Measuring arrangement and size distributions of flowing droplets in microchannels through deep learning
arXiv (Cornell University)(2023)
摘要
In microfluidic systems, droplets undergo intricate deformations as they
traverse flow-focusing junctions, posing a challenging task for accurate
measurement, especially during short transit times. This study investigates the
physical behavior of droplets within dense emulsions in diverse microchannel
geometries, specifically focusing on the impact of varying opening angles
within the primary channel and injection rates of fluid components. Employing a
sophisticated droplet tracking tool based on deep-learning techniques, we
analyze multiple frames from flow-focusing experiments to quantitatively
characterize droplet deformation in terms of ratio between maximum width and
height and propensity to form liquid with hexagonal crystalline order. Our
findings reveal the existence of an optimal opening angle where shape
deformations are minimal and crystal-like arrangement is maximal. Variations of
fluid injection rates are also found to affect size and packing fraction of the
emulsion in the exit channel. This paper offers insights into deformations,
size and structure of fluid emulsions relative to microchannel geometry and
other flow-related parameters captured through machine learning, with potential
implications for the design of microchips utilized in cellular transport and
tissue engineering applications.
更多查看译文
关键词
droplets,microchannels,deep learning,size distributions
AI 理解论文
溯源树
样例
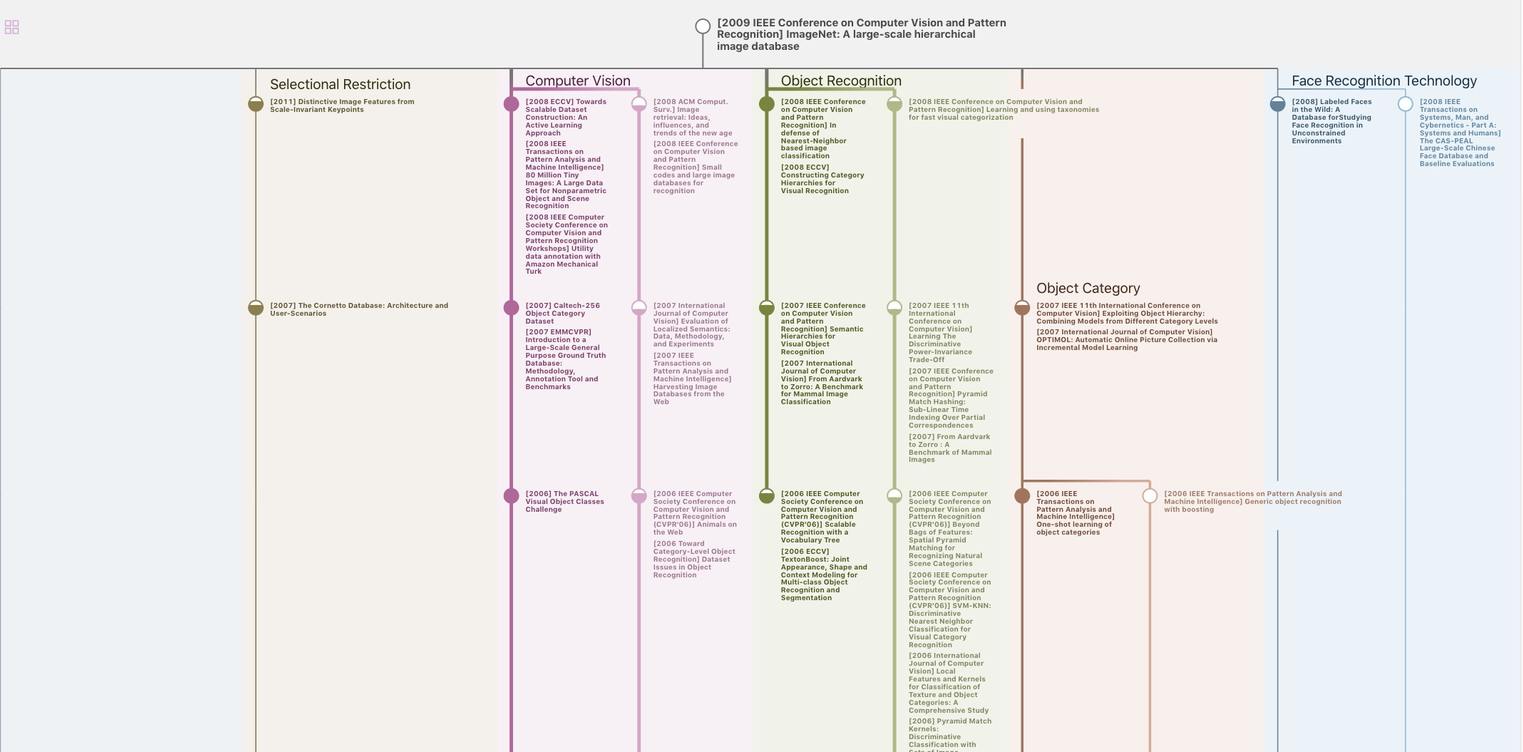
生成溯源树,研究论文发展脉络
Chat Paper
正在生成论文摘要