Physics-Informed Scaling Evolutionary Transformer for In-Situ Tool Condition Monitoring
IEEE-ASME TRANSACTIONS ON MECHATRONICS(2024)
摘要
For intelligent condition monitoring (ICM) tasks of machine tools (MTs), physics-based and data-driven models typically suffer from two major challenges that constraint their applicability: first, the complex machining parameters set up along with the incompleteness of physics-based models and second, the limited representation ability of small-scale dataset for data-driven models. Considering that it is impractical for the cases of MTs to obtain sufficient scale and well-balanced dataset due to unaffordable specimen cost and strict manufacturing schedule. Accordingly, this paper proposes a new physics-informed scaling evolutionary transformer network, abbreviated as PIS-ETN, to incorporate prior knowledge into the ICM model. Specifically, it mainly includes three parts. First, a texture digital twin (TDT) model is designed to exploit prior knowledge from machining parameters and semi-observable sensor information. Secondly, a texture knowledge embedding module is designed to enhance representation capability. Thirdly, the Pareto-optimal solution space is adopted for further architecture optimization. The experiments indicate that the designed TDT model can effectively provide rich prior empirical knowledge for the designed scaling lightweight model and accelerate model convergence. The proposed lightweight architecture with its Pareto optimal training strategy shows promising fine-grained texture representation ability.
更多查看译文
关键词
Machining,Task analysis,Trajectory,Degradation,Condition monitoring,Transformers,Stochastic processes,Intelligent condition monitoring,physics-informed model,texture digital twin (TDT),texture knowledge embedding
AI 理解论文
溯源树
样例
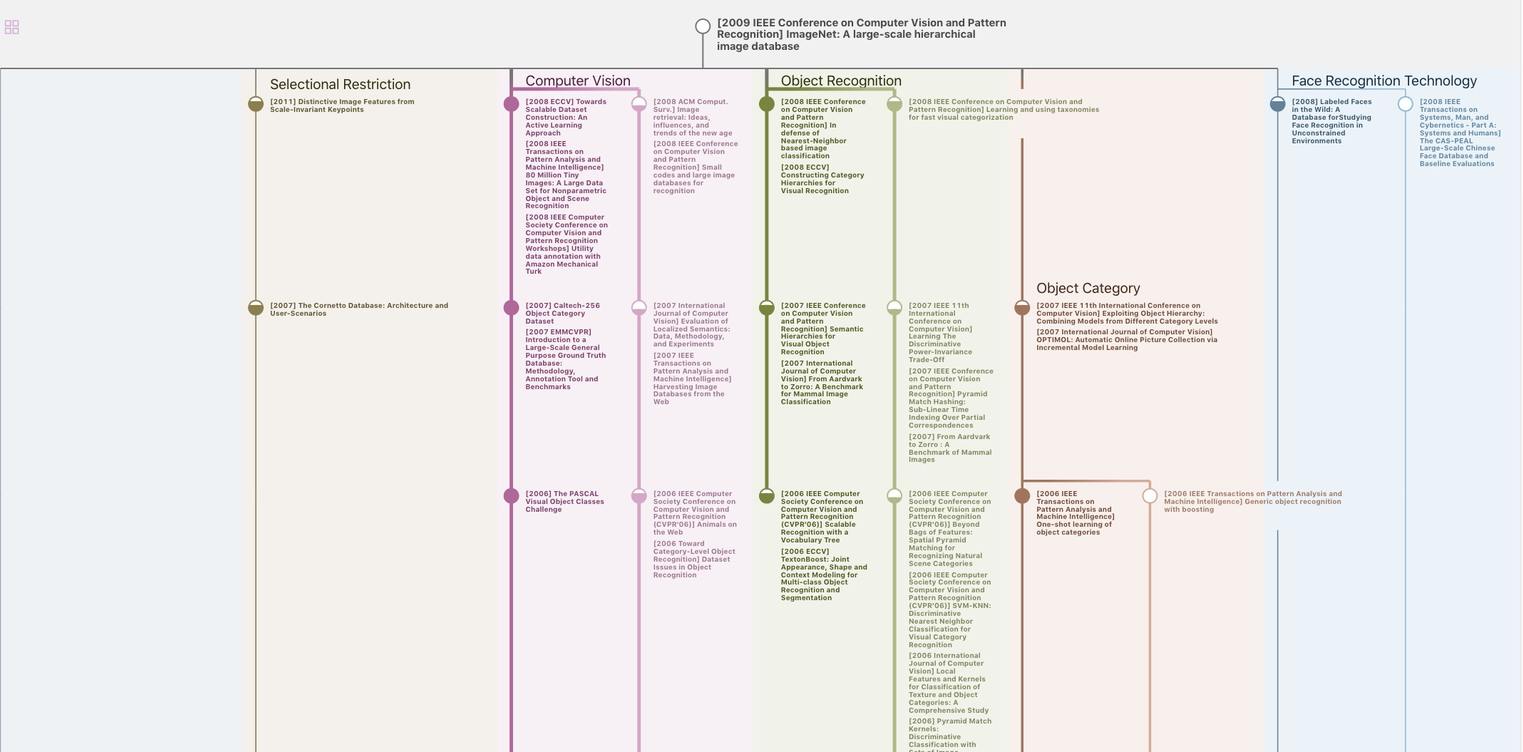
生成溯源树,研究论文发展脉络
Chat Paper
正在生成论文摘要