Measures of uncertainty for partially labeled categorical data based on an indiscernibility relation: an application in semi-supervised attribute reduction
APPLIED INTELLIGENCE(2023)
摘要
In many practical applications of machine learning, only part of data is labeled because the cost of assessing class label is relatively high. This paper concentrates on measures of uncertainty for a partial label categorical decision information system (p-CDIS), and considers an application to semi-supervised attribute reduction. Firstly, two decision information systems (DISs) can be induced by a p-CDIS ( U , C , d ): one is for a decision information system for labeled categorical data (U^l,C,d) and the other one is a decision information system for unlabeled categorical data (U^u,C,d) , and the missing rate of labels in ( U , C , d ) is introduced. In view of partial label data, the existential research did not take into account the missing rate of labels and only considered one importance of each attribute subset. Then, four importance of an attribute subset P⊆ C in ( U , C , d ) are defined based on an indiscernibility relation. They are the weighted sum of the importance of P in (U^l,C,d) and (U^u,C,d) determined by the missing rate of labels. These four importance can be regarded as four uncertainty measurements (UMs) for ( U , P , d ). Next, numerical experiments and statistical tests are carried out on 15 datasets of UCI to demonstrate four UMs’ advantages and disadvantages. Finally, as an application for UM in p-CDIS, two better UMs are used as semi-supervised attribute reduction and two corresponding algorithms are designed that can automatically adapt to different missing rates of labels. The experimental results show the feasibility and superiority of the designed algorithms.
更多查看译文
关键词
p-CDIS,Uncertainty measurement,Indiscernibility relation,Conditional information entropy,Conditional information amount,Semi-supervised attribute reduction.
AI 理解论文
溯源树
样例
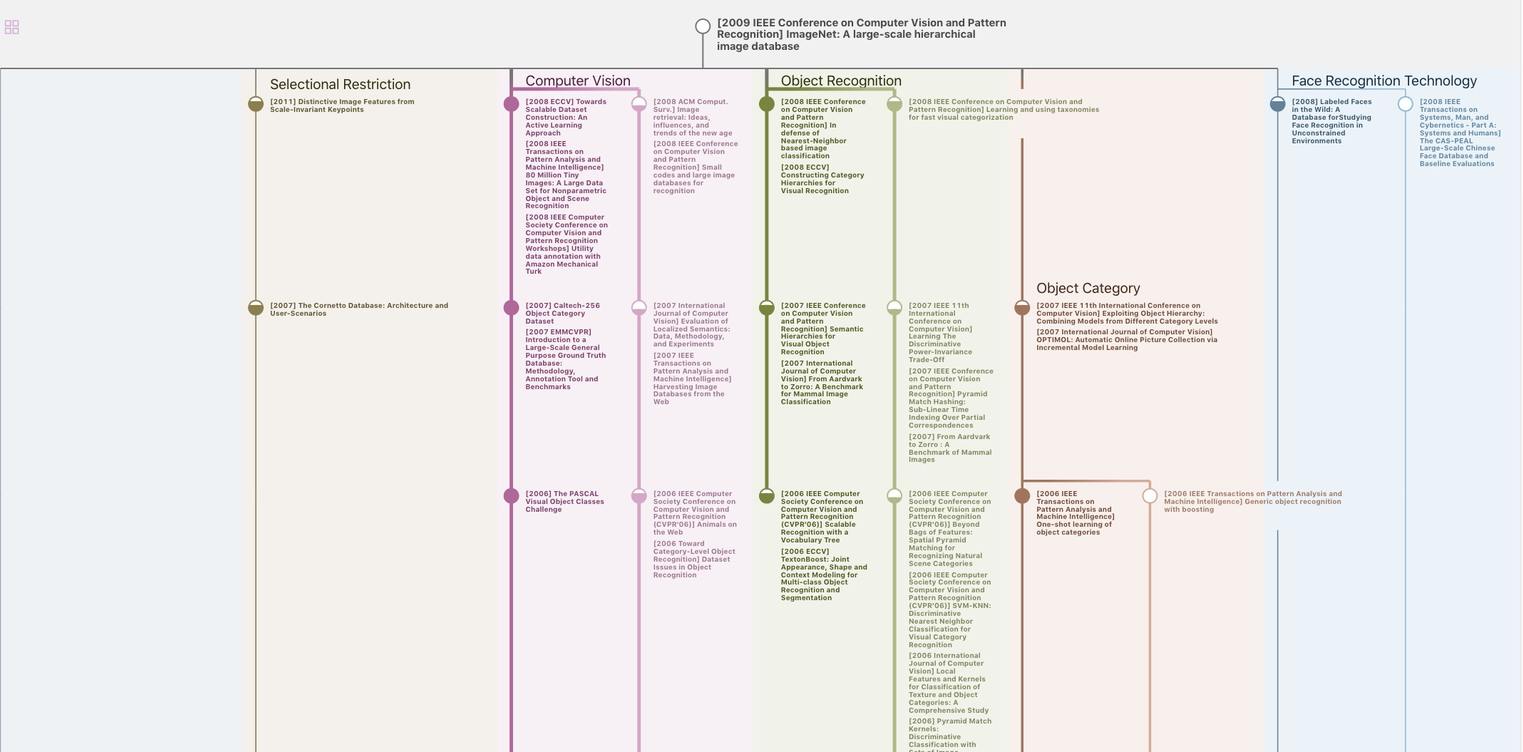
生成溯源树,研究论文发展脉络
Chat Paper
正在生成论文摘要