Deep learning with data preprocessing methods for water quality prediction in ultrafiltration
JOURNAL OF CLEANER PRODUCTION(2023)
摘要
Ultrafiltration (UF) has been widely used to remove colloidal substances and suspended solids in feed water. However, UF membrane breakage can lead to downstream impurities flow, hindering subsequent filtration such as reverse osmosis. Preliminary detection for abnormal water quality after UF is vital for cost-efficient operations, but current predictive models lack accuracy. This study investigated the predictive models using deep learning algorithms, specifically convolutional neural network (CNN) and long short-term memory (LSTM) structures. One month of data was provided from a UF system in a real seawater desalination plant. Unfortunately, conventional CNN and LSTM models struggled to predict sudden turbidity spikes caused by UF membrane damage (R-2 < 0.2351). To address this challenge, we proposed a novel approach coupling wavelet signals and raw data. This technique enriched turbidity data with abundant waveform signals, resulting in a significant improvement in predictive accuracy (R-2 < 0.9203). Shapley additive explanation demonstrated that the wavelet signals emphasized turbidity spikes, helping models in recognizing the extent of changes. This outcome of this study is the development of highly accurate predictive models for outflow turbidity after UF. These models will enhance the safety and efficiency of UF and subsequent filtration systems, improving their overall performance.
更多查看译文
关键词
Ultrafiltration,Data preprocessing,Wavelet transform,Deep learning,Convolutional neural network,Long short -term memory
AI 理解论文
溯源树
样例
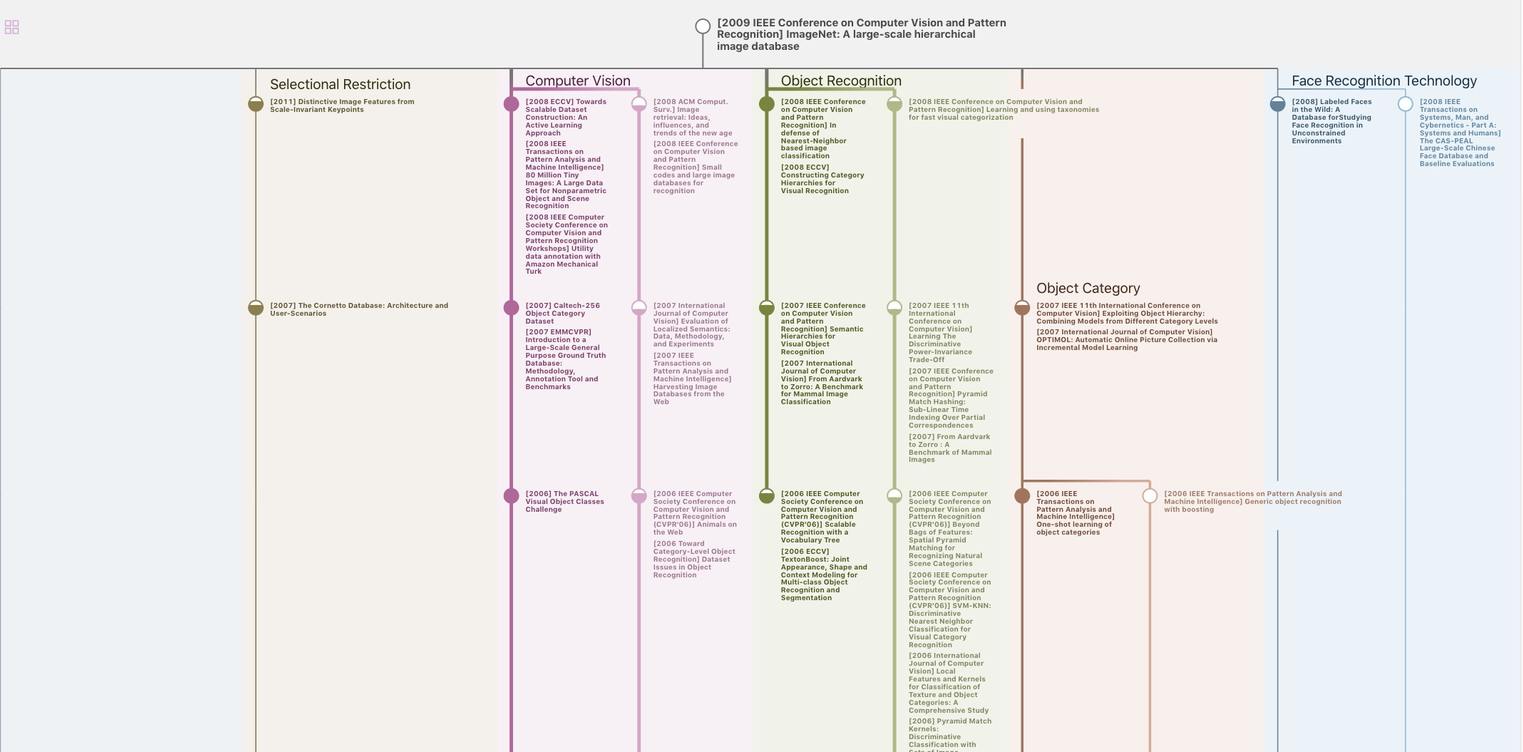
生成溯源树,研究论文发展脉络
Chat Paper
正在生成论文摘要