HEDNet: A Hierarchical Encoder-Decoder Network for 3D Object Detection in Point Clouds
arXiv (Cornell University)(2023)
摘要
3D object detection in point clouds is important for autonomous driving systems. A primary challenge in 3D object detection stems from the sparse distribution of points within the 3D scene. Existing high-performance methods typically employ 3D sparse convolutional neural networks with small kernels to extract features. To reduce computational costs, these methods resort to submanifold sparse convolutions, which prevent the information exchange among spatially disconnected features. Some recent approaches have attempted to address this problem by introducing large-kernel convolutions or self-attention mechanisms, but they either achieve limited accuracy improvements or incur excessive computational costs. We propose HEDNet, a hierarchical encoder-decoder network for 3D object detection, which leverages encoder-decoder blocks to capture long-range dependencies among features in the spatial space, particularly for large and distant objects. We conducted extensive experiments on the Waymo Open and nuScenes datasets. HEDNet achieved superior detection accuracy on both datasets than previous state-of-the-art methods with competitive efficiency. The code is available at https://github.com/zhanggang001/HEDNet.
更多查看译文
关键词
3d object detection
AI 理解论文
溯源树
样例
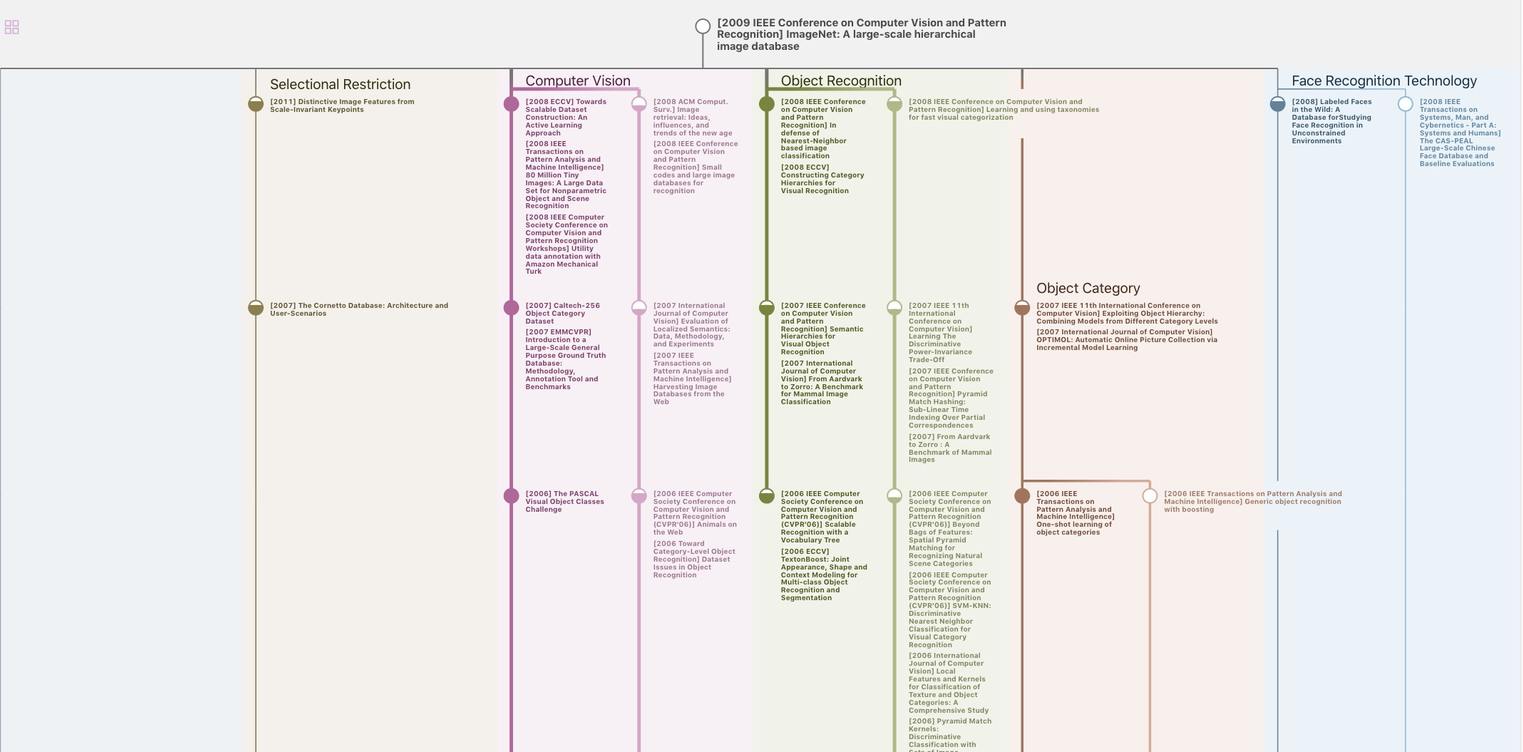
生成溯源树,研究论文发展脉络
Chat Paper
正在生成论文摘要