Assessing and Enhancing Robustness of Deep Learning Models with Corruption Emulation in Digital Pathology
2023 IEEE International Conference on Bioinformatics and Biomedicine (BIBM)(2023)
摘要
Deep learning in digital pathology brings intelligence and automation as substantial enhancements to pathological analysis, the gold standard of clinical diagnosis. However, multiple steps from tissue preparation to slide imaging introduce various image corruptions, making it difficult for deep neural network (DNN) models to achieve stable diagnostic results for clinical use. In order to assess and further enhance the robustness of the models, we analyze the physical causes of the full-stack corruptions throughout the pathological life-cycle and propose an Omni-Corruption Emulation (OmniCE) method to reproduce 21 types of corruptions quantified with 5-level severity. We then construct three OmniCE-corrupted benchmark datasets at both patch level and slide level and assess the robustness of popular DNNs in classification and segmentation tasks. Further, we explore to use the OmniCE-corrupted datasets as augmentation data for training and experiments to verify that the generalization ability of the models has been significantly enhanced.
更多查看译文
关键词
Digital Pathology,Deep Learning,Representation Learning
AI 理解论文
溯源树
样例
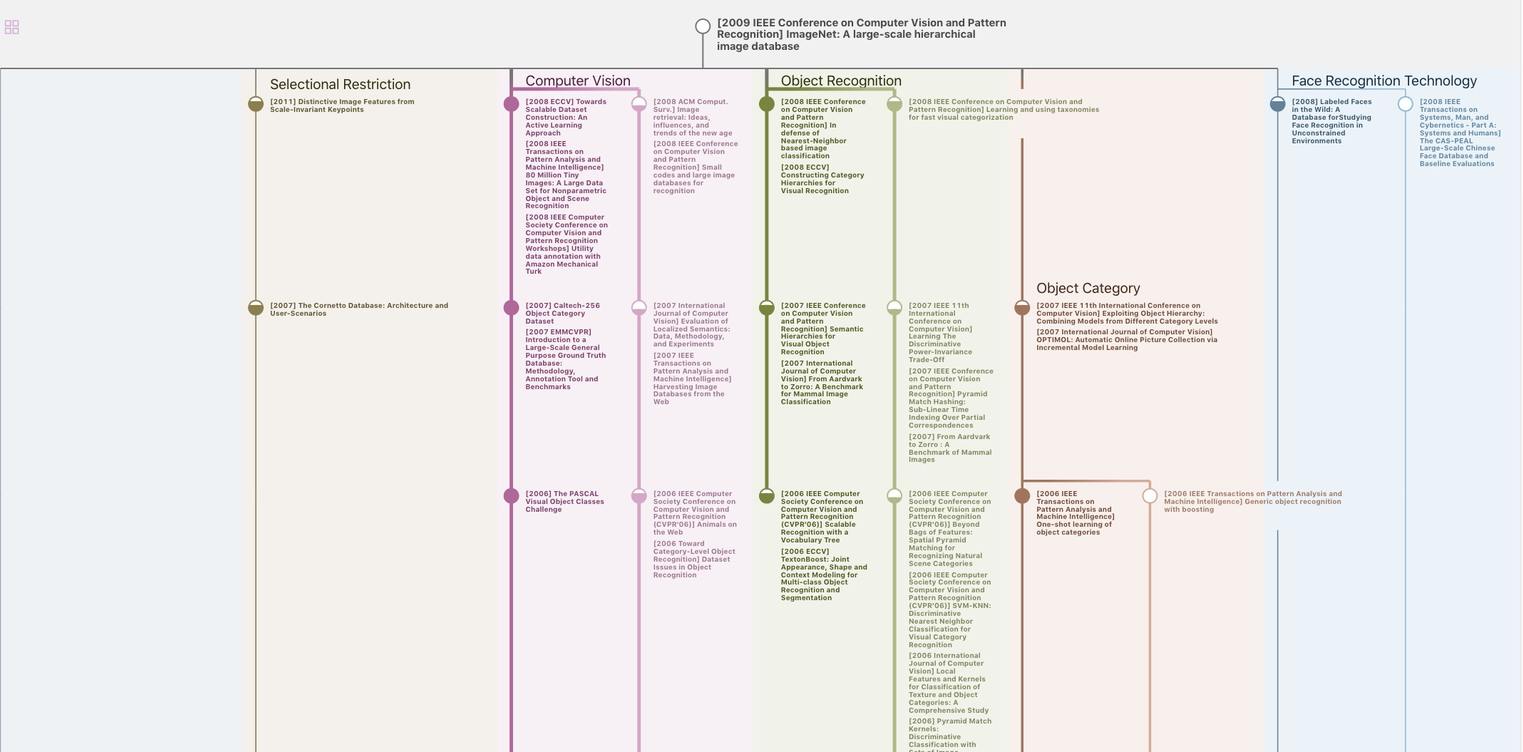
生成溯源树,研究论文发展脉络
Chat Paper
正在生成论文摘要