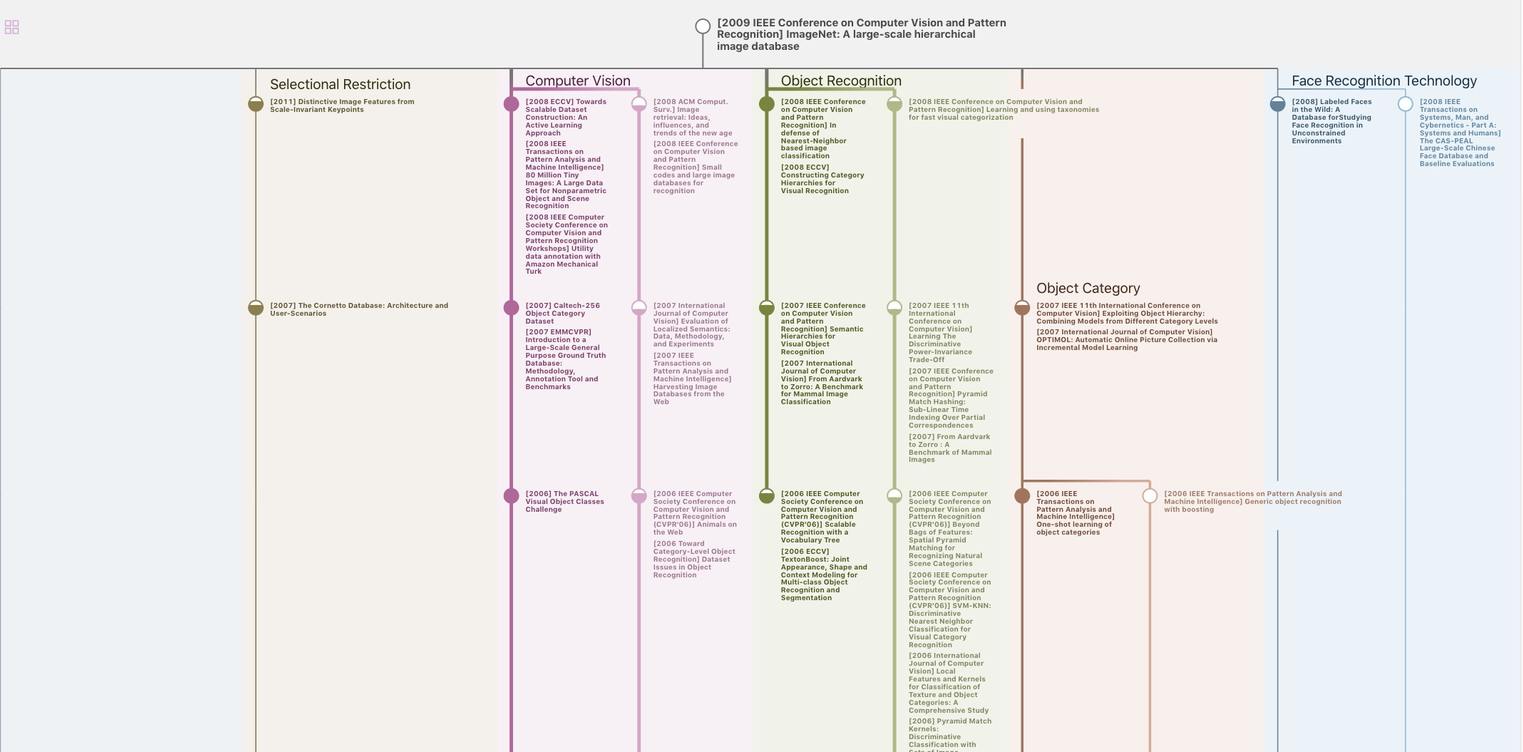
生成溯源树,研究论文发展脉络
Chat Paper
正在生成论文摘要