Negative Binomial Mixture Model for Identification of Noise in Antigen-Specificity Predictions by LIBRA-seq.
bioRxiv : the preprint server for biology(2023)
摘要
Motivation:LIBRA-seq (linking B cell receptor to antigen specificity by sequencing) provides a powerful tool for interrogating the antigen-specific B cell compartment and identifying antibodies against antigen targets of interest. Identification of noise in LIBRA-seq antigen count data is critical for improving antigen binding predictions for downstream applications including antibody discovery and machine learning technologies.
Results:In this study, we present a method for denoising LIBRA-seq data by clustering antigen counts into signal and noise components with a negative binomial mixture model. This approach leverages the VRC01 negative control cells included in a recent LIBRA-seq study(Abu-Shmais et al.) to provide a data-driven means for identification of technical noise. We apply this method to a dataset of nine donors representing separate LIBRA-seq experiments and show that our approach provides improved predictions for in vitro antibody-antigen binding when compared to the standard scoring method used in LIBRA-seq, despite variance in data size and noise structure across samples. This development will improve the ability of LIBRA-seq to identify antigen-specific B cells and contribute to providing more reliable datasets for future machine learning based approaches to predicting antibody-antigen binding as the corpus of LIBRA-seq data continues to grow.
更多查看译文
关键词
noise,antigen-specificity,libra-seq
AI 理解论文
溯源树
样例
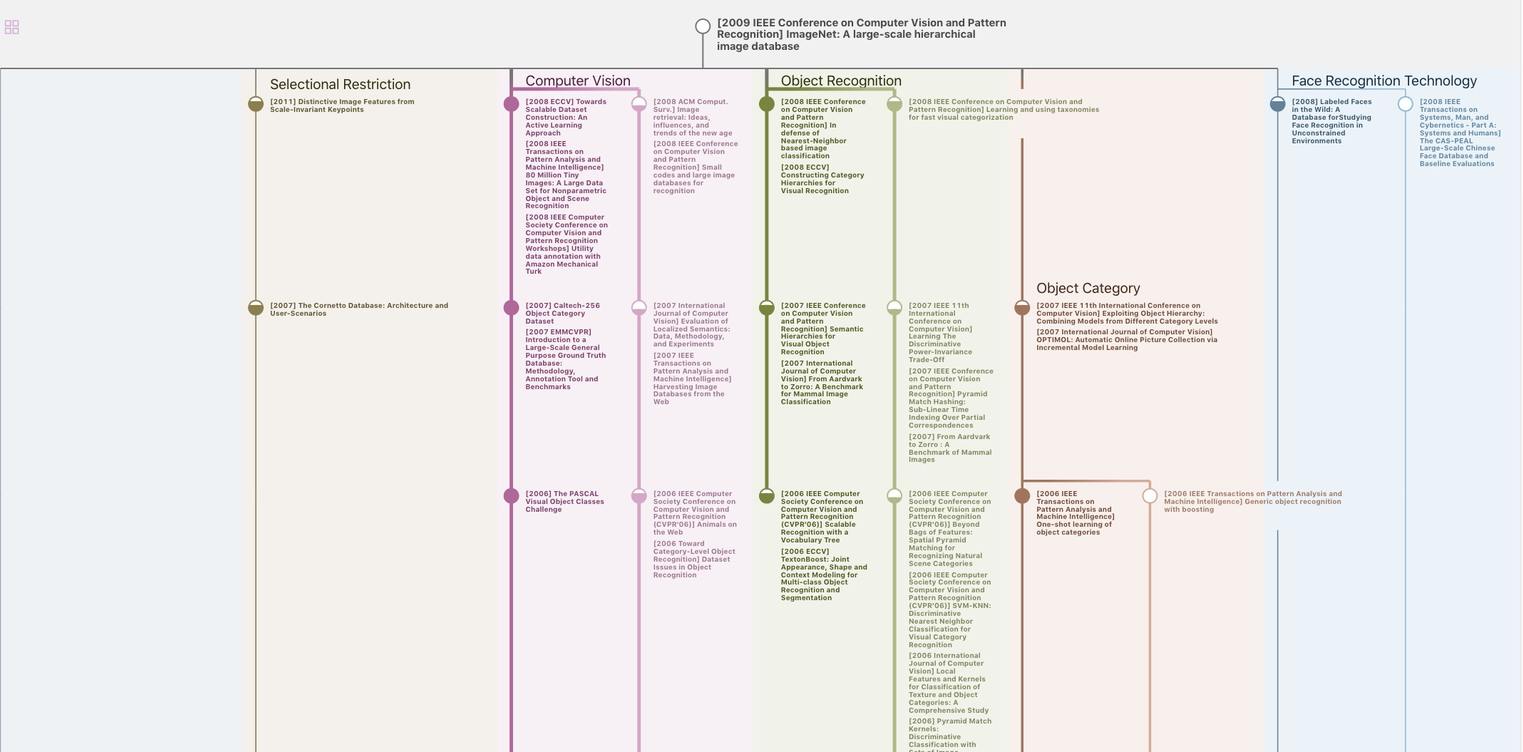
生成溯源树,研究论文发展脉络
Chat Paper
正在生成论文摘要