Subset Sensor Selection Optimization: A Genetic Algorithm Approach With Innovative Set Encoding Methods
IEEE SENSORS JOURNAL(2023)
摘要
The sensor subset selection problem is a crucial task in the field of sensor systems, where the goal is to choose a subset of sensors from a larger pool such that the spatial estimation representation closely approximates the performance of using all the sensors while minimizing the number of sensors required. This is a challenging problem involving trade-offs between performance, cost, and complexity. In this work, a novel approach is proposed, which is based on set encoding and genetic algorithm (GA) for addressing the sensor subset selection problem. Our method incorporates a spatial algorithm to minimize the mean squared error (mse) between the reference field and the selected subset of sensors. The efficiency of this approach is demonstrated by optimizing the sensor selection based on the water table (WT) variable using the dataset from the Savannah River Site (SRS) F-Area, a Department of Energy monitored location with groundwater contamination issues. The proposed solution introduces two new crossover methods [complementary set crossover (CSC) and uniform set crossover (USC)] and two new mutation methods [inclusive set mutation (ISM) and exclusive set mutation (ESM)] that work with the novel set encoding. The effectiveness of this method is compared with the previously used greedy strategy, showing considerable improvements. The implemented algorithm shows a substantial performance for a 33, 50, and 75 decrease in sensors, achieving an R-2 higher than 0.98. This indicates an extremely accurate depiction of the ground truth field using the optimized sensor subset.
更多查看译文
关键词
Genetic algorithm (GA),optimization,sensor subset selection,set encoding,spatial estimation
AI 理解论文
溯源树
样例
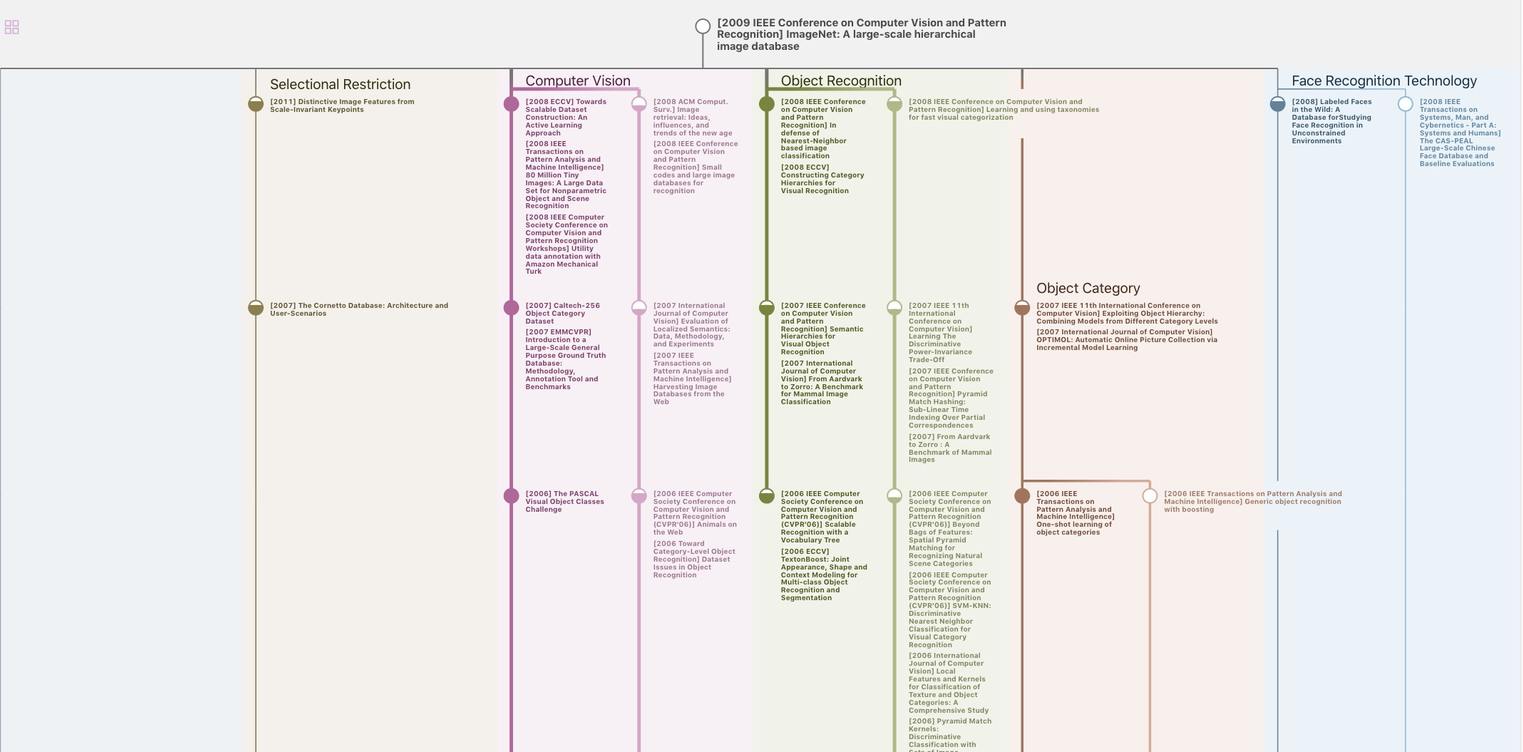
生成溯源树,研究论文发展脉络
Chat Paper
正在生成论文摘要