Quantile Super Learning for independent and online settings with application to solar power forecasting
arXiv (Cornell University)(2023)
摘要
Estimating quantiles of an outcome conditional on covariates is of
fundamental interest in statistics with broad application in probabilistic
prediction and forecasting. We propose an ensemble method for conditional
quantile estimation, Quantile Super Learning, that combines predictions from
multiple candidate algorithms based on their empirical performance measured
with respect to a cross-validated empirical risk of the quantile loss function.
We present theoretical guarantees for both iid and online data scenarios. The
performance of our approach for quantile estimation and in forming prediction
intervals is tested in simulation studies. Two case studies related to solar
energy are used to illustrate Quantile Super Learning: in an iid setting, we
predict the physical properties of perovskite materials for photovoltaic cells,
and in an online setting we forecast ground solar irradiance based on output
from dynamic weather ensemble models.
更多查看译文
关键词
quantile super learning,forecasting,solar
AI 理解论文
溯源树
样例
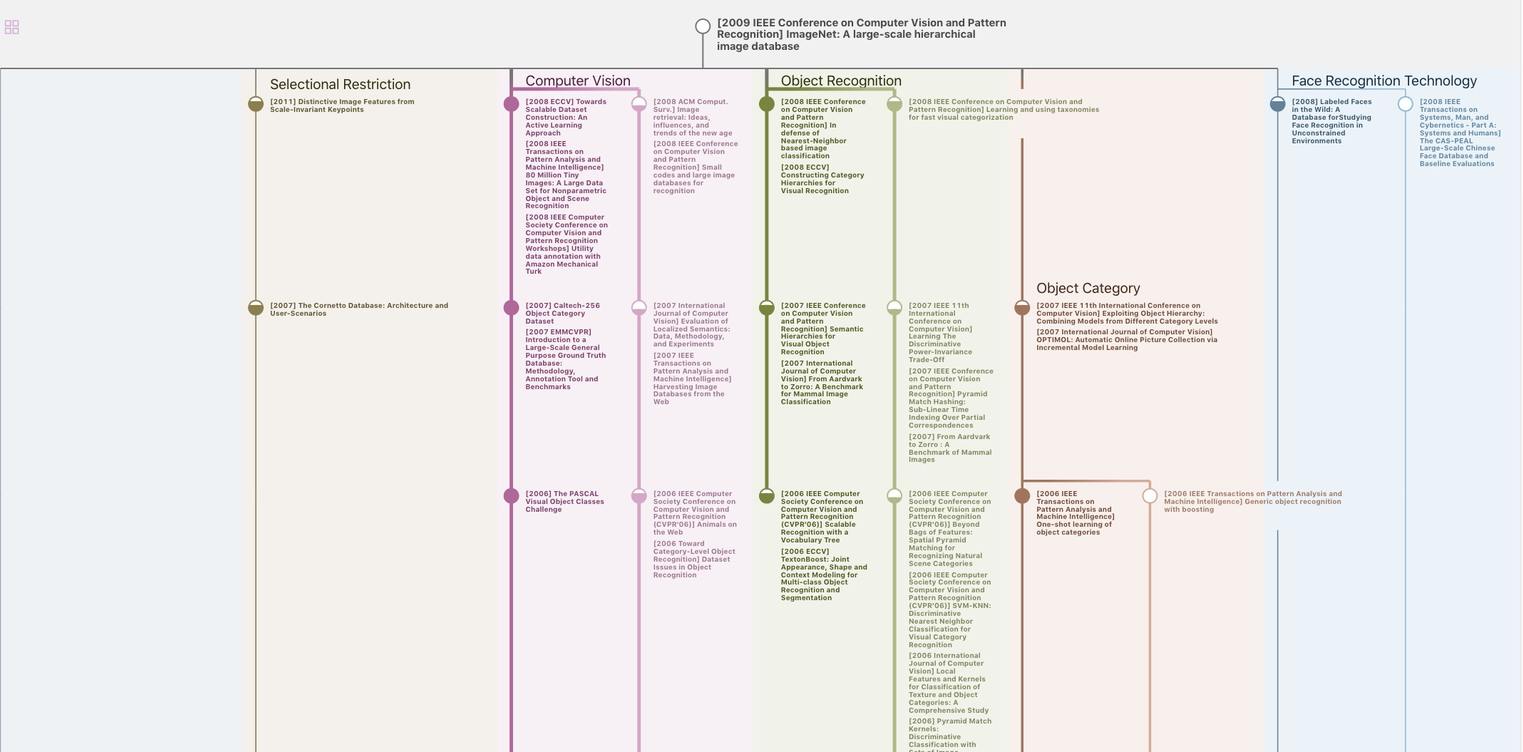
生成溯源树,研究论文发展脉络
Chat Paper
正在生成论文摘要