A decentralized federated learning-based spatial-temporal model for freight traffic speed forecasting
EXPERT SYSTEMS WITH APPLICATIONS(2024)
摘要
Accurately understanding the spatial-temporal information of future freight traffic speed in the metropolitan area is of vital importance to formulate freight-related traffic management strategies. In this study, we develop a novel decentralized federated learning-based spatial-temporal model for freight traffic speed forecasting while implementing collaborative training among multiple participants instead of requiring an exclusive cloud center server for centralized data processing. First, a tailored spatial-temporal transformer network is proposed to substitute the existing graph convolutional network for local personalized learning of each participant. Second, a decentralized federated learning model is designed to fuse local personalization models for freight traffic speed forecasting. The associated convergence properties are theoretically illustrated. rgb]0,0,0Finally, the experiments based on a real-world freight dataset of member cities in the Nanjing Metropolitan Area demonstrate that the proposed approach can accurately forecast freight traffic speed and outperform existing traffic speed forecasting methods, with the average improvement of 8.3% for MAE, 8.2% for RMSE, and 8.6% for MAPE respectively. The visualization results reveal that the proposed approach is able to effectively capture the internal spatial-temporal dependencies among urban regions from various neighboring cities, providing the insights for collaboratively developing proactive freight-related traffic management strategies.
更多查看译文
关键词
Freight traffic speed forecasting,Decentralized federated learning,Transformer network,Metropolitan area,Traffic management
AI 理解论文
溯源树
样例
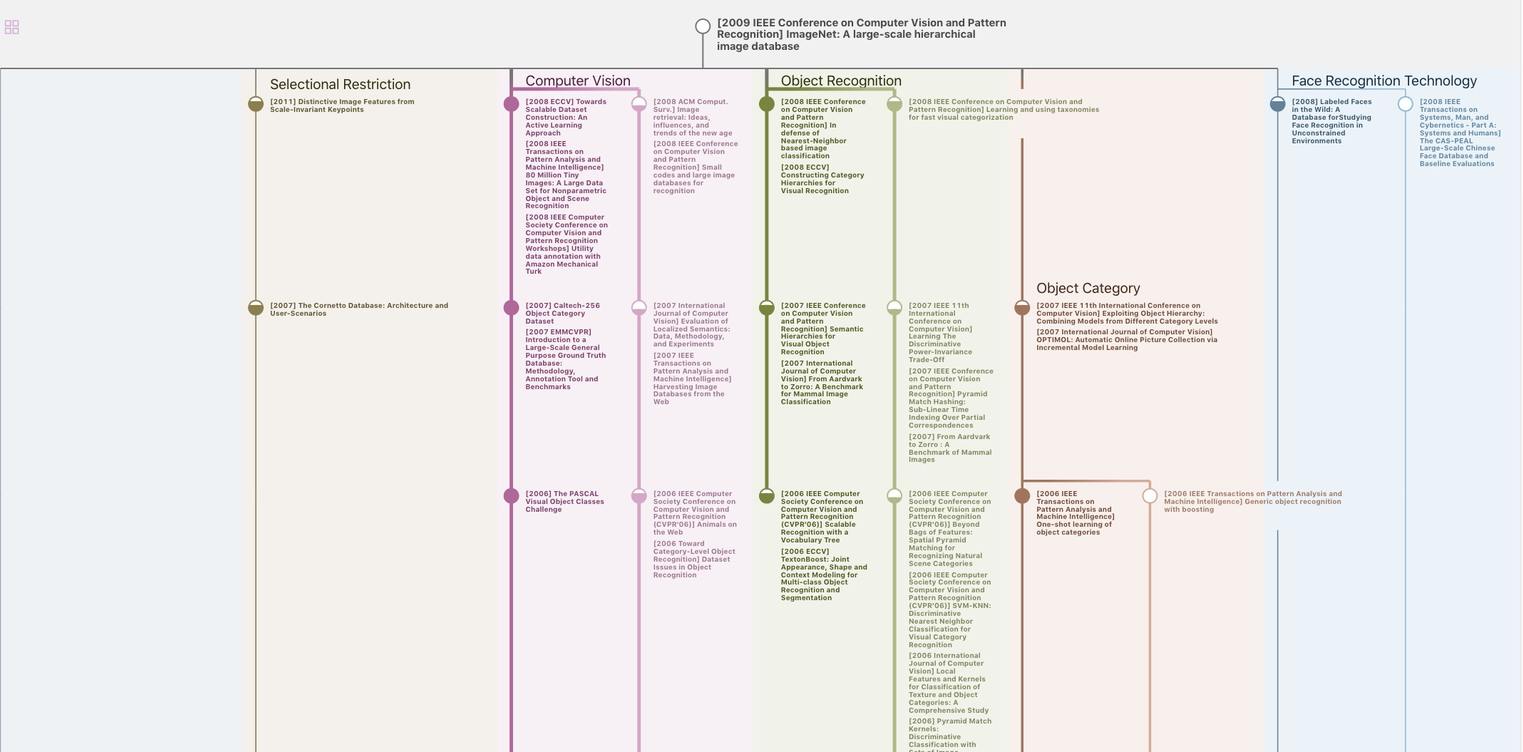
生成溯源树,研究论文发展脉络
Chat Paper
正在生成论文摘要