Deep learning based MLC aperture and monitor unit prediction as a warm start for breast VMAT optimisation
PHYSICS IN MEDICINE AND BIOLOGY(2023)
摘要
Objective. Automated treatment planning today is focussed on non-exact, two-step procedures. Firstly, dose-volume histograms (DVHs) or 3D dose distributions are predicted from the patient anatomy. Secondly, these are converted in multi-leaf collimator (MLC) apertures and monitor units (MUs) using a generic optimisation to obtain the final treatment plan. In contrast, we present a method to predict volumetric modulated arc therapy (VMAT) MLC apertures and MUs directly from patient anatomy using deep learning. The predicted plan is then provided as initialisation to the optimiser for fine-tuning. Approach. 148 patients (training: 101; validation: 23; test: 24), treated for right breast cancer, are replanned to obtain a homogeneous database of 3-arc VMAT plans (PTVBreast: 45.57 Gy; PTVBoost: 55.86 Gy) according to the clinical protocol, using RapidPlanTM with automatic optimisation and extended convergence mode (clinical workflow). Projections of the CT and contours are created along the beam's eye view of all control points and given as input to a U-net type convolutional neural networks (CNN). The output are the MLC aperture and MU for all control points, from which a DICOM RTplan is built. This is imported and further optimised in the treatment planning system using automatic optimisation without convergence mode, with clinical PTV objectives and organs-at-risk (OAR) objectives based on the DVHs calculated from the imported plan (CNN workflow). Main results. Mean dose differences between the clinical and CNN workflow over the test set are 0.2 +/- 0.5 Gy at D 95% and 0.6 +/- 0.4 Gy at D 0.035cc of PTVBreast and -0.4 +/- 0.3 Gy at D 95% and 0.7 +/- 0.3 Gy at D 0.035cc of PTVBoost. For the OAR, they are -0.2 +/- 0.2 Gy for D mean,heart and 0.04 +/- 0.8 Gy for D mean,ipsilateral lung. The mean computation time is 60 and 25 min respectively. Significance. VMAT optimisation can be initialised by MLC apertures and MUs, directly predicted from patient anatomy using a CNN, reducing planning time with more than half while maintaining clinically acceptable plans. This procedure puts the planner in a supervising role over an AI-based treatment planning workflow.
更多查看译文
关键词
radiotherapy,deep learning,VMAT,breast,automated treatment planning
AI 理解论文
溯源树
样例
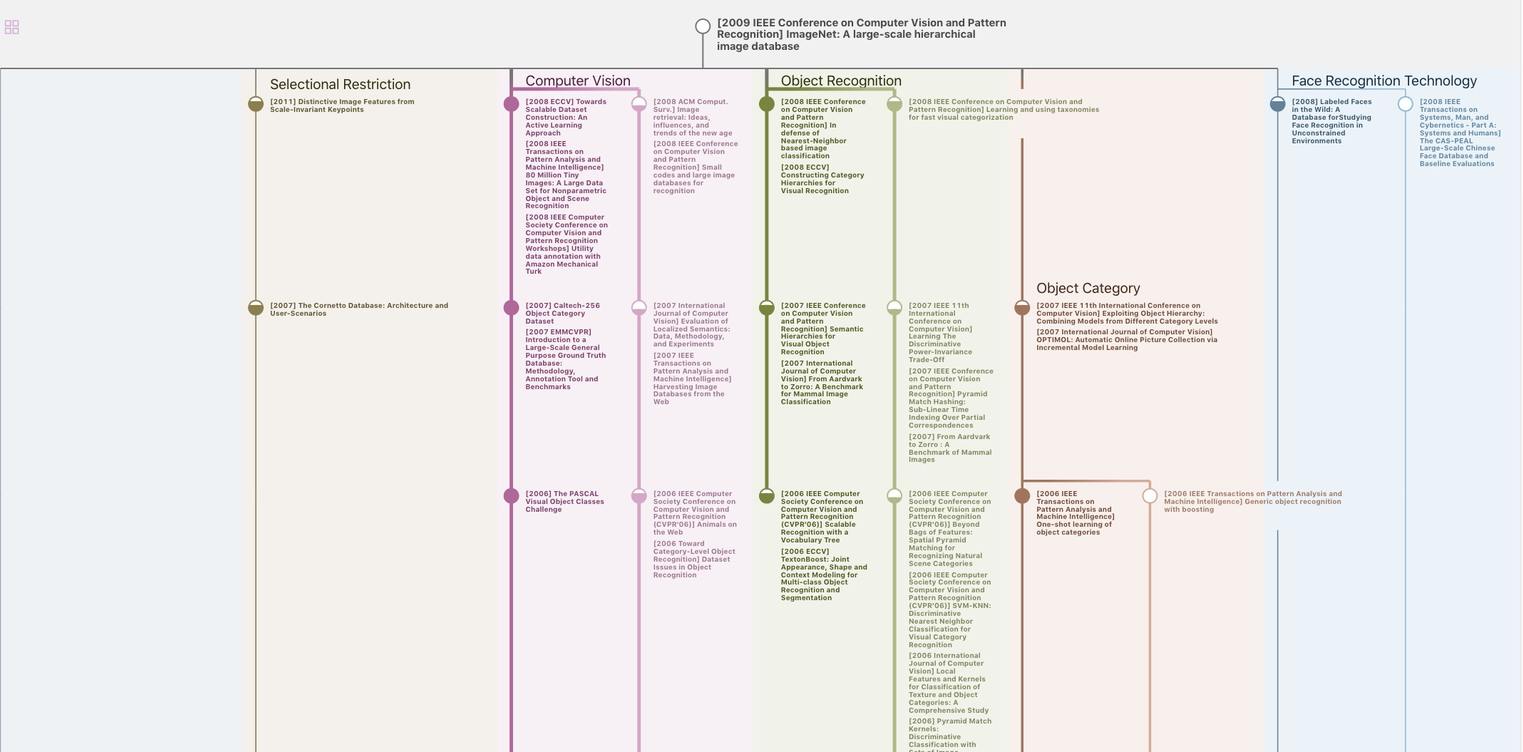
生成溯源树,研究论文发展脉络
Chat Paper
正在生成论文摘要