Semi-Supervised Semantic Segmentation with Pixel-Level Contrastive Learning from a Class-wise Memory Bank
arXiv (Cornell University)(2021)
摘要
This work presents a novel approach for semi-supervised semantic segmentation. The key element of this approach is our contrastive learning module that enforces the segmentation network to yield similar pixel-level feature representations for same-class samples across the whole dataset. To achieve this, we maintain a memory bank continuously updated with relevant and high-quality feature vectors from labeled data. In an end-to-end training, the features from both labeled and unlabeled data are optimized to be similar to same-class samples from the memory bank. Our approach outperforms the current state-of-the-art for semi-supervised semantic segmentation and semi-supervised domain adaptation on well-known public benchmarks, with larger improvements on the most challenging scenarios, i.e., less available labeled data. https://github.com/Shathe/SemiSeg-Contrastive
更多查看译文
关键词
segmentation,memory,learning,semi-supervised,pixel-level,class-wise
AI 理解论文
溯源树
样例
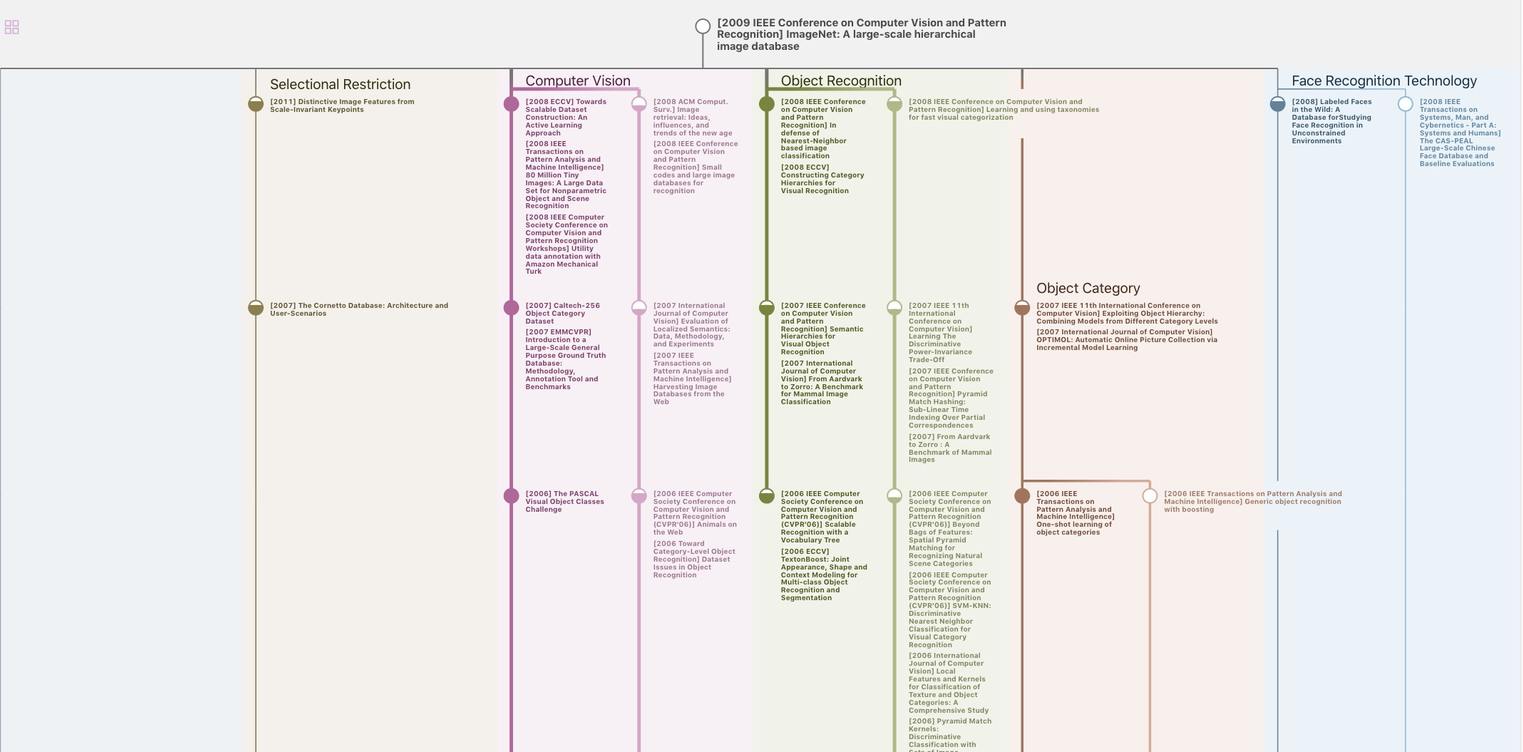
生成溯源树,研究论文发展脉络
Chat Paper
正在生成论文摘要