Conditional Unscented Autoencoders for Trajectory Prediction
CoRR(2023)
摘要
The \ac{CVAE} is one of the most widely-used models in trajectory prediction for \ac{AD}. It captures the interplay between a driving context and its ground-truth future into a probabilistic latent space and uses it to produce predictions. In this paper, we challenge key components of the CVAE. We leverage recent advances in the space of the VAE, the foundation of the CVAE, which show that a simple change in the sampling procedure can greatly benefit performance. We find that unscented sampling, which draws samples from any learned distribution in a deterministic manner, can naturally be better suited to trajectory prediction than potentially dangerous random sampling. We go further and offer additional improvements, including a more structured mixture latent space, as well as a novel, potentially more expressive way to do inference with CVAEs. We show wide applicability of our models by evaluating them on the INTERACTION prediction dataset, outperforming the state of the art, as well as at the task of image modeling on the CelebA dataset, outperforming the baseline vanilla CVAE. Code is available at https://github.com/boschresearch/cuae-prediction.
更多查看译文
关键词
conditional unscented autoencoders,trajectory prediction
AI 理解论文
溯源树
样例
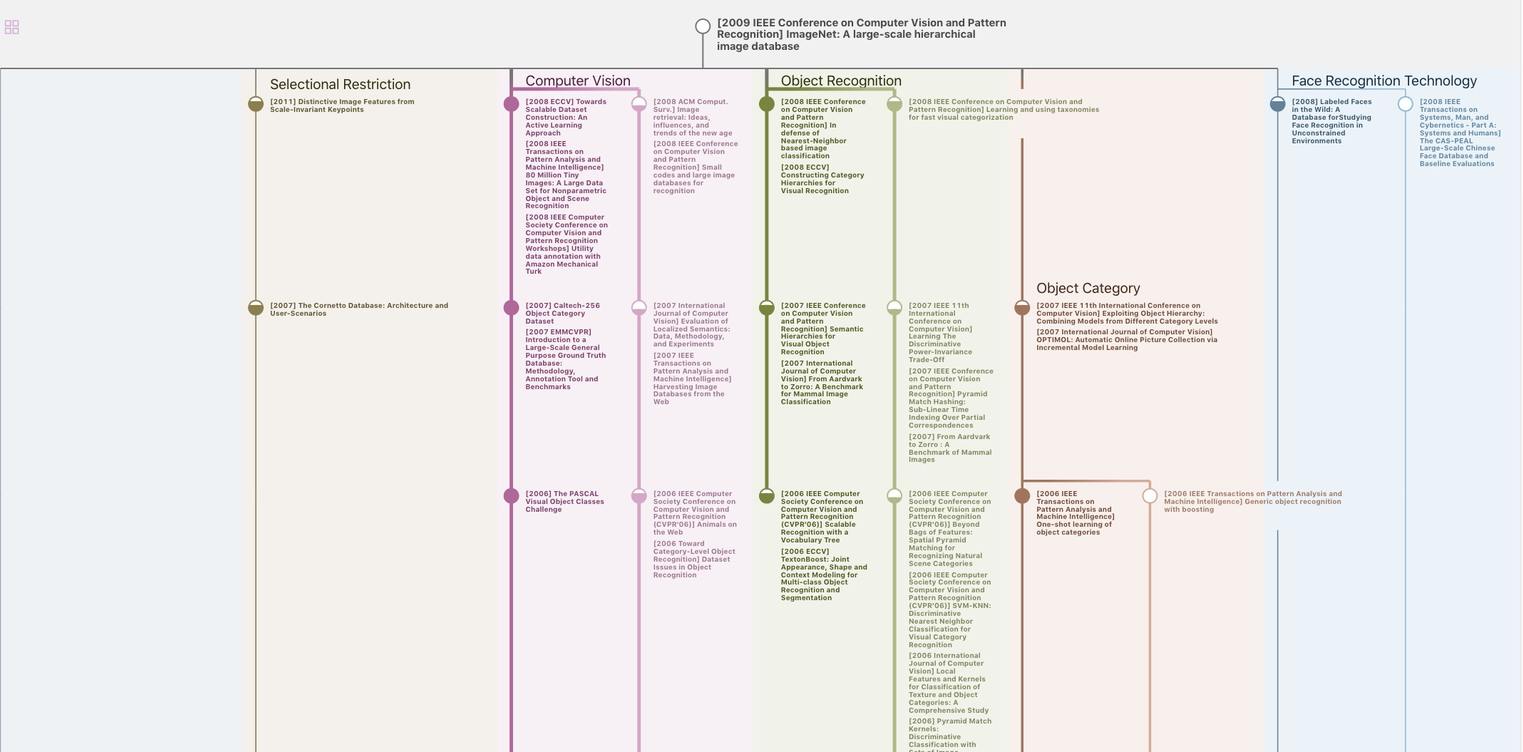
生成溯源树,研究论文发展脉络
Chat Paper
正在生成论文摘要