Multi-classification of High-Frequency Oscillations in Intracranial EEG Signals Based on CNN and Data Augmentation
Signal, image and video processing(2023)
Abstract
Interictal high-frequency oscillations (HFOs) recorded in intracranial electroencephalographic (iEEG) signals are reliable biomarkers for the epileptogenic zone. Visual identification of these particular events is manually time-consuming and is subject to clinicians’ expertise. Moreover, differentiating them from other transient events such as interictal epileptic spikes (IESs) presents a considerable challenge. Hence, various approaches have been developed with the aim of extracting automatically discriminant features for HFOs and IESs events. Typically, these approaches are based on machine learning (ML) algorithms, but their efficiency strongly depends on the computed features. To address this limitation, we explore deep learning (DL), as a powerful framework, for the classification of HFOs and IESs and propose a novel convolutional neural network (CNN) architecture for HFOs multi-classification. Time–frequency (TF)-based images, computed using the Stockwell transform of the events of interest, are used as inputs to the CNN-based approach. Furthermore, data augmentation (DA) is adopted to improve the generalization of the proposed CNN model. The numerical simulations on epileptic iEEG signals demonstrate that the proposed approach yields superior results when the DA is employed.
MoreTranslated text
Key words
Epilepsy,High-frequency oscillations,Time–frequency representation,Convolutional neural network,Data augmentation
AI Read Science
Must-Reading Tree
Example
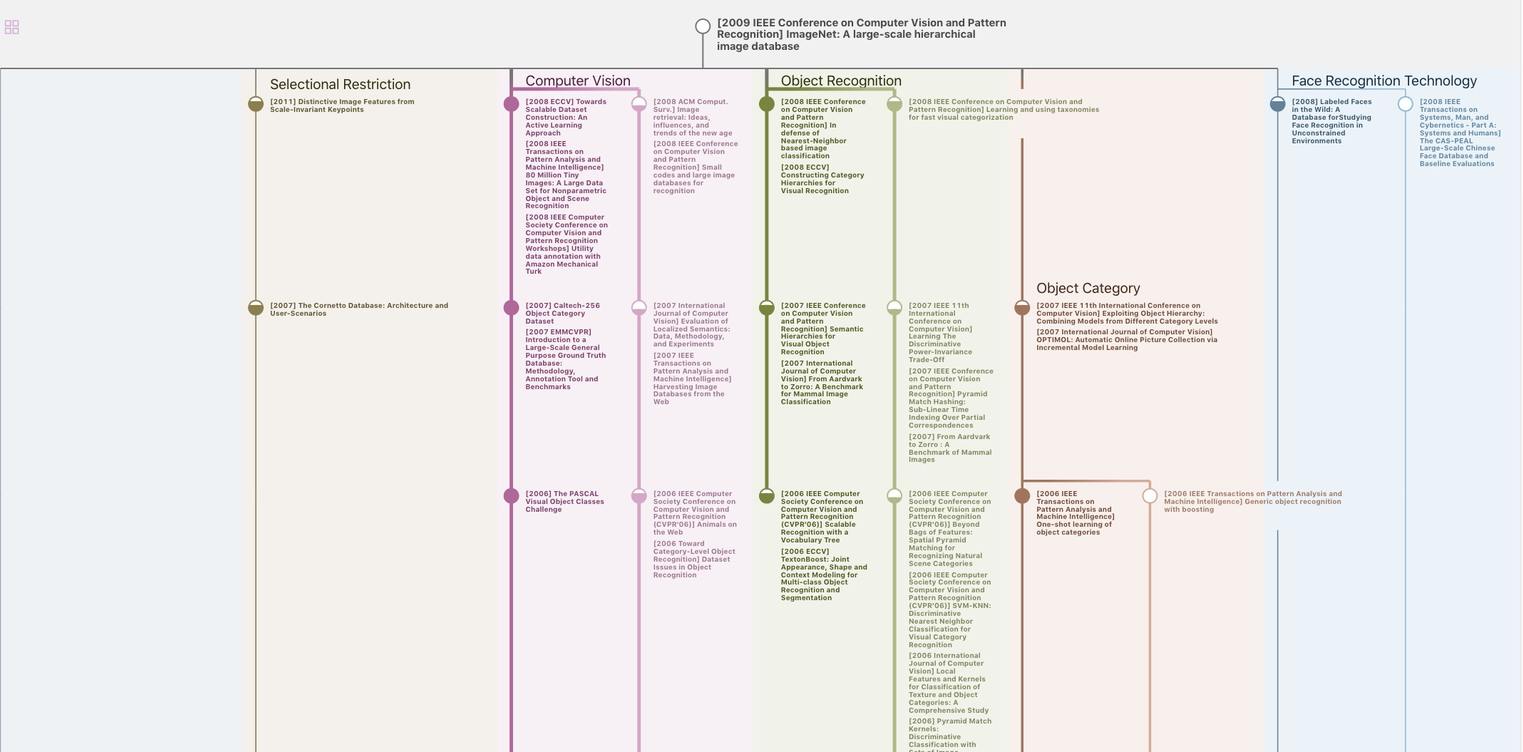
Generate MRT to find the research sequence of this paper
Chat Paper
Summary is being generated by the instructions you defined