CAFE: Conflict-Aware Feature-wise Explanations
CoRR(2023)
摘要
Feature attribution methods are widely used to explain neural models by determining the influence of individual input features on the models' outputs. We propose a novel feature attribution method, CAFE (Conflict-Aware Feature-wise Explanations), that addresses three limitations of the existing methods: their disregard for the impact of conflicting features, their lack of consideration for the influence of bias terms, and an overly high sensitivity to local variations in the underpinning activation functions. Unlike other methods, CAFE provides safeguards against overestimating the effects of neuron inputs and separately traces positive and negative influences of input features and biases, resulting in enhanced robustness and increased ability to surface feature conflicts. We show experimentally that CAFE is better able to identify conflicting features on synthetic tabular data and exhibits the best overall fidelity on several real-world tabular datasets, while being highly computationally efficient.
更多查看译文
关键词
conflict-aware,feature-wise
AI 理解论文
溯源树
样例
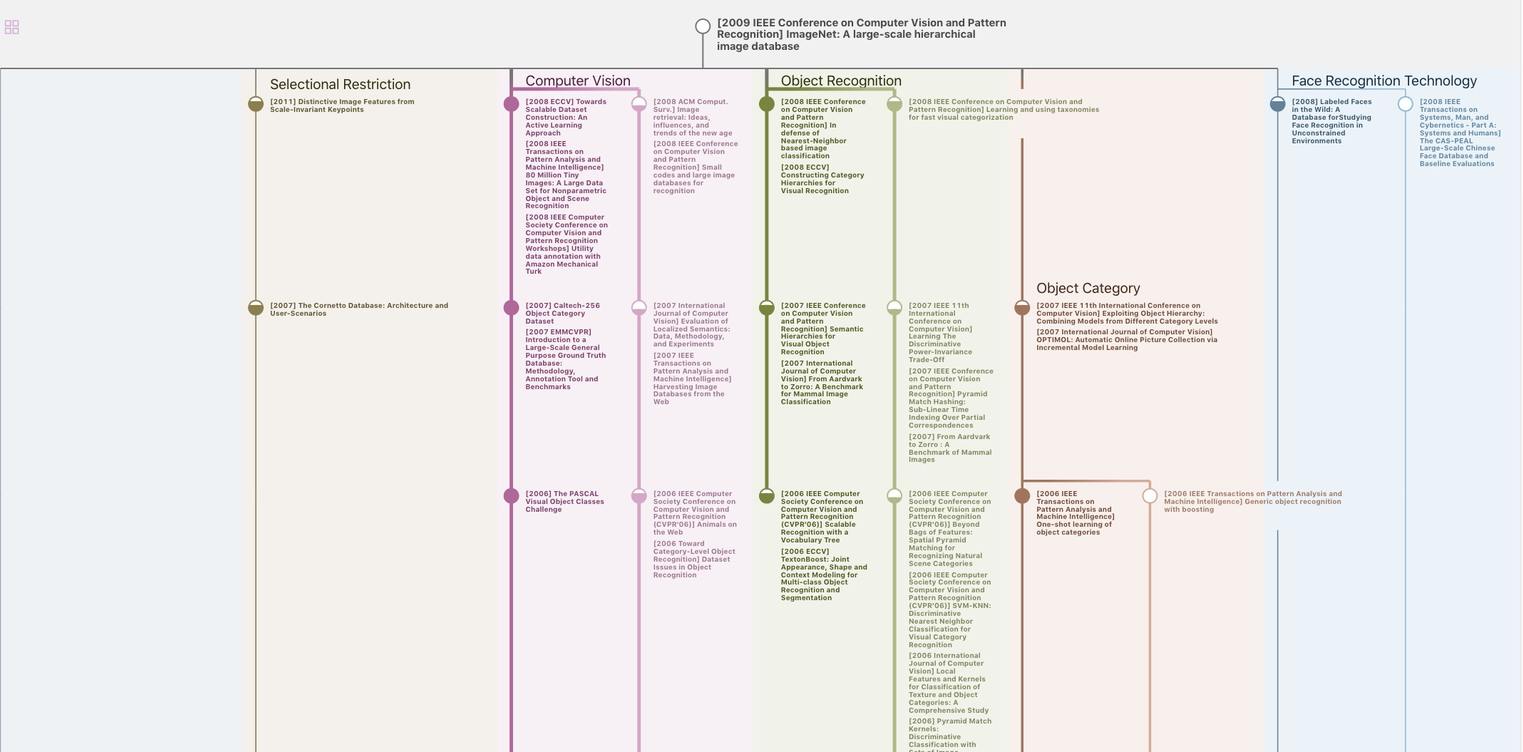
生成溯源树,研究论文发展脉络
Chat Paper
正在生成论文摘要