On the data-driven description of lattice materials mechanics
Results in Engineering(2024)
摘要
In the emerging field of mechanical metamaterials, using periodic lattice structures as a primary ingredient is relatively frequent. However, the choice of aperiodic lattices in these structures presents unique advantages regarding failure, e.g., buckling or fracture, because avoiding repeated patterns prevents global failures, with local failures occurring in turn that can beneficially delay structural collapse. Therefore, it is expedient to develop models for computing efficiently the effective mechanical properties in lattices from different general features while addressing the challenge of presenting topologies (or graphs) of different sizes. In this paper, we develop a deep learning model to predict energetically-equivalent mechanical properties of linear elastic lattices effectively. Considering the lattice as a graph and defining material and geometrical features on such, we show that Graph Neural Networks provide more accurate predictions than a dense, fully connected strategy, thanks to the geometrically induced bias through graph representation, closer to the underlying equilibrium laws from mechanics solved in the direct problem. Leveraging the efficient forward-evaluation of a vast number of lattices using this surrogate enables the inverse problem, i.e., to obtain a structure having prescribed specific behavior, which is ultimately suitable for multiscale structural optimization problems.
更多查看译文
关键词
Graph neural networks,lattice materials,mechanical metamaterials
AI 理解论文
溯源树
样例
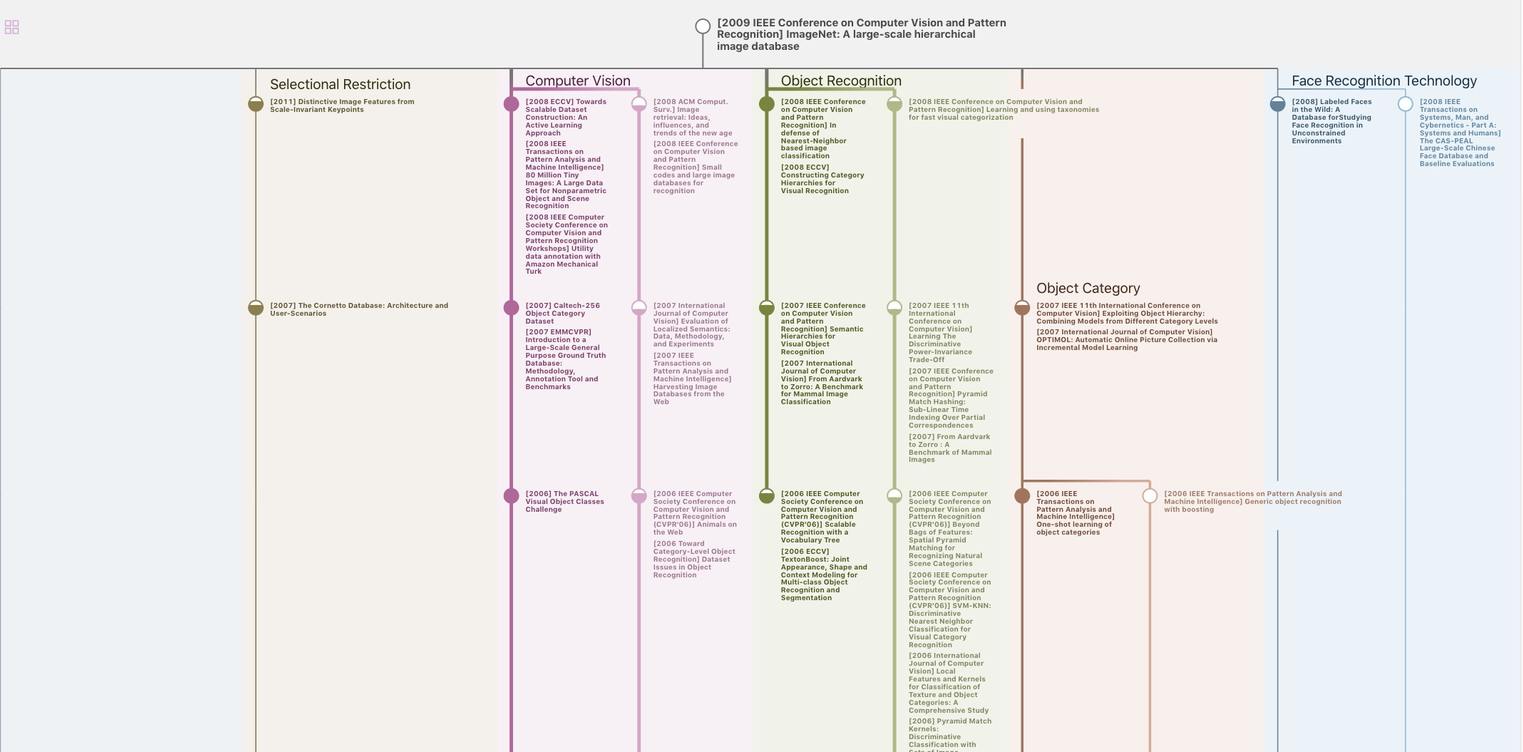
生成溯源树,研究论文发展脉络
Chat Paper
正在生成论文摘要