GG-LLM: Geometrically Grounding Large Language Models for Zero-shot Human Activity Forecasting in Human-Aware Task Planning
CoRR(2023)
摘要
A robot in a human-centric environment needs to account for the human's intent and future motion in its task and motion planning to ensure safe and effective operation. This requires symbolic reasoning about probable future actions and the ability to tie these actions to specific locations in the physical environment. While one can train behavioral models capable of predicting human motion from past activities, this approach requires large amounts of data to achieve acceptable long-horizon predictions. More importantly, the resulting models are constrained to specific data formats and modalities. Moreover, connecting predictions from such models to the environment at hand to ensure the applicability of these predictions is an unsolved problem. We present a system that utilizes a Large Language Model (LLM) to infer a human's next actions from a range of modalities without fine-tuning. A novel aspect of our system that is critical to robotics applications is that it links the predicted actions to specific locations in a semantic map of the environment. Our method leverages the fact that LLMs, trained on a vast corpus of text describing typical human behaviors, encode substantial world knowledge, including probable sequences of human actions and activities. We demonstrate how these localized activity predictions can be incorporated in a human-aware task planner for an assistive robot to reduce the occurrences of undesirable human-robot interactions by 29.2% on average.
更多查看译文
关键词
human activity forecasting,large language models,planning,language models,gg-llm,zero-shot,human-aware
AI 理解论文
溯源树
样例
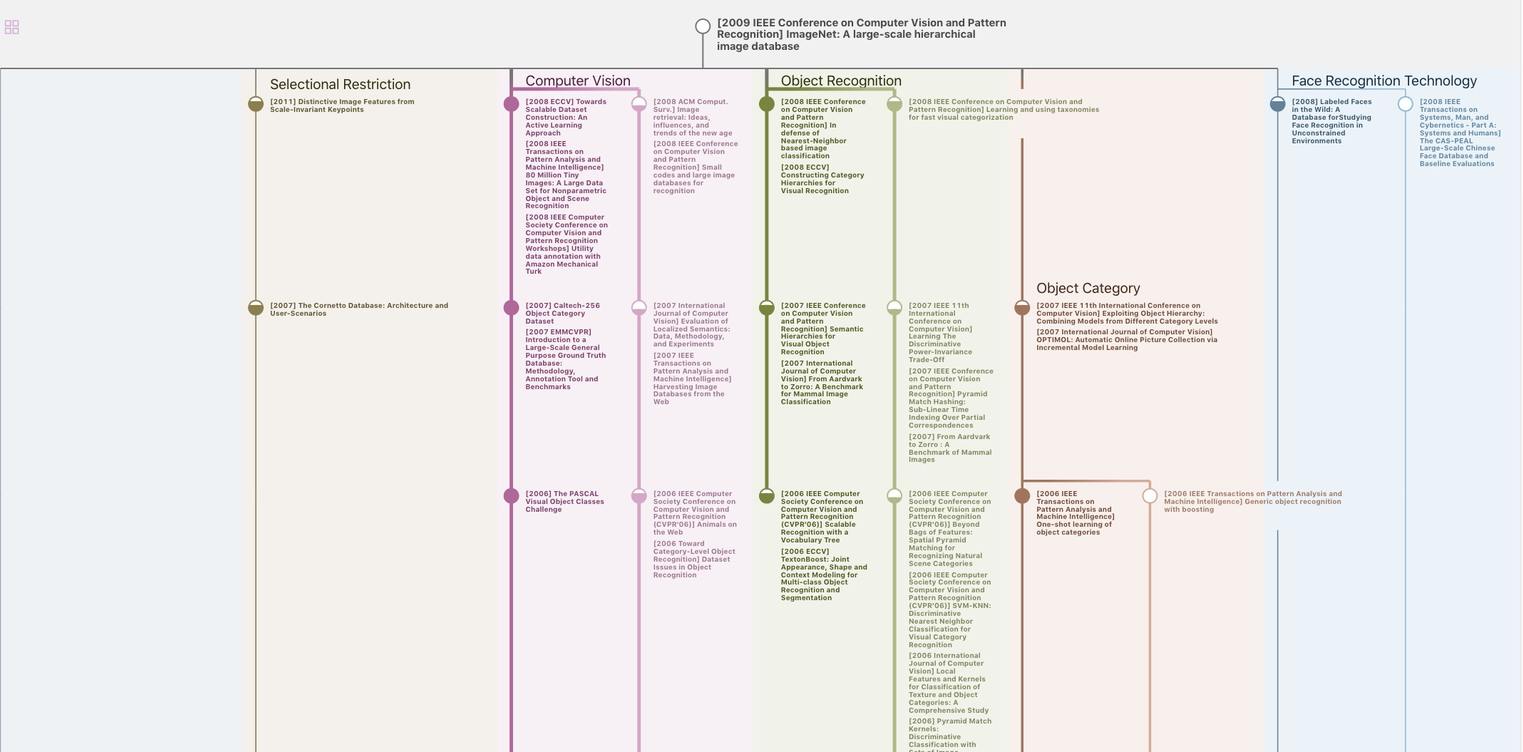
生成溯源树,研究论文发展脉络
Chat Paper
正在生成论文摘要