PSOR04 Presentation Time: 12:15 PM
Brachytherapy(2023)
摘要
Purpose For trans-rectal ultrasound (TRUS)-based high dose-rate (HDR) prostate brachytherapy, contouring the prostate can be challenging due to poor soft-tissue contrast, implanted needle artifacts, and bleeding. This project aimed to quantify the percentage of cases in which observers preferred the artificial intelligence (AI)-generated contours over the clinical contours, and to assess the potential of AI-assisted contouring as an educational tool for residents. Materials and Methods We conducted a single retrospective study of 150 patients, who underwent HDR brachytherapy as a monotherapy, boost, or salvage with a single radiation oncologist (MK). We downloaded TRUS images after needle-insertion and clinical target volume (CTV) contours utilized for treatment planning onto a research workstation. Since the CTV for high-risk disease often included a portion of the seminal vesicle, we truncated the CTV to include only the prostate on the research workstation, and labeled the CTV as the reference contour. We prepared a UNet to provide prostate contours from TRUS images utilizing 100 patients in the training set and 20 patients in the validation set. We then applied the UNet to a testing dataset of 20 patients. We calculated the Dice coefficient between the AI and the reference contours. Then, we recruited 8 observers, 3 of whom were attending physicians experienced in prostate brachytherapy (PN, PO, MD), and 5 of whom were residents (TC, AM, DR, TW, and AM) with prostate brachytherapy experience. For each observer, we presented TRUS images with both reference and AI contours for the 20 test cases. The contours were labeled as ‘R’ or ‘B’ and were scrambled with respect to their source (reference or AI). We asked the observer to choose which contour set (R or B) was preferred for clinical use. We also asked the observer to rate the contour corresponding to the AI contour on a 4-point rating scale (0 = no edits, 1 = minimal edits, 2 = moderate edits, 3 major edits). We calculated the percentage of cases, in which the observer preferred the AI contour. We also calculated the percentage of AI contours requiring no or minimal edits. Finally, we asked 5 residents to provide prostate contours for 10 cases prior to visualizing any AI contours. In a subsequent session, we asked residents to edit AI contours. For each session, we computed the average percentage of cases in which the Dice coefficient between the AI and resident contours was greater than 0.85. Results The median Dice coefficient between AI and reference contours was 0.93 (inter-quartile range (IQR): 0.91, 0.94). Observers preferred AI contours for a median of 57.5% (IQR: 47.5, 65.0) of the test cases. Six of 8 observers preferred AI contours in the majority (>=10 of the 20 test cases), while the remaining two preferred AI contours in 35% and 45% of the cases. The mean percentage of cases, in which observers requested no or minimal edits, was 72.5% (IQR: 66.3, 86.3). Among the 5 resident observers, the average percentages of cases with Dice coefficients >= 0.85 were 17% without and 100% with AI contours available (p < 0.01). Conclusions Observers preferred the AI contours to the clinical contours in the majority of cases. AI algorithms have the potential to aid radiation oncologists, including residents, in defining the prostate CTV prior to treatment planning. Further prospective studies are needed to identify how to best integrate AI prostate contours into the HDR brachytherapy workflow. For trans-rectal ultrasound (TRUS)-based high dose-rate (HDR) prostate brachytherapy, contouring the prostate can be challenging due to poor soft-tissue contrast, implanted needle artifacts, and bleeding. This project aimed to quantify the percentage of cases in which observers preferred the artificial intelligence (AI)-generated contours over the clinical contours, and to assess the potential of AI-assisted contouring as an educational tool for residents. We conducted a single retrospective study of 150 patients, who underwent HDR brachytherapy as a monotherapy, boost, or salvage with a single radiation oncologist (MK). We downloaded TRUS images after needle-insertion and clinical target volume (CTV) contours utilized for treatment planning onto a research workstation. Since the CTV for high-risk disease often included a portion of the seminal vesicle, we truncated the CTV to include only the prostate on the research workstation, and labeled the CTV as the reference contour. We prepared a UNet to provide prostate contours from TRUS images utilizing 100 patients in the training set and 20 patients in the validation set. We then applied the UNet to a testing dataset of 20 patients. We calculated the Dice coefficient between the AI and the reference contours. Then, we recruited 8 observers, 3 of whom were attending physicians experienced in prostate brachytherapy (PN, PO, MD), and 5 of whom were residents (TC, AM, DR, TW, and AM) with prostate brachytherapy experience. For each observer, we presented TRUS images with both reference and AI contours for the 20 test cases. The contours were labeled as ‘R’ or ‘B’ and were scrambled with respect to their source (reference or AI). We asked the observer to choose which contour set (R or B) was preferred for clinical use. We also asked the observer to rate the contour corresponding to the AI contour on a 4-point rating scale (0 = no edits, 1 = minimal edits, 2 = moderate edits, 3 major edits). We calculated the percentage of cases, in which the observer preferred the AI contour. We also calculated the percentage of AI contours requiring no or minimal edits. Finally, we asked 5 residents to provide prostate contours for 10 cases prior to visualizing any AI contours. In a subsequent session, we asked residents to edit AI contours. For each session, we computed the average percentage of cases in which the Dice coefficient between the AI and resident contours was greater than 0.85. The median Dice coefficient between AI and reference contours was 0.93 (inter-quartile range (IQR): 0.91, 0.94). Observers preferred AI contours for a median of 57.5% (IQR: 47.5, 65.0) of the test cases. Six of 8 observers preferred AI contours in the majority (>=10 of the 20 test cases), while the remaining two preferred AI contours in 35% and 45% of the cases. The mean percentage of cases, in which observers requested no or minimal edits, was 72.5% (IQR: 66.3, 86.3). Among the 5 resident observers, the average percentages of cases with Dice coefficients >= 0.85 were 17% without and 100% with AI contours available (p < 0.01). Observers preferred the AI contours to the clinical contours in the majority of cases. AI algorithms have the potential to aid radiation oncologists, including residents, in defining the prostate CTV prior to treatment planning. Further prospective studies are needed to identify how to best integrate AI prostate contours into the HDR brachytherapy workflow.
