Autism Detection of MRI Brain Images Using Hybrid Deep CNN With DM-Resnet Classifier
IEEE Access(2023)
摘要
The neurodevelopmental Autism Spectrum Disorder (ASD) causes problems in social communication. Earlier diagnosis of ASD from brain image is necessary for reducing the effect of disorder. In this paper, deep Convolutional Neural Network (CNN) with Dwarf Mongoose optimized Residual Network (DM-ResNet) is proposed for the classification of autism disorder from Magnetic Resonance Imaging (MRI) brain images. Initially, the input brain images are preprocessed to remove the non-brain tissues. The preprocessed images are segmented with hybrid Fuzzy C Means (FCM) and Gaussian Mixture Model (GMM) which partition the image into sub groups to make it easier for classification by reducing the complexity. FCM-GMM segments the volume into predefined cortical and sub cortical regions. After segmentation, the features are extracted with Visual Geometry Group (VGG)-16 networks which comprised of several tiny kernels with filters for enhancing the depth of network and permit to extract complicated and discriminative features. Region of Interest (ROI) based functional connectivity feature is extracted with VGG-16 and these features are classified with DM optimized ResNet. The hyper parameters are optimized with DM optimization algorithm which improves the accuracy of classifier. By using the proposed approach, the accuracy of autism detection is improved to 99.83%.
更多查看译文
关键词
Feature extraction,Magnetic resonance imaging,Image segmentation,Autism,Signal processing algorithms,Deep learning,Classification algorithms,Autism detection,MRI images,segmentation,VGG feature extraction,ResNet,dwarf mongoose optimization
AI 理解论文
溯源树
样例
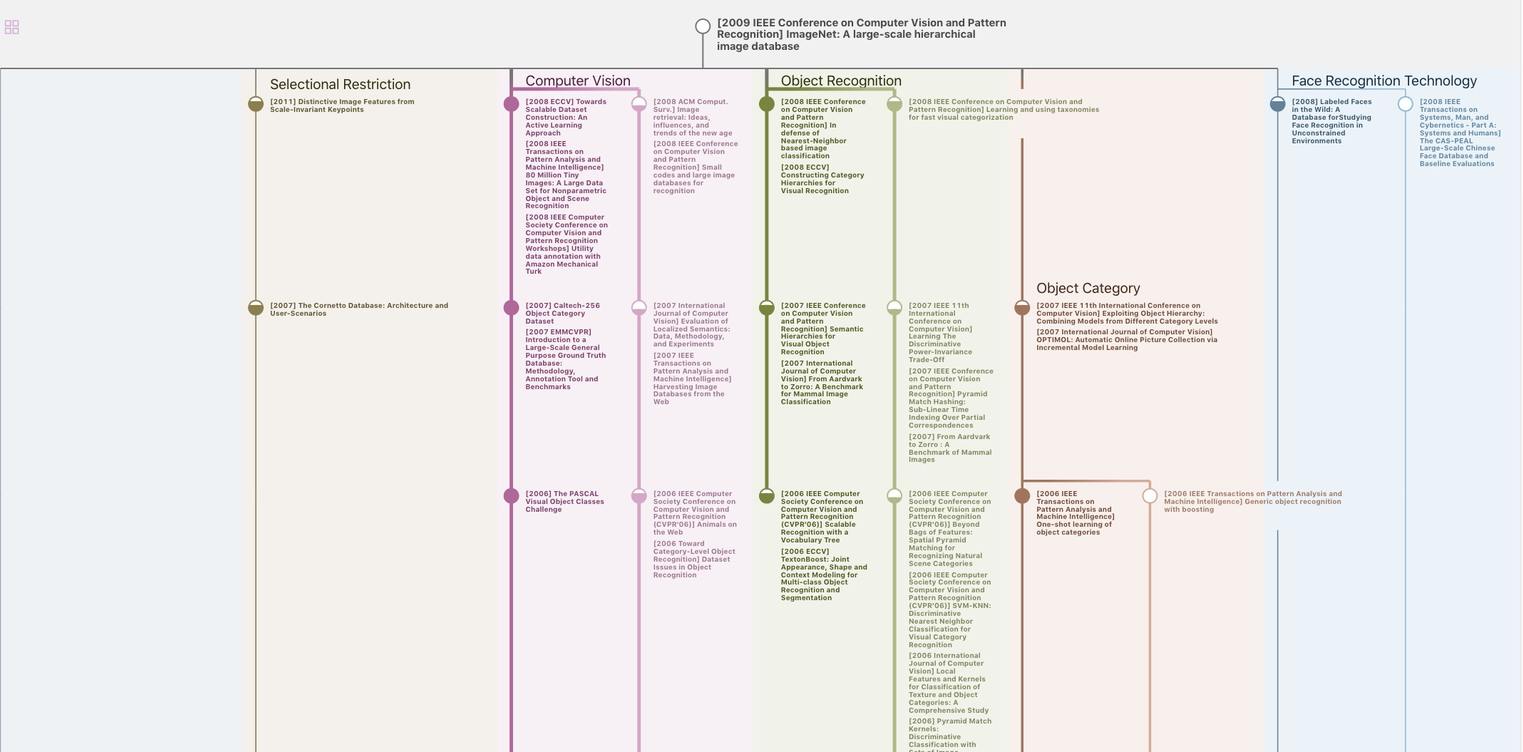
生成溯源树,研究论文发展脉络
Chat Paper
正在生成论文摘要