Debiasing Algorithm through Model Adaptation
ICLR 2024(2023)
摘要
Large language models are becoming the go-to solution for various language tasks. However, with growing capacity, models are prone to rely on spurious correlations stemming from biases and stereotypes present in the training data. This work proposes a novel method for detecting and mitigating gender bias in language models. We perform causal analysis to identify problematic model components and discover that mid-upper feed-forward layers are most prone to convey biases. Based on the analysis results, we adapt the model by multiplying these layers by a linear projection. Our titular method, DAMA, significantly decreases bias as measured by diverse metrics while maintaining the model's performance on downstream tasks. We release code for our method and models, which retrain LLaMA's state-of-the-art performance while being significantly less biased.
更多查看译文
关键词
Model Editingi,Gender Bias,Causal Inference
AI 理解论文
溯源树
样例
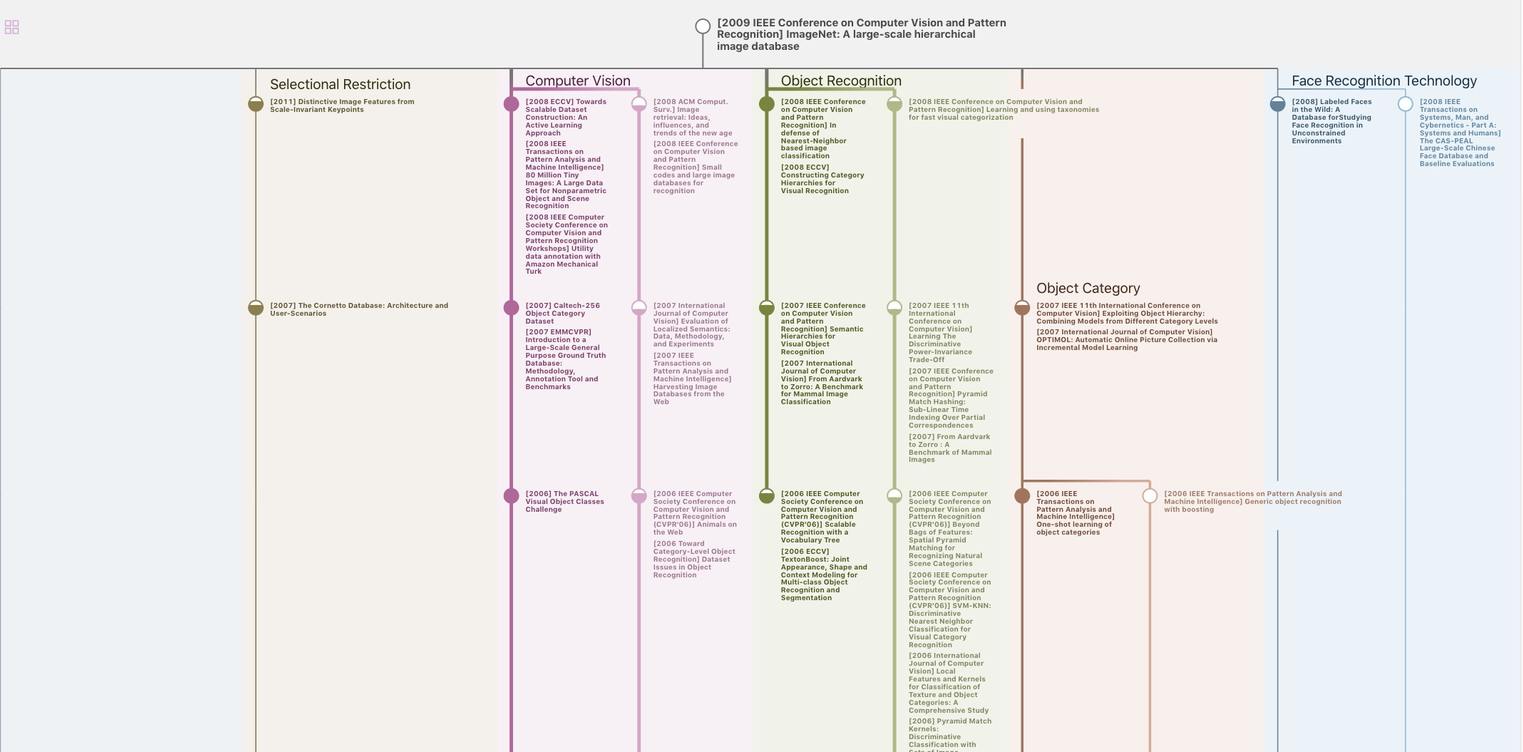
生成溯源树,研究论文发展脉络
Chat Paper
正在生成论文摘要