更多查看译文
AI 理解论文
溯源树
样例
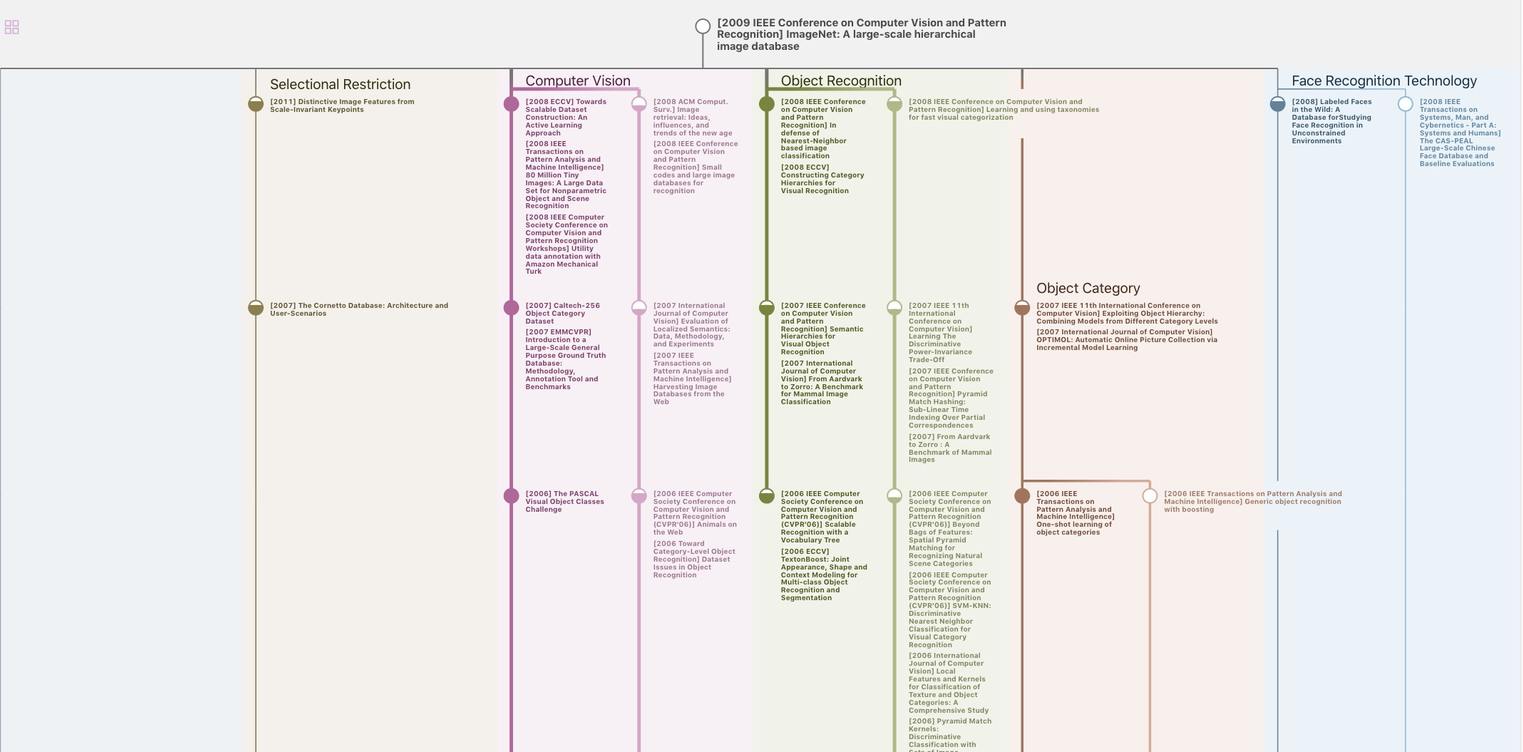
生成溯源树,研究论文发展脉络
Chat Paper
正在生成论文摘要