MultiVT: Multiple-Task Framework for Dentistry
DOMAIN ADAPTATION AND REPRESENTATION TRANSFER, DART 2023(2024)
摘要
Current image understanding methods on dental data are often trained end-to-end on inputs and labels, with focus on using state-of-the-art neural architectures. Such approaches, however, typically ignore domain specific peculiarities and lack the ability to generalize outside their training dataset. We observe that, in RGB images, teeth display a weak or unremarkable texture while exhibiting strong boundaries; similarly, in panoramic radiographs root tips and crowns are generally visible, while other parts of the teeth appear blurry. In their respective image type, these features are robust to the domain shift given by different sources or acquisition tools. Therefore, we formulate a method which we call MultiVT, able to leverage these low level image features to achieve results with good domain generalization properties. We demonstrate with experiments that, by focusing on such domain-robust features, we can achieve better segmentation and detection results. Additionally, MultiVT improves generalization capabilities without applying domain adaptive techniques - a characteristic which renders our method suitable for use in real-world applications.
更多查看译文
关键词
Dental Image Understanding,Domain Generalization,Instance Segmentation,Object Detection
AI 理解论文
溯源树
样例
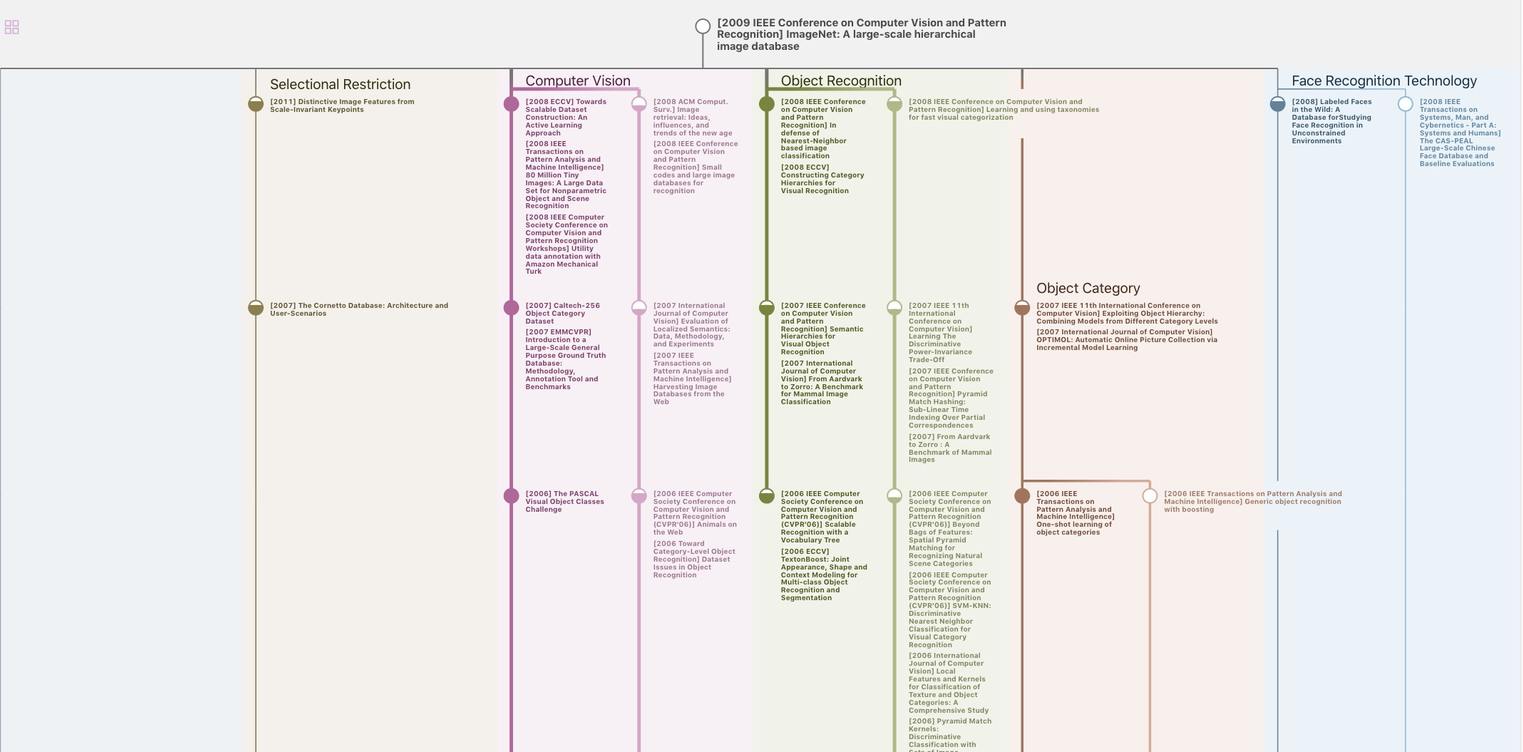
生成溯源树,研究论文发展脉络
Chat Paper
正在生成论文摘要