SPAR: An efficient self-attention network using Switching Partition Strategy for skeleton-based action recognition
NEUROCOMPUTING(2023)
摘要
Graph convolutional networks (GCN) have become the mainstream in skeleton-based action recognition. For further performance improvement, existing methods propose to utilize self-attention to model long-range features of joints. However, these methods cannot balance accuracy with computational efficiency. In this paper, we propose the Switching Partition Strategy (SPAR) Network that uses the self-attention mechanism for the simultaneous and efficient extraction of spatial-temporal long-range information from the skeleton. We design two partition strategies that reduce the computational cost and improve the efficiency of the computation of self-attention. Extensive experiments are conducted on two large-scale datasets, i.e. NTU RGB+D 60 and NTU RGB+D 120, to evaluate the performance of the proposed SPAR network. The results demonstrate that our method outperforms the state-of-the-art on accuracy as well as computational cost.
更多查看译文
关键词
Action recognition,Self-attention,3D-skeleton,Graph convolutional networks
AI 理解论文
溯源树
样例
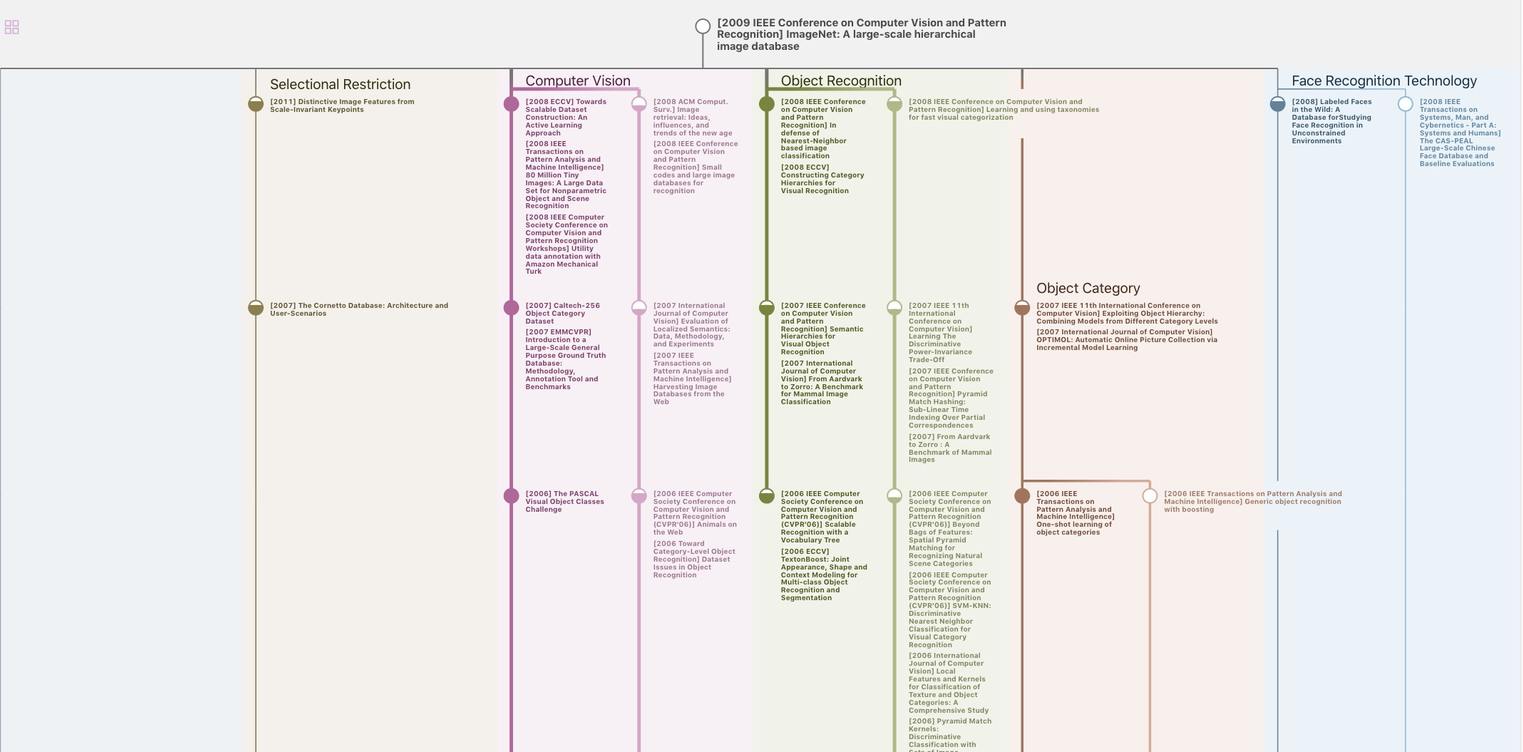
生成溯源树,研究论文发展脉络
Chat Paper
正在生成论文摘要