MPCCT: Multimodal vision-language learning paradigm with context-based compact Transformer
PATTERN RECOGNITION(2024)
摘要
Transformer and its variants have become the preferred option for multimodal vision-language paradigms. However, they struggle with tasks that demand high-dependency modeling and reasoning, like visual question answering (VQA) and visual grounding (VG). For this, we propose a general scheme called MPCCT, which: (1) incorporates designed textual global-context information to facilitate precise computation of dependency relationships between language tokens in the language encoder; (2) dynamically modulates and filters image features using optimized textual global-context information, combined with designed spatial context information, to further enhance the dependency modeling of image tokens and the model's reasoning ability; (3) reasonably align the language sequence containing textual global-context information with the image sequence information modulated by spatial position information. To validate MPCCT, we conducted extensive experiments on five benchmark datasets in VQA and VG, achieving new SOTA performance on multiple benchmarks, especially 73.71% on VQA-v2 and 99.15% on CLEVR. The code is available at https://github. com/RainyMoo/myvqa.
更多查看译文
关键词
Multimodal vision-language paradigms,High-dependency modeling,Visual question answering (VQA),Visual grounding (VG),Logical relationship reasoning
AI 理解论文
溯源树
样例
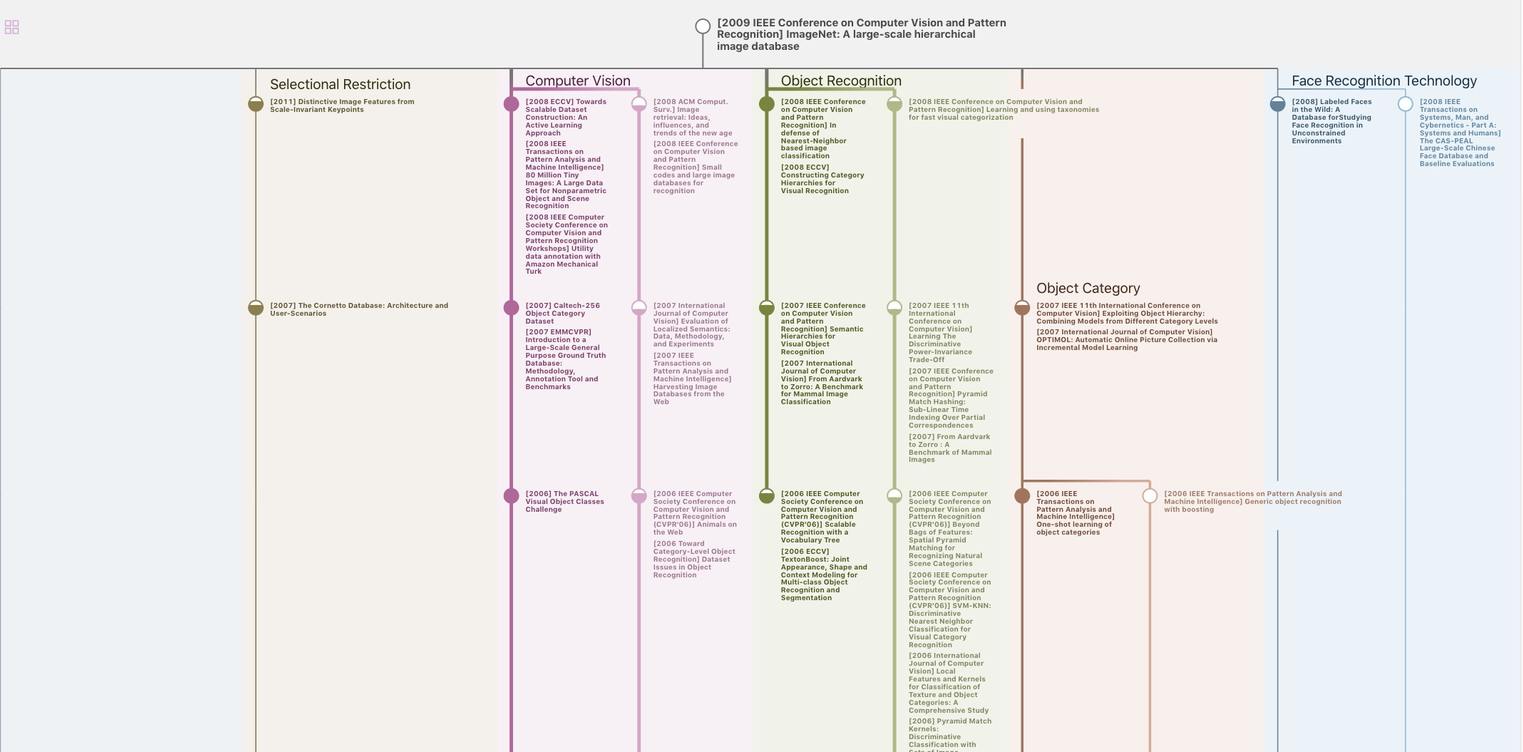
生成溯源树,研究论文发展脉络
Chat Paper
正在生成论文摘要