Enhancing Motor Imagery Decoding in Brain Computer Interfaces using Riemann Tangent Space Mapping and Cross Frequency Coupling
CoRR(2023)
摘要
Objective: Motor Imagery (MI) serves as a crucial experimental paradigm
within the realm of Brain Computer Interfaces (BCIs), aiming to decoding motor
intentions from electroencephalogram (EEG) signals. Method: Drawing inspiration
from Riemannian geometry and Cross-Frequency Coupling (CFC), this paper
introduces a novel approach termed Riemann Tangent Space Mapping using
Dichotomous Filter Bank with Convolutional Neural Network (DFBRTS) to enhance
the representation quality and decoding capability pertaining to MI features.
DFBRTS first initiates the process by meticulously filtering EEG signals
through a Dichotomous Filter Bank, structured in the fashion of a complete
binary tree. Subsequently, it employs Riemann Tangent Space Mapping to extract
salient EEG signal features within each sub-band. Finally, a lightweight
convolutional neural network is employed for further feature extraction and
classification, operating under the joint supervision of cross-entropy and
center loss. To validate the efficacy, extensive experiments were conducted
using DFBRTS on two well-established benchmark datasets: the BCI competition IV
2a (BCIC-IV-2a) dataset and the OpenBMI dataset. The performance of DFBRTS was
benchmarked against several state-of-the-art MI decoding methods, alongside
other Riemannian geometry-based MI decoding approaches. Results: DFBRTS
significantly outperforms other MI decoding algorithms on both datasets,
achieving a remarkable classification accuracy of 78.16% for four-class and
71.58% for two-class hold-out classification, as compared to the existing
benchmarks.
更多查看译文
关键词
motor imagery decoding,brain computer interfaces,riemann tangent space mapping,cross frequency coupling
AI 理解论文
溯源树
样例
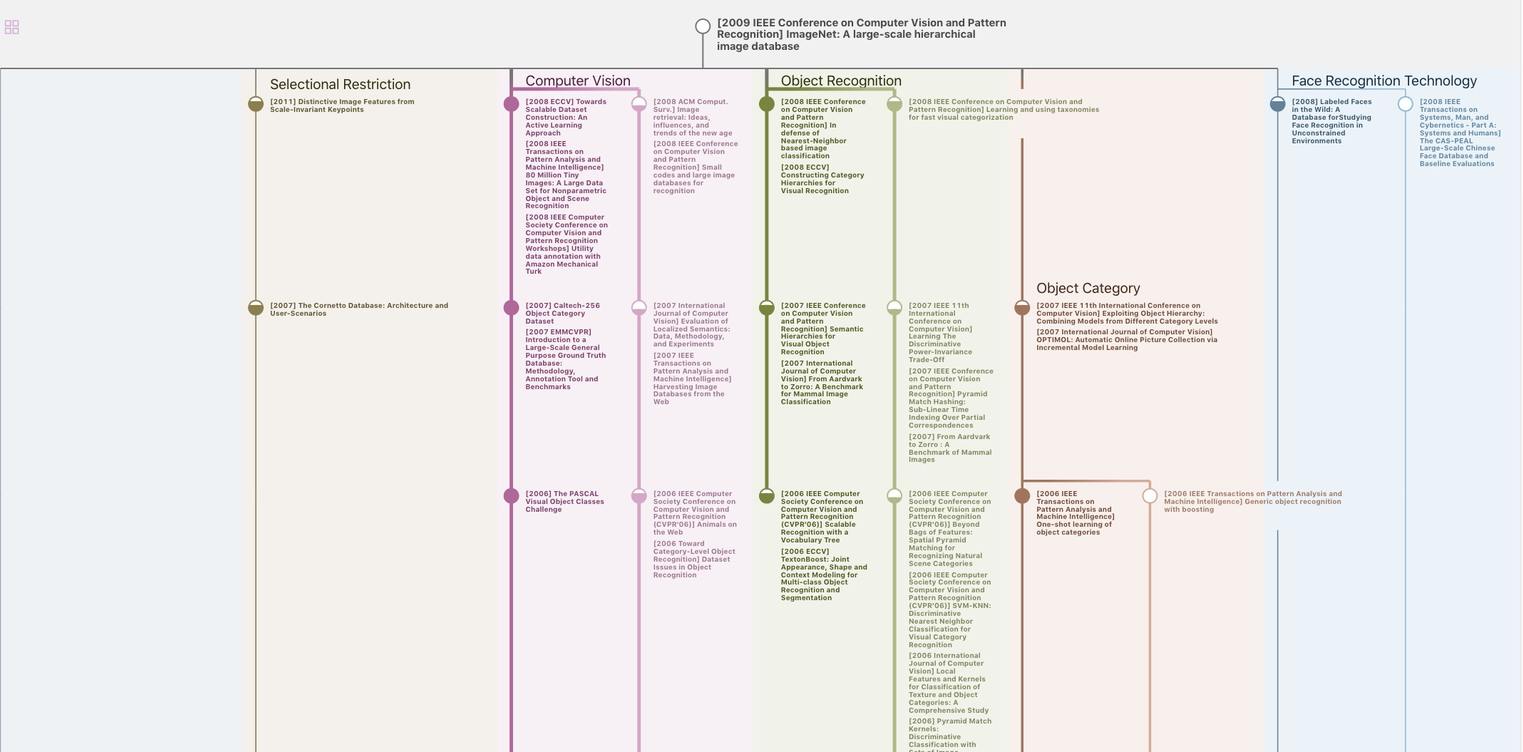
生成溯源树,研究论文发展脉络
Chat Paper
正在生成论文摘要