Leveraging machine learning to automate regression model evaluations for large multi-site water-quality trend studies
Environmental Modelling & Software(2023)
摘要
Large multi-site trend studies provide an opportunity to evaluate progress of waterbodies towards water-quality goals across broad geographic areas. Such studies often aggregate the results of site-specific models and thus contend with evaluating each model for appropriate fit and statistical assumptions. We explored the use of four traditional machine learning models (logistic regression, linear and quadratic discriminant analysis, and k-nearest neighbors) to perform these checks and estimate probabilities that an analyst would publish or reject a site-specific trend model from a multi-site study. We trained these “model-checking models” (MCMs) using a national study of over 6000 trend models and tested the MCMs using a smaller set of novel trend models. Although the MCMs did not perform well enough to bypass analyst review entirely, we found incorporating an MCM into a larger evaluation workflow can reduce the number of trend models needing an analyst review by more than half.
更多查看译文
关键词
Weighted regressions on time,discharge,and season (WRTDS),United States,Trend assessment,water-quality trends,machine learning
AI 理解论文
溯源树
样例
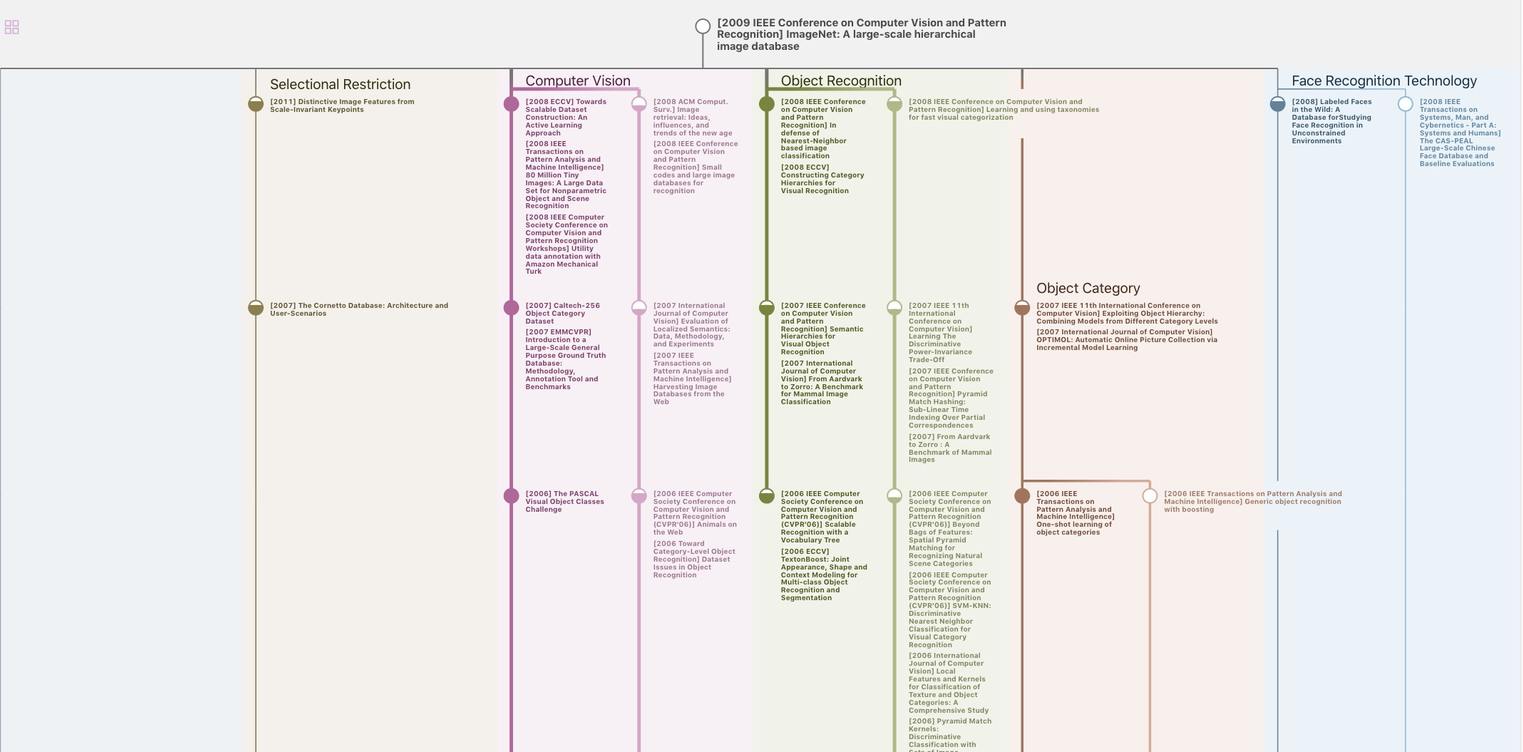
生成溯源树,研究论文发展脉络
Chat Paper
正在生成论文摘要