Developing a hybrid model for predicting the reaction kinetics between chlorine and micropollutants in water
WATER RESEARCH(2023)
摘要
Understanding the reactivities of chlorine towards micropollutants is crucial for assessing the fate of micropollutants in water chlorination. In this study, we integrated machine learning with kinetic modeling to predict the reaction kinetics between micropollutants and chlorine in deionized water and real surface water. We first established a framework to predict the apparent second-order rate constants for micropollutants with chlorine by combining Morgan molecular fingerprints with machine learning algorithms. The framework was tuned using Bayesian optimization and showed high prediction accuracy. It was validated through experiments and used to predict the unreported apparent second-order rate constants for 103 emerging micropollutants with chlorine. The framework also improved the understanding of the structure-dependence of micropollutants' reactivity with chlorine. We incorporated the predicted apparent second-order rate constants into the Kintecus software to establish a hybrid model to profile the time-dependent changes of micropollutant concentrations by chlorination. The hybrid model was validated by experiments conducted in real surface water in the presence of natural organic matter. The hybrid model could predict how much micropollutants were degraded by chlorination with varied chlorine contact times and/or initial chlorine dosages. This study advances fundamental understanding of the reaction kinetics between chlorine and emerging micropollutants, and also offers a valuable tool to assess the fate of micropollutants during chlorination of drinking water.
更多查看译文
AI 理解论文
溯源树
样例
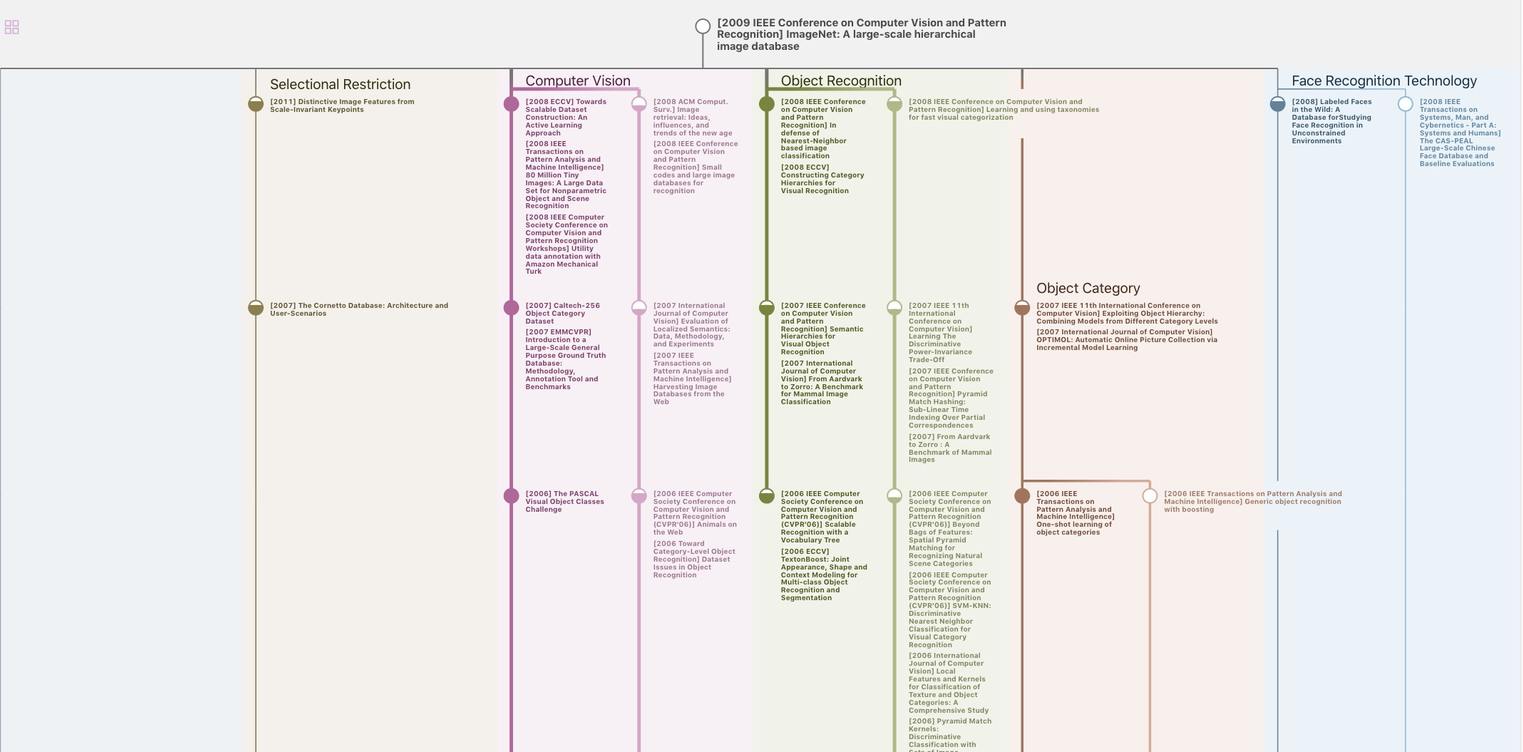
生成溯源树,研究论文发展脉络
Chat Paper
正在生成论文摘要