A deep learning framework for predicting the neutralizing activity of COVID-19 therapeutics and vaccines against evolving SARS-CoV-2 variants
biorxiv(2023)
摘要
Understanding how viral variants evade neutralization is crucial for improving antibody-based treatments, especially with rapidly evolving viruses like SARS-CoV-2. Yet, conventional assays are limited in the face of rapid viral evolution, relying on a narrow set of viral isolates, and falling short in capturing the full spectrum of variants. To address this, we have developed a deep learning approach to predict changes in neutralizing antibody activity of COVID-19 therapeutics and vaccines against emerging viral variants. First, we trained a variational autoencoder (VAE) using all 67,885 unique SARS-CoV-2 spike protein sequences from the NCBI virus (up to October 31, 2022) database to encode spike protein variants into a latent space. Using this VAE and a curated dataset of 7,069 in vitro assay data points from the NCATS OpenData Portal, we trained a neural network regression model to predict fold changes in neutralizing activity of 40 COVID-19 therapeutics and vaccines against spike protein sequence variants, relative to their neutralizing activity against the ancestral strain (Wuhan-Hu-1). Our model also employs Bayesian inference to quantify prediction uncertainty, providing more nuanced and informative estimates. To validate the model's predictive capacity, we assessed its performance on a test set of in vitro assay data collected up to eight months after the data included in the model training (N = 980). The model accurately predicted fold changes in neutralizing activity for this prospective dataset, with an R2 of 0.77. Expanding our methodology to include all available data from NCBI virus and NCATS OpenData Portal up to date, we assessed predicted changes in activity for current COVID-19 monoclonal antibodies and vaccines against newly identified SARS-CoV-2 lineages. Our predictions suggest that current therapeutic and vaccine-induced antibodies will have significantly reduced activity against newer XBB descendants, notably EG.5, FL.1.5.1, and XBB.1.16. Using the model, we were able to primarily attribute the observed predicted loss in activity to the F456L spike mutation found in EG.5 and FL.1.5.1 sequences. Conversely, mRNA-bivalent vaccines are predicted to be less susceptible to the recent BA.2.86 variant compared to new XBB descendants. These findings align closely with recent research, underscoring the potential of deep learning in shaping therapeutic and vaccine strategies for emerging viral variants.
### Competing Interest Statement
RM, ES, MN, KM, DP, RS, AV, and VS are employees of nference and have financial interests in the company and in the successful application of this research. VS is an employee of nference and Anumana and has financial interests in these companies and in the successful application of this research.
更多查看译文
AI 理解论文
溯源树
样例
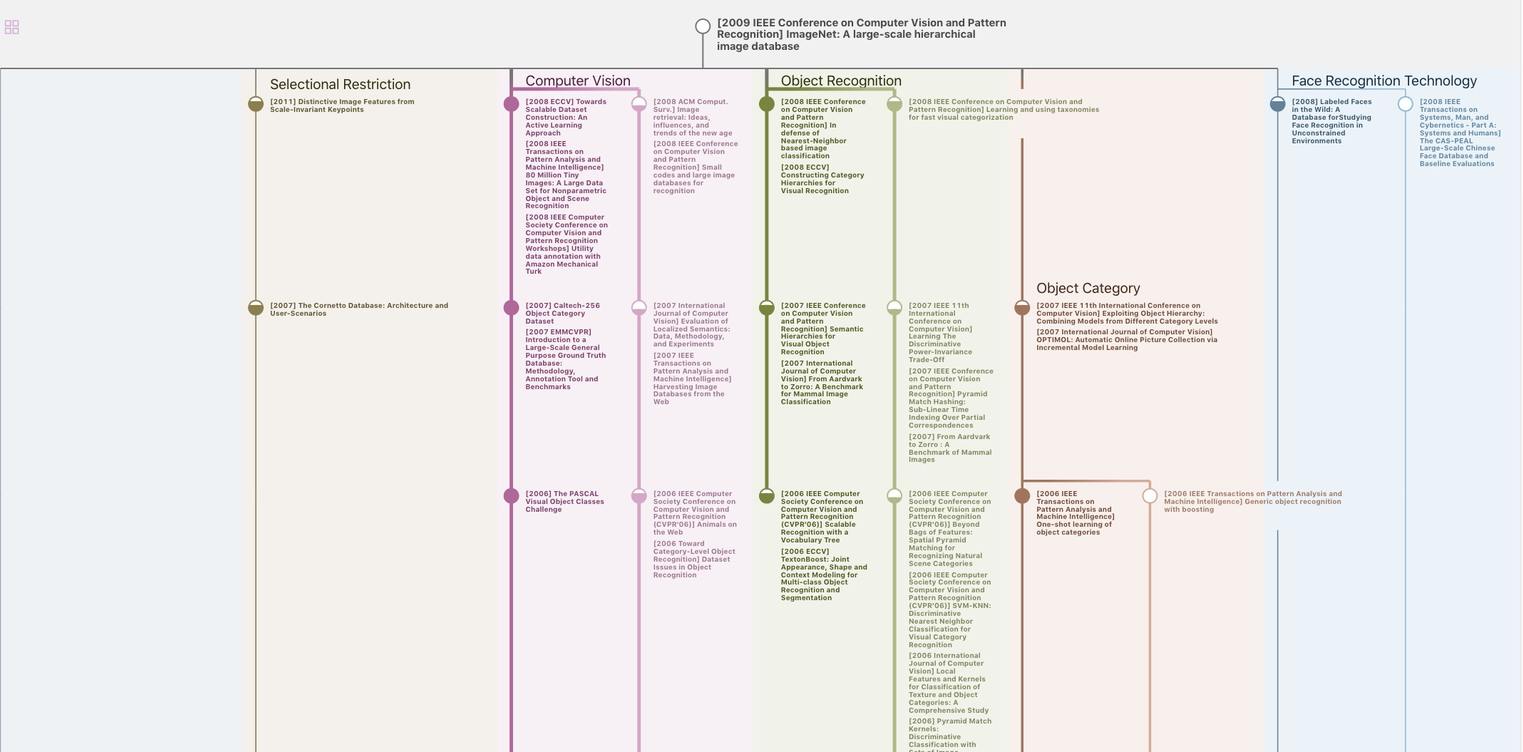
生成溯源树,研究论文发展脉络
Chat Paper
正在生成论文摘要