Hybridizing Physics and Neural ODEs for Predicting Plasma Inductance Dynamics in Tokamak Fusion Reactors
CoRR(2023)
摘要
While fusion reactors known as tokamaks hold promise as a firm energy source, advances in plasma control, and handling of events where control of plasmas is lost, are needed for them to be economical. A significant bottleneck towards applying more advanced control algorithms is the need for better plasma simulation, where both physics-based and data-driven approaches currently fall short. The former is bottle-necked by both computational cost and the difficulty of modelling plasmas, and the latter is bottle-necked by the relative paucity of data. To address this issue, this work applies the neural ordinary differential equations (ODE) framework to the problem of predicting a subset of plasma dynamics, namely the coupled plasma current and internal inductance dynamics. As the neural ODE framework allows for the natural inclusion of physics-based inductive biases, we train both physics-based and neural network models on data from the Alcator C-Mod fusion reactor and find that a model that combines physics-based equations with a neural ODE performs better than both existing physics-motivated ODEs and a pure neural ODE model.
更多查看译文
关键词
tokamak fusion reactors,neural odes,plasma inductance
AI 理解论文
溯源树
样例
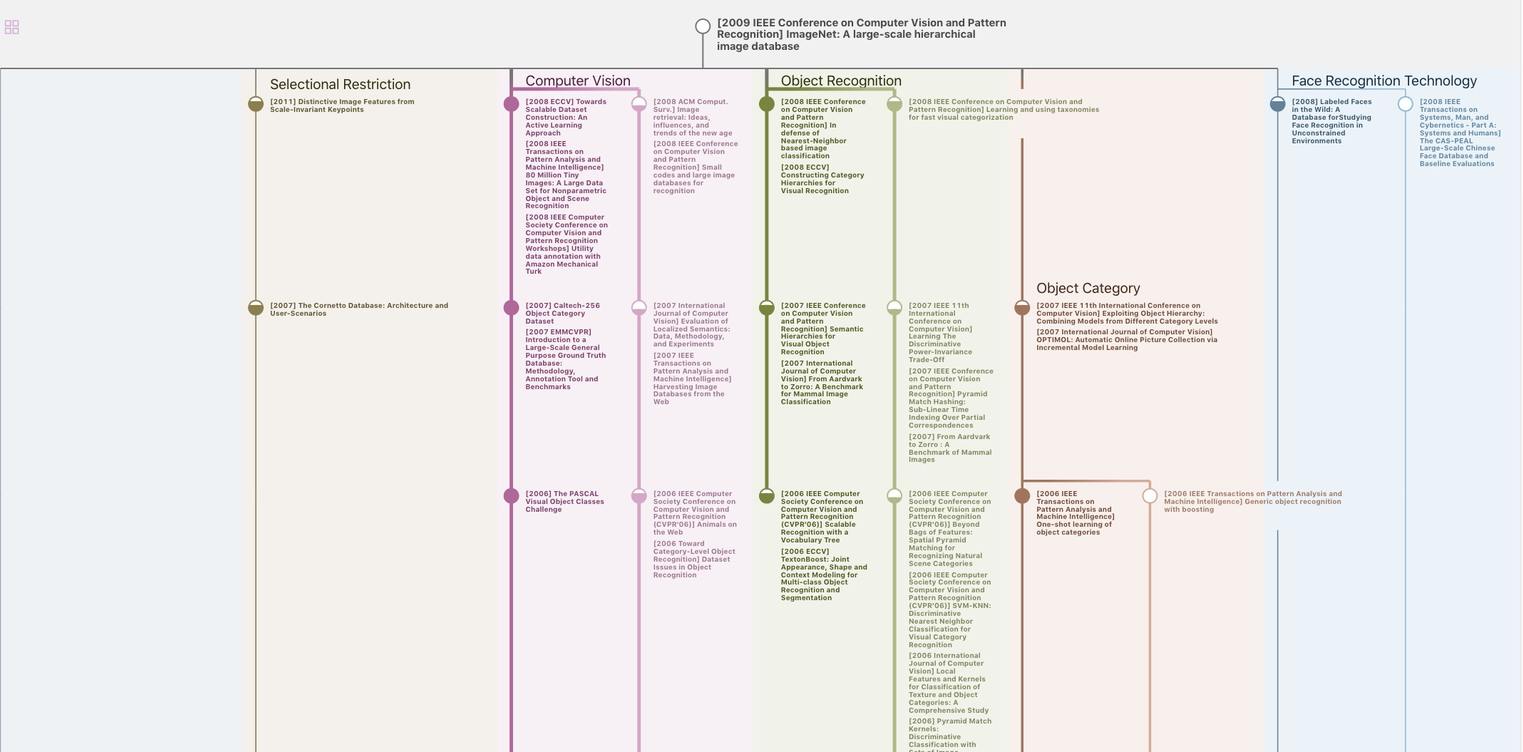
生成溯源树,研究论文发展脉络
Chat Paper
正在生成论文摘要