Machine Learning-based Beamforming Design for Millimeter Wave IRS Communications with Discrete Phase Shifters
arXiv (Cornell University)(2022)
摘要
In this paper, we investigate an intelligent reflecting surface (IRS)-assisted millimeter-wave multiple-input single-output downlink wireless communication system. By jointly calculating the active beamforming at the base station and the passive beamforming at the IRS, we aim to minimize the transmit power under the constraint of each user' signal-to-interference-plus-noise ratio. To solve this problem, we propose a low-complexity machine learning-based cross-entropy (CE) algorithm to alternately optimize the active beamforming and the passive beamforming. Specifically, in the alternative iteration process, the zero-forcing (ZF) method and CE algorithm are applied to acquire the active beamforming and the passive beamforming, respectively. The CE algorithm starts with random sampling, by the idea of distribution focusing, namely shifting the distribution towards a desired one by minimizing CE, and a near optimal reflection coefficients with adequately high probability can be obtained. In addition, we extend the original one-bit phase shift at the IRS to the common case with high-resolution phase shift to enhance the effectiveness of the algorithms. Simulation results verify that the proposed algorithm can obtain a near optimal solution with lower computational complexity.
更多查看译文
关键词
millimeter wave irs
AI 理解论文
溯源树
样例
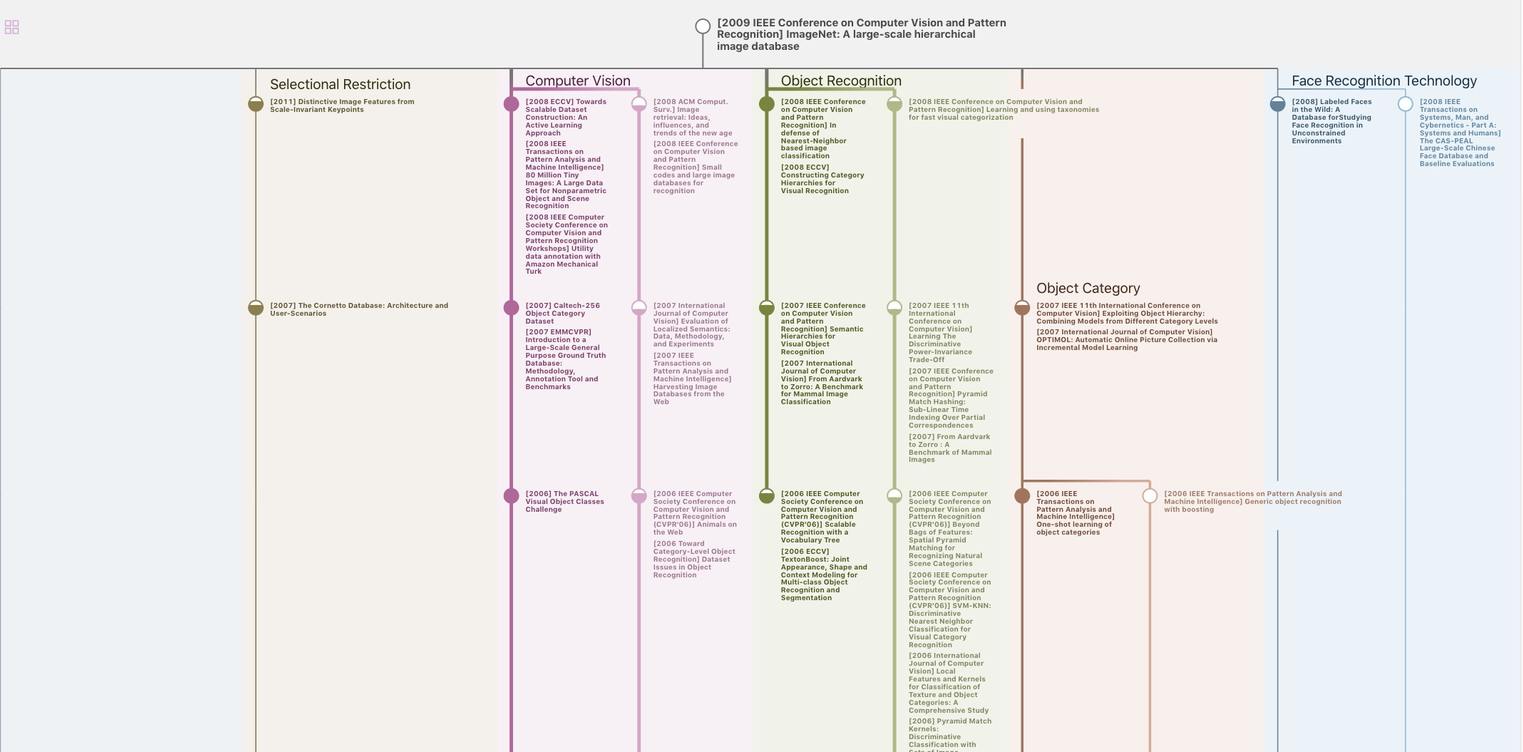
生成溯源树,研究论文发展脉络
Chat Paper
正在生成论文摘要