Collaborative Decision-Making Using Spatiotemporal Graphs in Connected Autonomy
ICRA 2024(2024)
摘要
Collaborative decision-making is an essential capability for multi-robot systems, such as connected vehicles, to collaboratively control autonomous vehicles in accident-prone scenarios. Under limited communication bandwidth, capturing comprehensive situational awareness by integrating connected agents' observation is very challenging. In this paper, we propose a novel collaborative decision-making method that efficiently and effectively integrates collaborators' representations to control the ego vehicle in accident-prone scenarios. Our approach formulates collaborative decision-making as a classification problem. We first represent sequences of raw observations as spatiotemporal graphs, which significantly reduce the package size to share among connected vehicles. Then we design a novel spatiotemporal graph neural network based on heterogeneous graph learning, which analyzes spatial and temporal connections of objects in a unified way for collaborative decision-making. We evaluate our approach using a high-fidelity simulator that considers realistic traffic, communication bandwidth, and vehicle sensing among connected autonomous vehicles. The experimental results show that our representation achieves over 100x reduction in the shared data size that meets the requirements of communication bandwidth for connected autonomous driving. In addition, our approach achieves over 30% improvements in driving safety.
更多查看译文
关键词
RGB-D Perception,Multi-Robot Systems
AI 理解论文
溯源树
样例
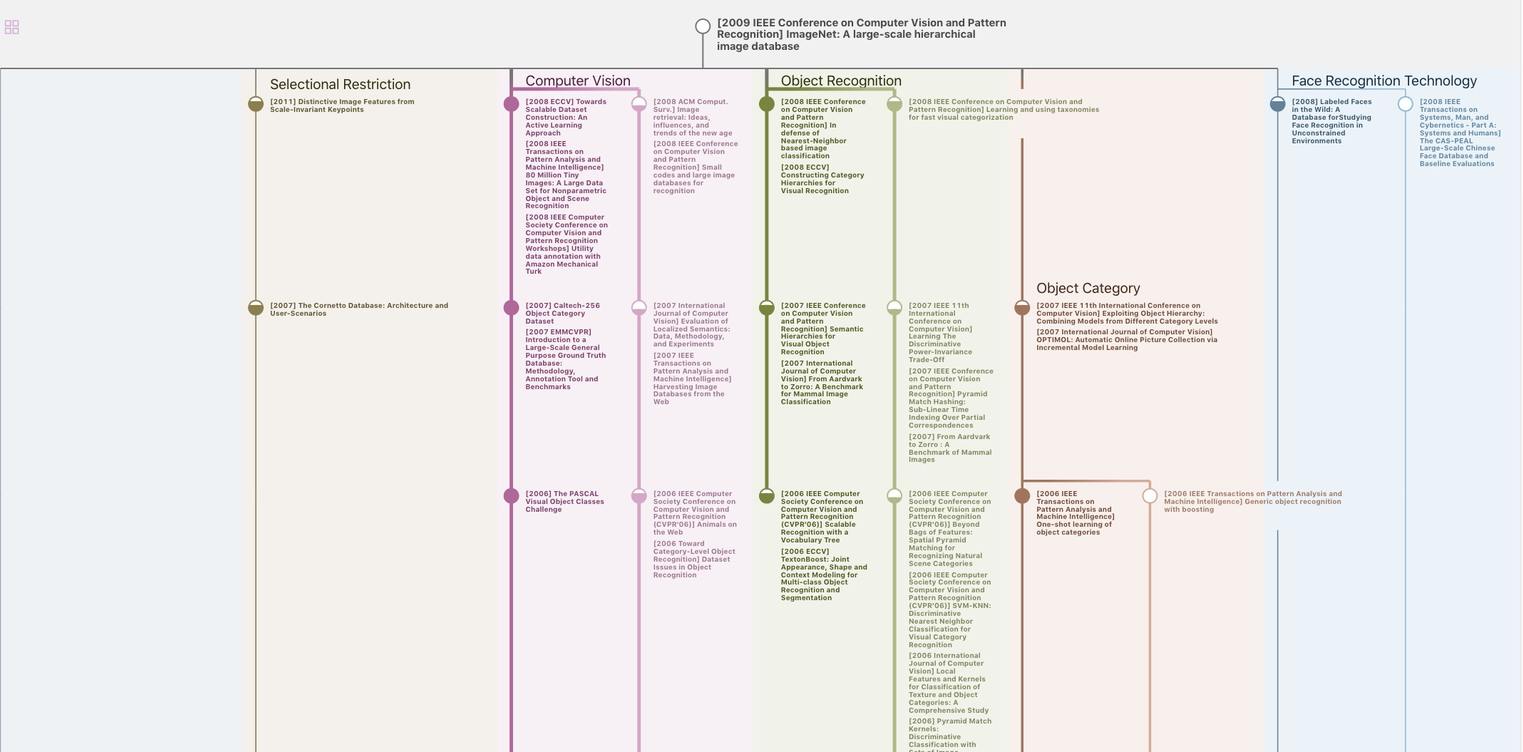
生成溯源树,研究论文发展脉络
Chat Paper
正在生成论文摘要